Electromagnetic Conductivity Imaging of Soil Salinity in an Estuarine–Alluvial Landscape
Soil Science Society of America Journal(2014)SCI 3区SCI 4区
Univ New S Wales | Monash Univ | IDL Univ Lisboa
Abstract
In coastal–estuarine agricultural landscapes, shallow and often saline water tables are problematic because they limit agricultural productivity. To understand the dynamics of rainfall events and tidal influences, natural resource management baseline data need to be collected to describe the heterogeneous nature of the soil and interactions with fluctuating groundwater tables, particularly in areas characterized by coastal acid sulfate soil. Electromagnetic induction instruments are increasingly being used because they measure the apparent soil electrical conductivity (ECa, mS m−1), which is related to soil salinity and hydrologic properties. Our aim was to demonstrate how the ECa data from a DUALEM‐421 can be used in conjunction with inversion software (EM4Soil) to develop electromagnetic conductivity images, which are two‐dimensional cross‐sections of estimates of the true electrical conductivity (σ, mS m−1). We show how the estimates of σ can be related to the electrical conductivity of a saturated soil paste extract (ECe, dS m−1) across an estuarine landscape and situated within Quaternary fluvial sediments adjacent to Rocky Mouth Creek on the far northern coast of New South Wales, Australia. Our results indicate that a good linear relationship (r2 = 0.70) can be established between σ and ECe, and the results reflect the existing soil landscape units.
MoreTranslated text
PDF
View via Publisher
AI Read Science
AI Summary
AI Summary is the key point extracted automatically understanding the full text of the paper, including the background, methods, results, conclusions, icons and other key content, so that you can get the outline of the paper at a glance.
Example
Background
Key content
Introduction
Methods
Results
Related work
Fund
Key content
- Pretraining has recently greatly promoted the development of natural language processing (NLP)
- We show that M6 outperforms the baselines in multimodal downstream tasks, and the large M6 with 10 parameters can reach a better performance
- We propose a method called M6 that is able to process information of multiple modalities and perform both single-modal and cross-modal understanding and generation
- The model is scaled to large model with 10 billion parameters with sophisticated deployment, and the 10 -parameter M6-large is the largest pretrained model in Chinese
- Experimental results show that our proposed M6 outperforms the baseline in a number of downstream tasks concerning both single modality and multiple modalities We will continue the pretraining of extremely large models by increasing data to explore the limit of its performance
Try using models to generate summary,it takes about 60s
Must-Reading Tree
Example
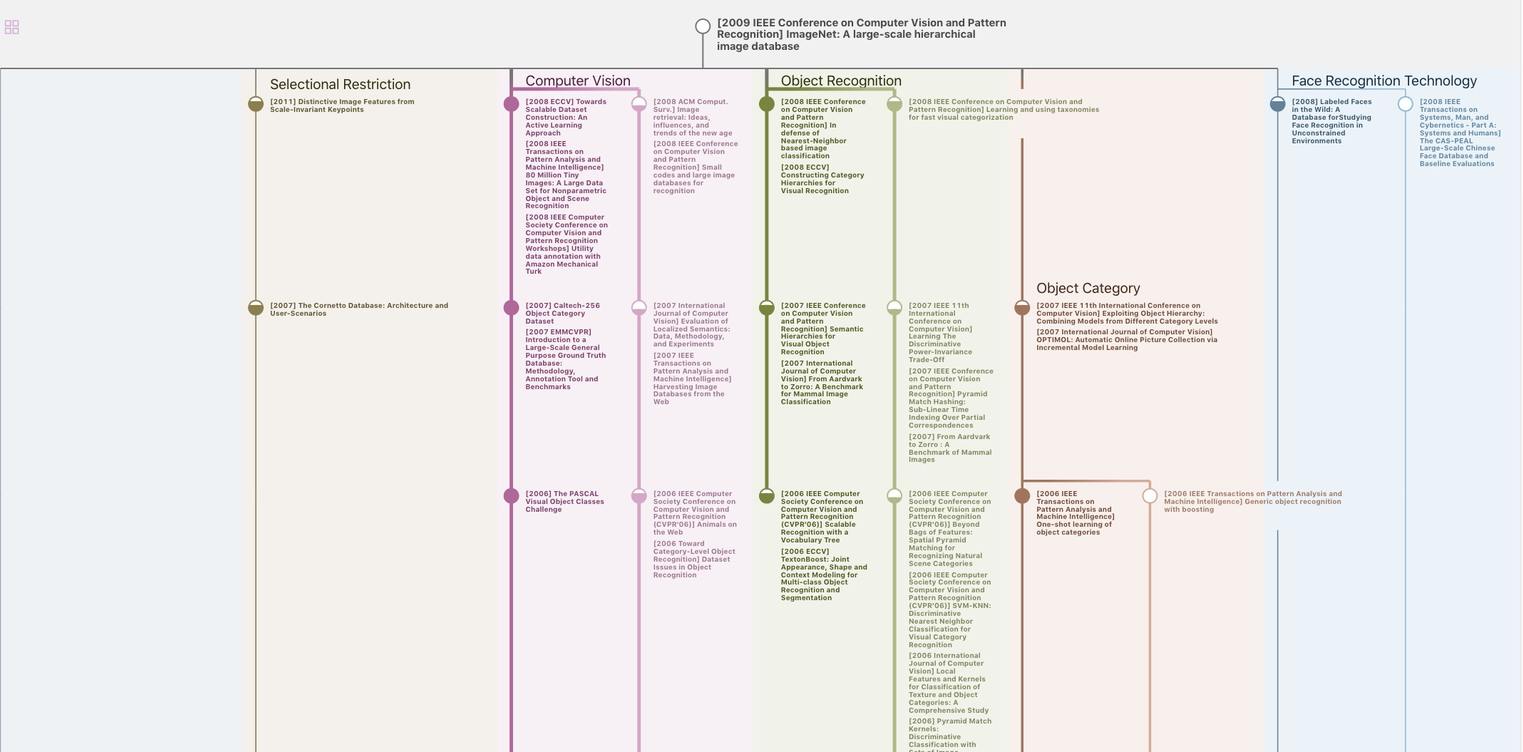
Generate MRT to find the research sequence of this paper
Related Papers
2016
被引用6 | 浏览
2018
被引用8 | 浏览
2015
被引用47 | 浏览
2017
被引用10 | 浏览
2018
被引用17 | 浏览
2018
被引用29 | 浏览
2018
被引用37 | 浏览
2016
被引用37 | 浏览
2017
被引用25 | 浏览
2019
被引用29 | 浏览
2020
被引用16 | 浏览
Data Disclaimer
The page data are from open Internet sources, cooperative publishers and automatic analysis results through AI technology. We do not make any commitments and guarantees for the validity, accuracy, correctness, reliability, completeness and timeliness of the page data. If you have any questions, please contact us by email: report@aminer.cn
Chat Paper
GPU is busy, summary generation fails
Rerequest