The Time Course of Individual Face Processing
Journal of vision(2011)
摘要
Whether the entry point of face recognition is at the individual level (e.g. Brad Pitt) or at the superordinate level (e.g. human) when processing familiar faces is unclear. We measured reaction times (RT) in a speeded go no-go task in two different conditions: at a superordinate level (unknown human faces among animals faces) and at an individual level (famous faces among unknown faces). In experiment 1 participants (n = 31) categorized stimuli by releasing a response button as quickly as possible when they saw a target (270 targets, 270 distractors for each condition). In experiment 2, subjects (n = 7) underwent 4 additional sessions of Experiment 1 with learning sessions of all targets in between. Experiment 1 demonstrates that individual faces require 200 ms longer to be categorized than human faces, whether computing minimum RT (359 ms vs 559 ms) or median RT (439 ms vs 635 ms). An unexpected result was that 3 subjects were much faster than other participants in the individual condition (median RT 393 ms vs 479 ms). Those fast subjects showed a 100 ms difference between superordinate and individual conditions. Experiment 2 revealed that subjects improved their RT but could not go below a 100 ms RT difference between the two conditions. Interestingly, the “fast” subjects identified in Experiment 1 could not improve their RT. Results show, in contrast to numerous studies, that additional time is required to categorize a face from a superordinate to an individual level. We identified two additional stages after the superordinate level, one short with an incompressible cost of ~100 ms, probably related to visual familiarity processes, the other longer with a cost of ~200 ms probably also including semantic activation. Therefore individual faces are processed hierarchically following the classic visual ventral stream hierarchy, at least when individual faces can't be preactivated (“bottom-up recognition”) like in those experiments.
更多查看译文
AI 理解论文
溯源树
样例
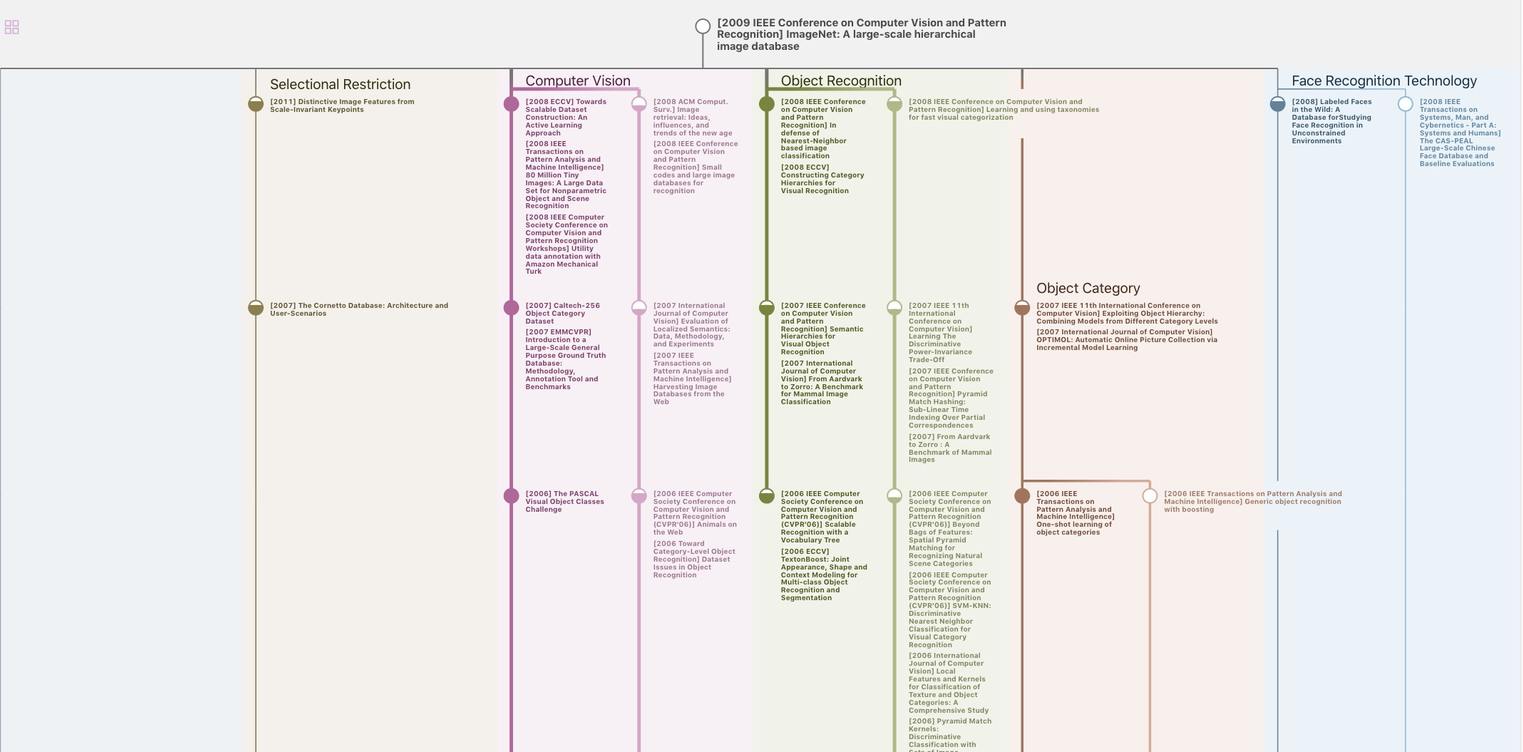
生成溯源树,研究论文发展脉络
Chat Paper
正在生成论文摘要