NeuralNetTools: Visualization and Analysis Tools for Neural Networks
Journal of Statistical Software(2018)
Abstract
Supervised neural networks have been applied as a machine learning technique to identify and predict emergent patterns among multiple variables. A common criticism of these methods is the inability to characterize relationships among variables from a fitted model. Although several techniques have been proposed to "illuminate the black box", they have not been made available in an open-source programming environment. This article describes the NeuralNetTools package that can be used for the interpretation of supervised neural network models created in R. Functions in the package can be used to visualize a model using a neural network interpretation diagram, evaluate variable importance by disaggregating the model weights, and perform a sensitivity analysis of the response variables to changes in the input variables. Methods are provided for objects from many of the common neural network packages in R, including caret, neuralnet, nnet, and RSNNS. The article provides a brief overview of the theoretical foundation of neural networks, a description of the package structure and functions, and an applied example to provide a context for model development with NeuralNetTools. Overall, the package provides a toolset for neural networks that complements existing quantitative techniques for data-intensive exploration.
MoreTranslated text
Key words
neural networks,plotnet,sensitivity,variable importance
PDF
View via Publisher
AI Read Science
AI Summary
AI Summary is the key point extracted automatically understanding the full text of the paper, including the background, methods, results, conclusions, icons and other key content, so that you can get the outline of the paper at a glance.
Example
Background
Key content
Introduction
Methods
Results
Related work
Fund
Key content
- Pretraining has recently greatly promoted the development of natural language processing (NLP)
- We show that M6 outperforms the baselines in multimodal downstream tasks, and the large M6 with 10 parameters can reach a better performance
- We propose a method called M6 that is able to process information of multiple modalities and perform both single-modal and cross-modal understanding and generation
- The model is scaled to large model with 10 billion parameters with sophisticated deployment, and the 10 -parameter M6-large is the largest pretrained model in Chinese
- Experimental results show that our proposed M6 outperforms the baseline in a number of downstream tasks concerning both single modality and multiple modalities We will continue the pretraining of extremely large models by increasing data to explore the limit of its performance
Try using models to generate summary,it takes about 60s
Must-Reading Tree
Example
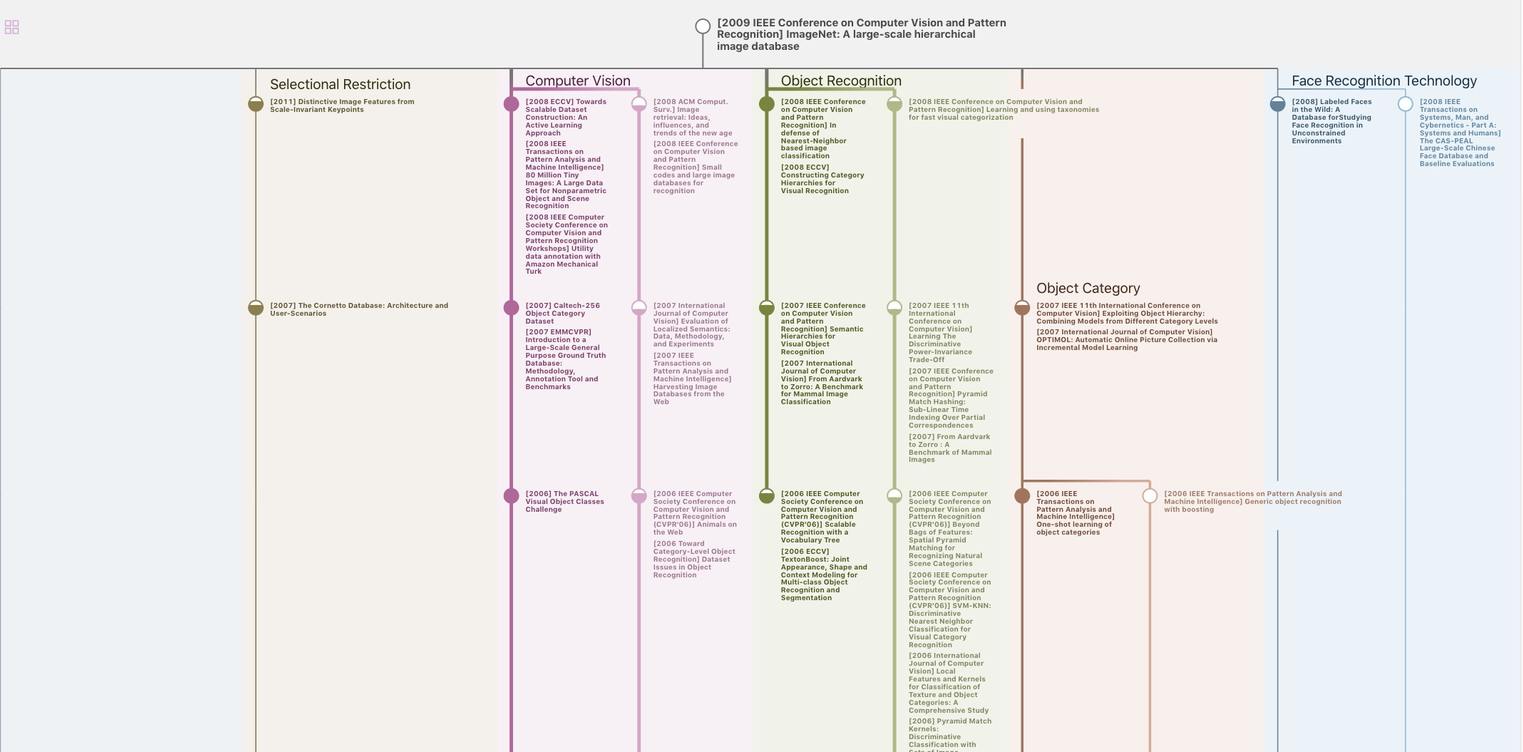
Generate MRT to find the research sequence of this paper
Related Papers
Journal of Transport Geography 2019
被引用14
Understanding Support Vector Machines with Polynomial Kernels
European Signal Processing Conference 2019
被引用9
Data-driven Ultimate Conditions Prediction and Stress-Strain Model for FRP-confined Concrete
Composite structures 2020
被引用63
Cancers 2020
被引用34
Journal of Dairy Science 2020
被引用32
Stock Price Reaction to the Drug Development Setbacks in the Pharmaceutical Industry
DARU Journal of Pharmaceutical Sciences 2021
被引用3
Automated PD-L1 Scoring Using Artificial Intelligence in Head and Neck Squamous Cell Carcinoma.
Cancers 2021
被引用20
PLoS ONE 2021
被引用1
SSRN Electronic Journal 2019
被引用1
BMC Neurology 2022
被引用3
An Expert System for Insect Pest Population Dynamics Prediction
Computers and Electronics in Agriculture 2022
被引用11
Papers in Applied Geography 2022
被引用0
Frontiers in Medicine 2022
被引用0
Frontiers in Aging Neuroscience 2022
被引用11
Remote sensing 2022
被引用8
Evidence-based Complementary and Alternative Medicine 2022
被引用2
Protective Effect of Zinc Oxide Nanoparticles on Spinal Cord Injury
FRONTIERS IN PHARMACOLOGY 2022
被引用2
BMC Veterinary Research 2022
被引用3
FRONTIERS IN ENDOCRINOLOGY 2023
被引用5
Marine Pollution Bulletin 2023
被引用4
FRONTIERS IN MOLECULAR NEUROSCIENCE 2023
被引用3
FRONTIERS IN PSYCHIATRY 2023
被引用4
ANNALS OF REGIONAL SCIENCE 2024
被引用0
COMPUTERS IN BIOLOGY AND MEDICINE 2023
被引用10
HELIYON 2023
被引用1
Data Disclaimer
The page data are from open Internet sources, cooperative publishers and automatic analysis results through AI technology. We do not make any commitments and guarantees for the validity, accuracy, correctness, reliability, completeness and timeliness of the page data. If you have any questions, please contact us by email: report@aminer.cn
Chat Paper
GPU is busy, summary generation fails
Rerequest