Maximum-Likelihood Positioning Algorithm for Segmented Modular Detectors
The Journal of nuclear medicine(2009)
摘要
1517 Objectives To evaluate the maximum-likelihood (ML) positioning algorithm for use in segmented NaI(Tl) detector modules. Methods Segmented detector modules are formed from 1D or 2D array of discrete elements. The dimensions of each element are chosen to achieve desired intrinsic spatial resolution (ISR) for imaging applications. Measurement of positions in such modules is then reduced to the identification of the location of each element. Compared to conventional Anger logic, ML positioning algorithms have been shown to offer improved ISR and linearity. We used the ML method to characterize the performance of the SPRINT II detector module (1D array of 3mm-wide bars) coupled to a hexagonal array of seven 2 round PMTs. The mean detector response functions (MDRFs) were estimated from a 2D scan of a 1mm Tc-99m point source. Across the bars (x direction), the MDRF is a step function. The digitized positions are calculated directly by maximizing the likelihood function based on the MDRF with respect to the position of each bar. The resulting point spread function for the bars have unusual shapes. Thus, the ISR is not most effectively characterized by the FWHM value. We therefore used two metrics to quantify the ISR in the x direction: 1) percentage of correct bar identification; 2) spectral resolution of the modulation transfer function. These two metrics are related to the FWHM of a Gaussian distribution. Results The average CBI is above 72% in x direction, which is about a 3.3 mm FWHM equivalent. The average ISR is about 3.5mm in the y direction. These results are comparable to the original SPRINT II results even with the larger 2 PMTs. Conclusions The segmented detector, together with the ML algorithm, offers high ISR and extended field of view.
更多查看译文
AI 理解论文
溯源树
样例
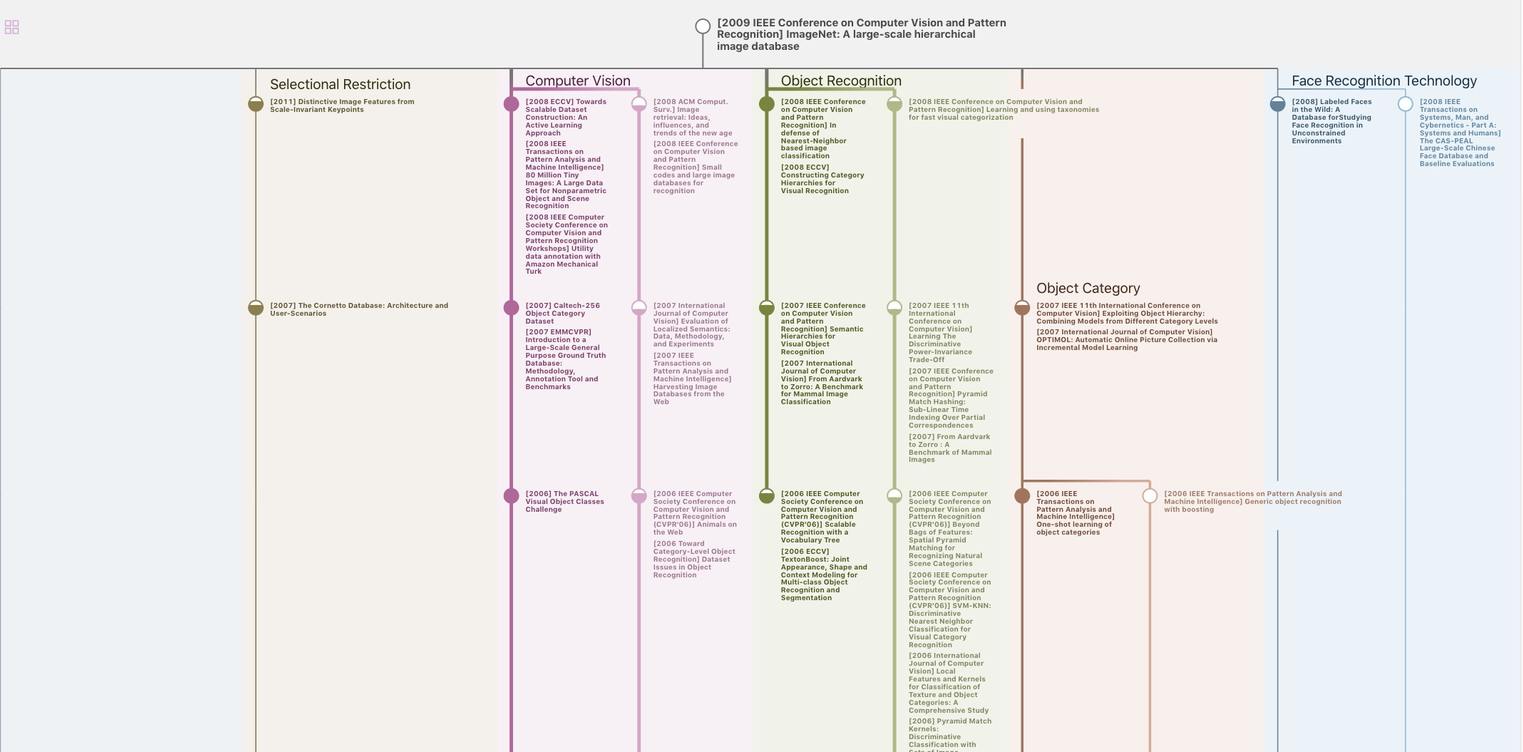
生成溯源树,研究论文发展脉络
Chat Paper
正在生成论文摘要