Parametric Non-Intrusive Reduced-Order Models Via Operator Inference for Large-Scale Rotating Detonation Engine Simulations
AIAA SCITECH 2023 Forum(2023)
摘要
This paper investigates the construction of unsteady parametric non-intrusive reduced-order models (ROMs) for large-scale, realistic rotating detonation rocket engine (RDRE) combustion chambers, with the end goal of employing these reduced models to accelerate the design optimization of RDREs. The large computational cost of the corresponding high-fidelity simulations restricts the number of parametric instances that can realistically be simulated to generate training data. Moreover, the resulting training data sets are often sparse and comprise down-sampled time instants from the high-fidelity simulation. This paper's goal is therefore to assess the potential of constructing accurate and predictive data-driven parametric ROMs for these challenging unsteady problems. To this end, we employ the operator inference (OpInf) procedure, which learns structure-preserving ROMs for systems with polynomial structure, without needing access to the high-fidelity simulator source code. To mitigate the challenge associated with training parametric ROMs using data from only a low number of parameter instances, we approximate the parametric dependence of the ROM operators via a Taylor expansion. We consider RDRE simulations for two injector designs, for which the corresponding high-fidelity simulations require more than $10,000$ cores and $5$--$10$ million CPU-hours to simulate $1$--$2$ ms of physical time. We consider parametric variations in the mass flow-rate and equivalence ratio. Our results are promising and show that OpInf can be used to construct accurate parametric ROMs from sparse training sets for parametric predictions outside of the training set and for predictions beyond the temporal training horizon.
更多查看译文
AI 理解论文
溯源树
样例
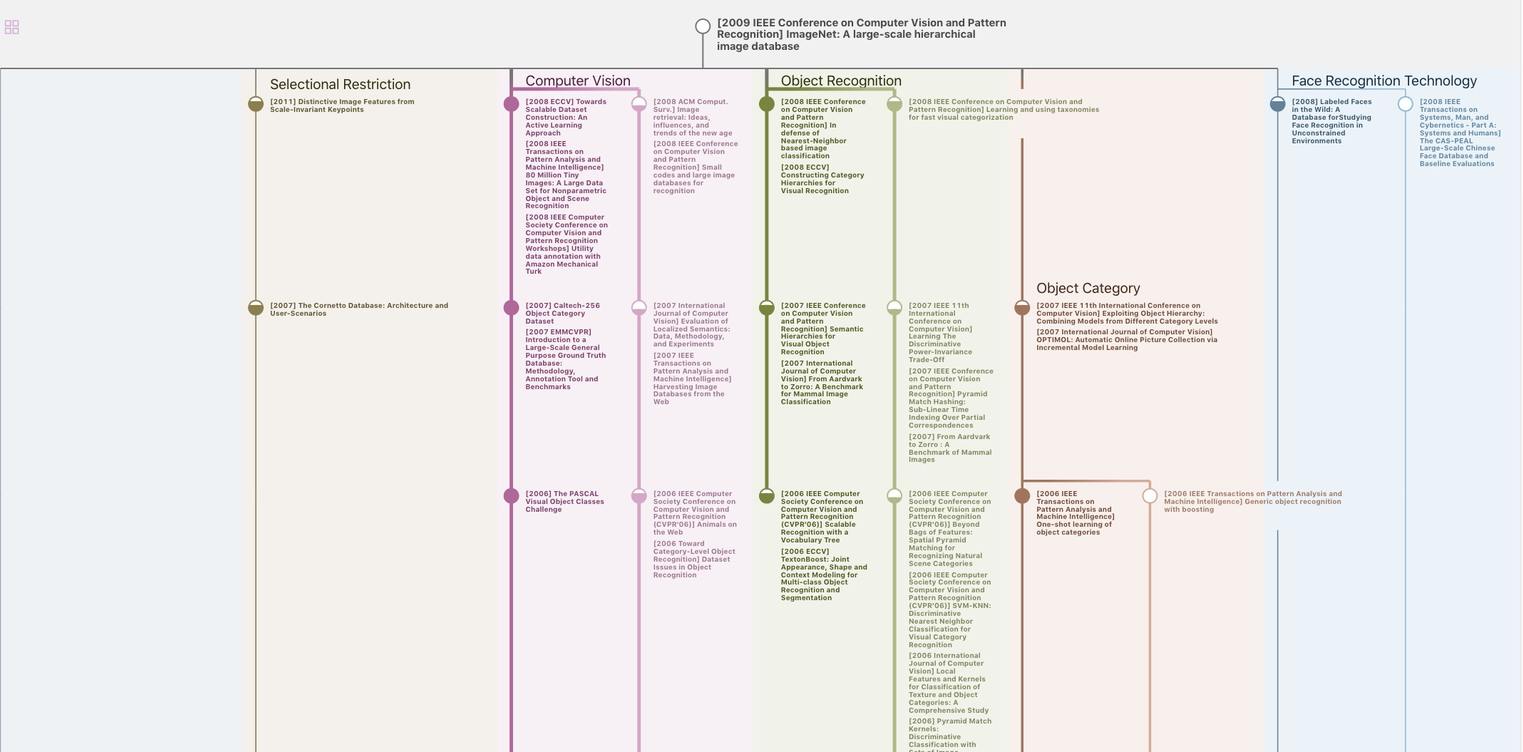
生成溯源树,研究论文发展脉络
Chat Paper
正在生成论文摘要