Negative Constraints Underlie the ErbB Specificity of Epidermal Growth Factor-like Ligands
Journal of Biological Chemistry(2006)SCI 2区
Radboud Univ Nijmegen | Department of Cell Biology | Centre for Molecular and Biomolecular Informatics
Abstract
Epidermal growth factor (EGF)-like growth factors bind their ErbB receptors in a highly selective manner, but the molecular basis for this specificity is poorly understood. We have previously shown that certain residues in human EGF (Ser(2)-Asp(3)) and TGF alpha (Glu(26)) are not essential for their binding to ErbB1 but prevent binding to ErbB3 and ErbB4. In the present study, we have used a phage display approach to affinity-optimize the C-terminal linear region of EGF-like growth factors for binding to each ErbB receptor and thereby shown that Arg(45) in EGF impairs binding to both ErbB3 and ErbB4. By omitting all these so-called negative constraints from EGF, we designed a ligand designated panerbin that binds ErbB1, ErbB3, and ErbB4 with similarly high affinity as their wild-type ligands. Homology models, based on the known crystal structure of TGF alpha-bound ErbB1, showed that panerbin is able to bind ErbB1, ErbB3, and ErbB4 in a highly similar manner with respect to position and number of interaction sites. Upon in silico introduction of the experimentally known negative constraints into panerbin, we found that Arg45 induced local charge repulsion and Glu26 induced steric hindrance in a receptor-specific manner, whereas Ser2-Asp3 impaired binding due to a disordered conformation. Furthermore, radiolabeled panerbin was used to quantify the level of all three receptors on human breast cancer cells in a single radioreceptor assay. It is concluded that the ErbB specificity of EGF-like growth factors primarily results from the presence of a limited number of residues that impair the unintended interaction with other ErbB receptors.
MoreTranslated text
Key words
EGFR
PDF
View via Publisher
AI Read Science
AI Summary
AI Summary is the key point extracted automatically understanding the full text of the paper, including the background, methods, results, conclusions, icons and other key content, so that you can get the outline of the paper at a glance.
Example
Background
Key content
Introduction
Methods
Results
Related work
Fund
Key content
- Pretraining has recently greatly promoted the development of natural language processing (NLP)
- We show that M6 outperforms the baselines in multimodal downstream tasks, and the large M6 with 10 parameters can reach a better performance
- We propose a method called M6 that is able to process information of multiple modalities and perform both single-modal and cross-modal understanding and generation
- The model is scaled to large model with 10 billion parameters with sophisticated deployment, and the 10 -parameter M6-large is the largest pretrained model in Chinese
- Experimental results show that our proposed M6 outperforms the baseline in a number of downstream tasks concerning both single modality and multiple modalities We will continue the pretraining of extremely large models by increasing data to explore the limit of its performance
Try using models to generate summary,it takes about 60s
Must-Reading Tree
Example
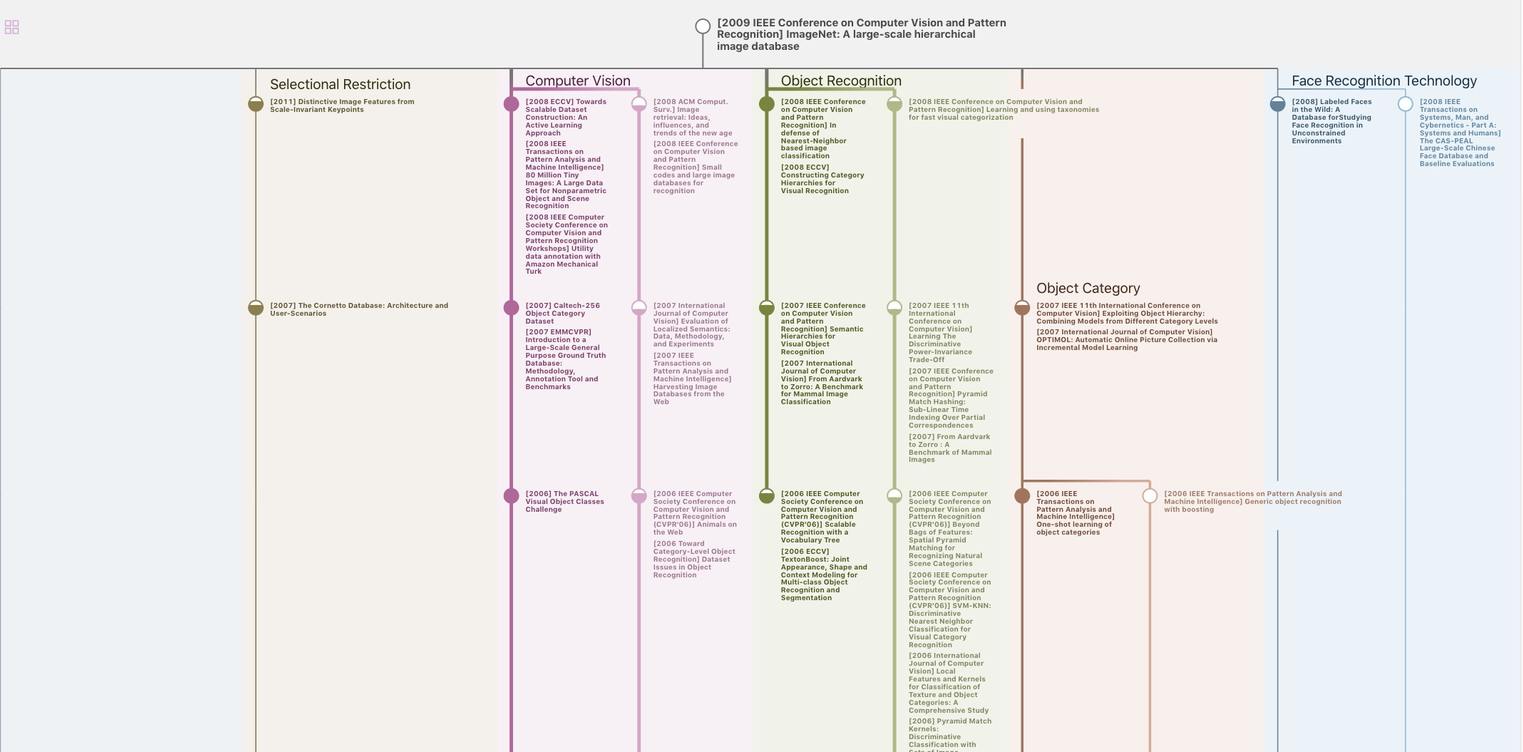
Generate MRT to find the research sequence of this paper
Related Papers
A Potent Anti-Carcinoma and Anti-Acute Myeloblastic Leukemia Agent, AG490
Anti-Cancer Agents in Medicinal Chemistry 2008
被引用33
Rational Optimization of a Bispecific Ligand Trap Targeting EGF Receptor Family Ligands
Molecular Medicine 2009
被引用13
Role of the C-terminal Linear Region of EGF-like Growth Factors in ErbB Specificity
Growth Factors 2009
被引用5
MABS 2014
被引用16
Egf-Containing Membrane-Bound Mucins: A Hidden Erbb2 Targeting Pathway?
JOURNAL OF MEDICINAL CHEMISTRY 2020
被引用11
Data Disclaimer
The page data are from open Internet sources, cooperative publishers and automatic analysis results through AI technology. We do not make any commitments and guarantees for the validity, accuracy, correctness, reliability, completeness and timeliness of the page data. If you have any questions, please contact us by email: report@aminer.cn
Chat Paper
GPU is busy, summary generation fails
Rerequest