Convergence of Momentum-Based Stochastic Gradient Descent.
2020 IEEE 16TH INTERNATIONAL CONFERENCE ON CONTROL & AUTOMATION (ICCA)(2020)
Abstract
With the rapid increase of data amount in many fields, such as machine learning and networked systems, optimization-based methods inevitably confront the computational issues, which can be well dealt by the stochastic optimization strategies. As one of the most fundamental stochastic optimization algorithms, stochastic gradient descent (SGD) has been intensively developed and employed in the machine learning in the past decade. But unfortunately, due to the technical difficulties, other SGD based algorithms which could achieve better performance, such as momentum-based SGD (mSGD), still lack theoretical basis. Based on this fact, in this paper, we prove that the mSGD algorithm is almost surely convergent at each trajectory. The convergence rate of mSGD is also analyzed.
MoreTranslated text
Key words
networked systems,optimization-based methods,stochastic optimization strategies,stochastic optimization algorithms,stochastic gradient descent,machine learning,SGD based algorithms,momentum-based SGD,mSGD algorithm,momentum-based stochastic gradient
AI Read Science
Must-Reading Tree
Example
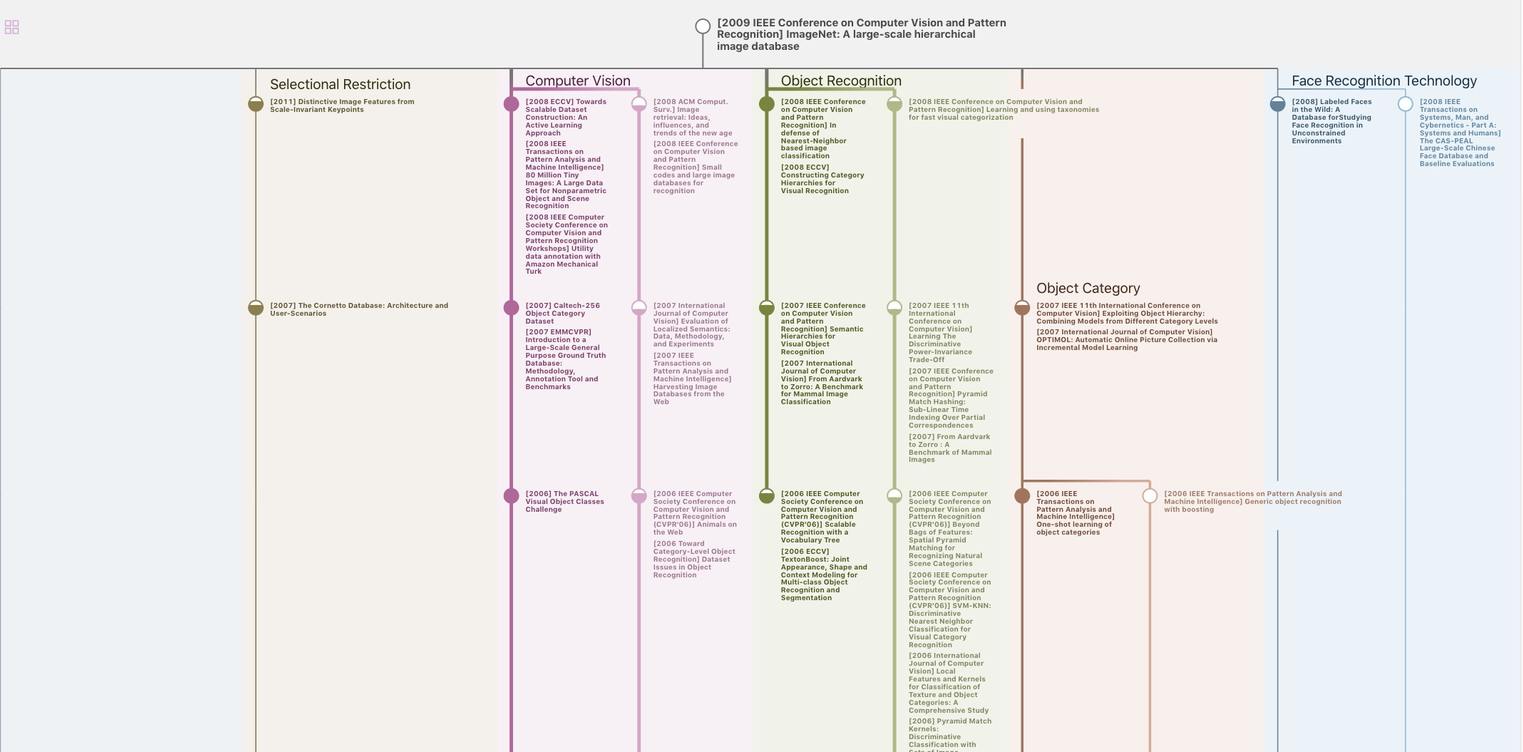
Generate MRT to find the research sequence of this paper
Chat Paper
Summary is being generated by the instructions you defined