Using Latent Class Probability Estimation and Residual Inclusion to Address Confounding in Medication Adherence Modeling
Value in health(2013)
摘要
It is well-established that patients’ medication adherence is often suboptimal and variable in chronic disease settings, but less is known about the association between adherence and outcomes, in part due to potential confounding by unobserved disease severity when using claims data. Our objective was to estimate adherence unexplained by observed characteristics and use this estimate to proxy severity, which could motivate better adherence but also worsen outcomes. A sample of the IMS LifeLink Health Plan Claims Database was used to identify a cohort of 26,893 primary prevention statin users. Adherence was estimated as the yearly proportion of days covered in beginning with the index statin prescription. A finite mixture model (FMM) was used to predict a posteriori the membership to better or worse adherer types. Using OLS regression adjusting for patient characteristics and the ‘healthy adherer effect’, the determinants of this probability were estimated. A residual inclusion estimation approach was used in a Cox proportional hazards model to estimate the effect of adherence on cardiovascular events. In a sample of new statin users, groups of low- and high-adherers existed. While year one adherence was not associated with cardiovascular events, year two mean adherence was (0.49 vs. 0.46, P<0.001). In the second year of statin use, the probability of being a high-adherer increased for males, those over 65 years and ‘healthy adherers’. The Cox model adjusting for the covariates and residuals showed that as year two adherence increased, CV hazard decreased by 38% (P>0.001), versus a 20% reduction when the residual was not included. The included residuals per se increased hazard by 28% (P=0.04), consistent with a disease severity effect. FMM estimation reveals adherence ‘types’. Estimating the probability of the ‘type’ allows isolation of a measure to address unobserved characteristics, such as disease severity, when modeling outcomes.
更多查看译文
AI 理解论文
溯源树
样例
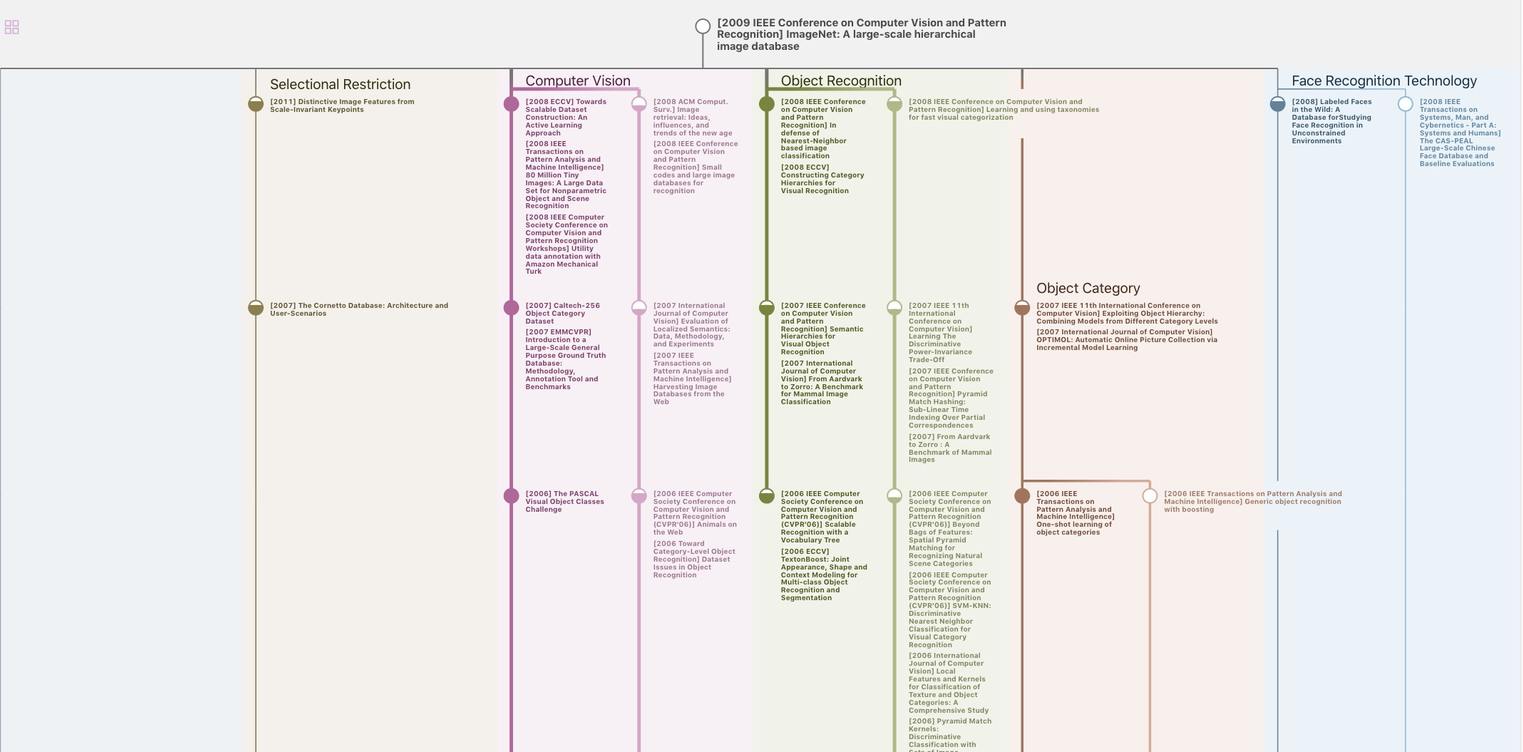
生成溯源树,研究论文发展脉络
Chat Paper
正在生成论文摘要