Deep Neural Network and Random Forest Hybrid Architecture for Learning to Detect Retinal Vessels in Fundus Images
Annual International Conference of the IEEE Engineering in Medicine and Biology Society IEEE Engineering in Medicine and Biology Society Annual International Conference(2015)
摘要
Vision impairment due to pathological damage of the retina can largely be prevented through periodic screening using fundus color imaging. However the challenge with large-scale screening is the inability to exhaustively detect fine blood vessels crucial to disease diagnosis. In this work we present a computational imaging framework using deep and ensemble learning based hybrid architecture for reliable detection of blood vessels in fundus color images. A deep neural network (DNN) is used for unsupervised learning of vesselness dictionaries using sparse trained denoising auto-encoders (DAE), followed by supervised learning of the DNN response using a random forest for detecting vessels in color fundus images. In experimental evaluation with the DRIVE database, we achieve the objective of vessel detection with max. avg. accuracy of 0.9327 and area under ROC curve of 0.9195.
更多查看译文
关键词
Computational imaging,deep learning,denoising auto-encoder,random forests,vessel detection
AI 理解论文
溯源树
样例
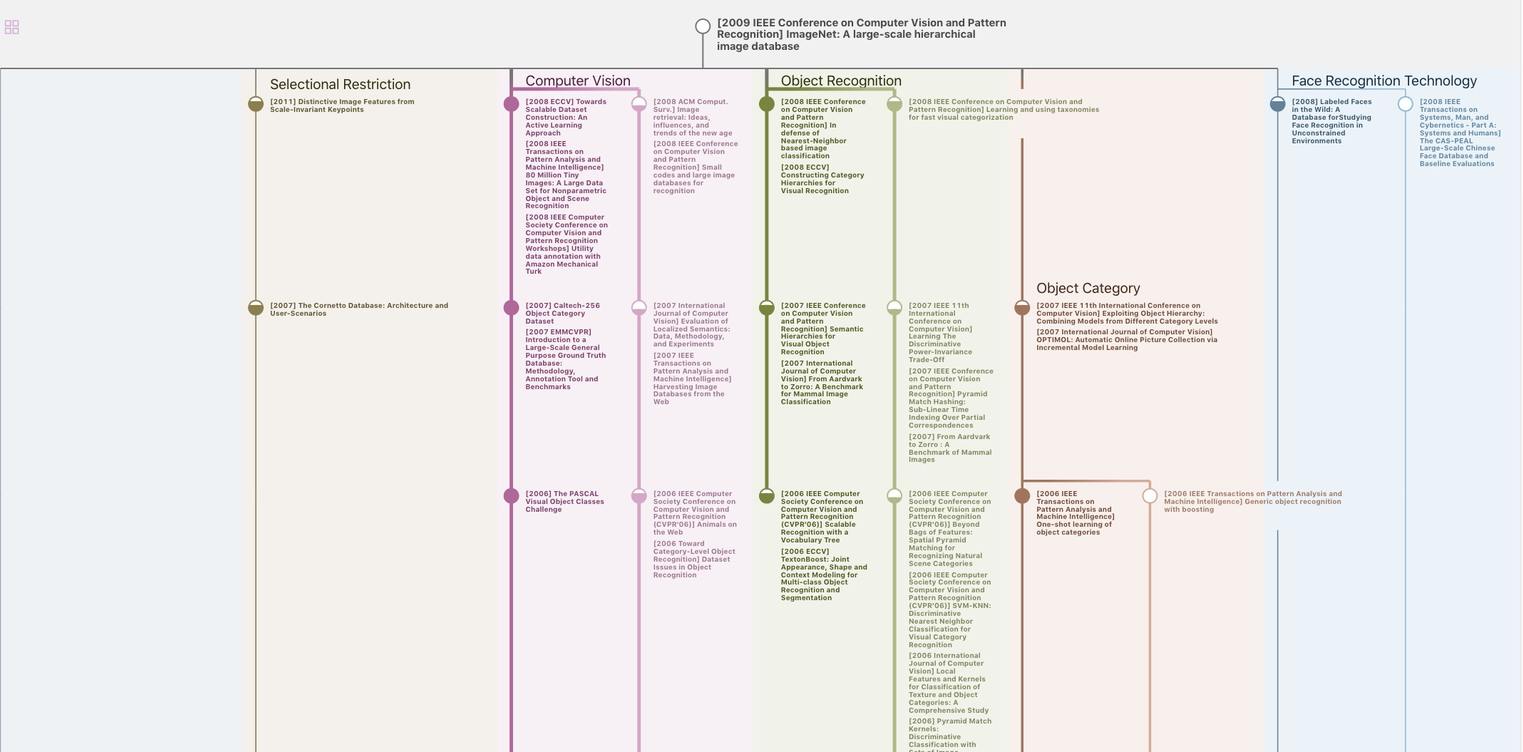
生成溯源树,研究论文发展脉络
Chat Paper
正在生成论文摘要