Group Membership Verification via Nonlinear Sparsifying Transform Learning
IEEE Access(2024)
Abstract
In today’s digitally interconnected landscape, confirming the genuine associations between entities—whether they are items, devices, or individuals—and specific groups is critical. This paper introduces a new group membership verification method while ensuring minimal information loss, coupled with privacy-preservation and discrimination priors. Instead of verifying based on a similarity score in the original data space, we use a min-max functional measure in a transformed space. This method comprises two stages: (i) generating candidate nonlinear transform representations, and (ii) evaluating the min-max measure over these representations for both group assignment and transform selection. We simultaneously determine group membership and pick the appropriate representation from the candidate set based on the evaluation score. To solve within this framework, we employ an iterative alternating algorithm that both learns the parameters of candidate transforms and assigns group membership. Our method’s efficacy is assessed on public datasets across various verification and identification scenarios and further tested on real-world image databases, CFP and LFW.
MoreTranslated text
Key words
Group testing,transform learning,discrimination,identification,verification
AI Read Science
Must-Reading Tree
Example
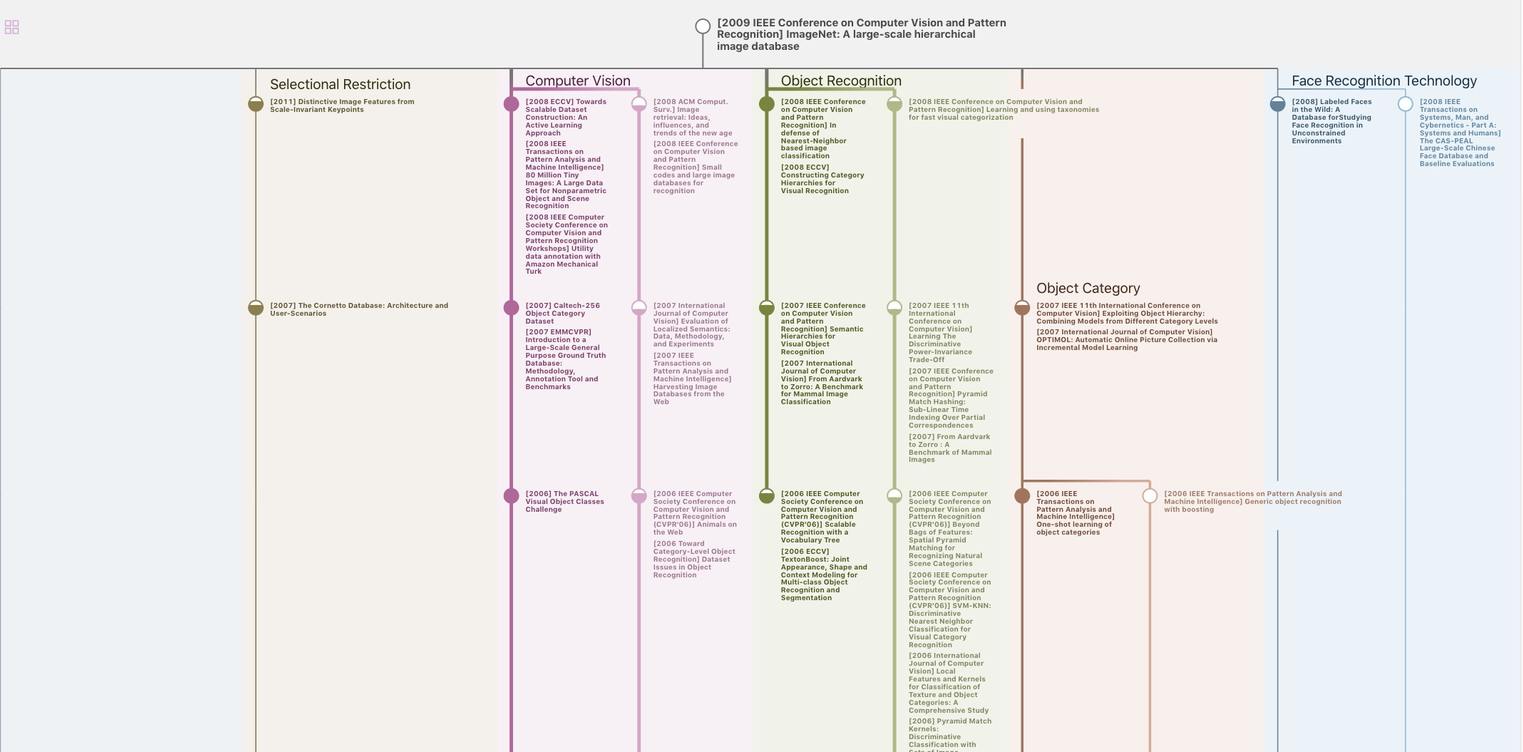
Generate MRT to find the research sequence of this paper
Chat Paper
Summary is being generated by the instructions you defined