Spatial-temporal Graph Feature Learning Driven by Time–frequency Similarity Assessment for Robust Fault Diagnosis of Rotating Machinery
Advanced Engineering Informatics(2024)
摘要
Noises widely exist in the actual working environment of rotating machinery, which makes it difficult to extract high-quality fault features. Recently, the superiority of graph neural network (GNN) in the aspect of fault feature representation has been confirmed. By converting one-dimensional signals into graph structural data, hidden structural information inside the signals can be revealed. In this study, a novel graph-level fault diagnosis method based on spatial–temporal graph feature learning is developed. It mainly contains the following steps: First, Short-Time Fourier Transform (STFT) is used to construct time–frequency energy heatmaps, which can reflect the distribution of frequencies over time windows and the temporal variation characteristics. Second, a time–frequency similarity assessment method based on dynamic frequency warping is presented. On this basis, a time–frequency similarity graph (TFSG) is constructed to describe the frequency relationship over different time windows, which can bring extra structural information for the domain of time–frequency to support the subsequent classification task. Then, with the input of TFSG, a graph-level fault diagnosis model based on an improved GraphSAGE network (IGN) is developed. Finally, the fault labels for all TFSGs are recognized with a SoftMax classifier. Two experimental cases based on axial flow pumps and a public gearbox dataset verified the effectiveness of the proposed fault diagnosis method.
更多查看译文
关键词
Rotating machinery,Fault diagnosis,GraphSAGE,Time-frequency similarity graph
AI 理解论文
溯源树
样例
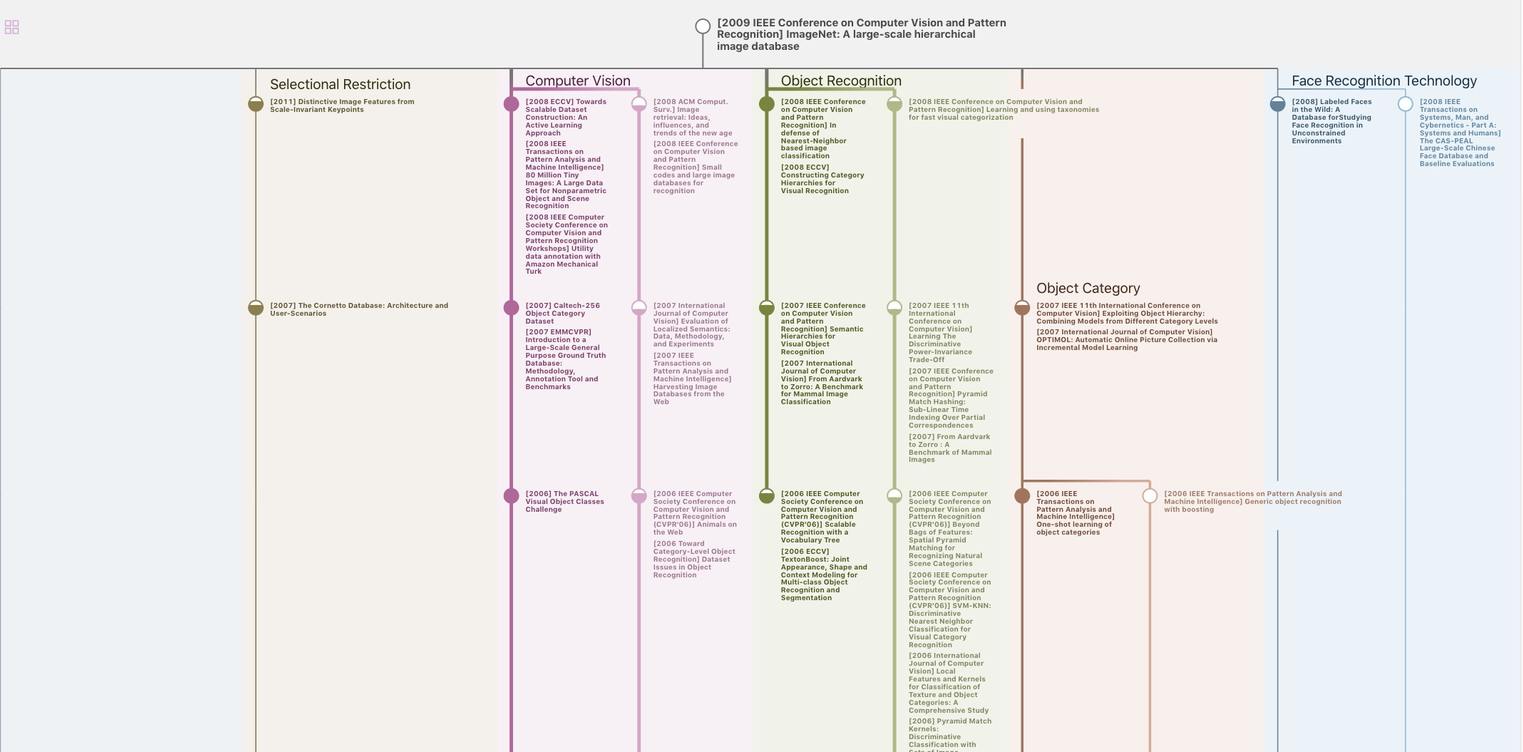
生成溯源树,研究论文发展脉络
Chat Paper
正在生成论文摘要