Motility-Induced Phase Separation
Annual Review of Condensed Matter Physics(2015)SCI 1区
Univ Edinburgh | Univ Paris Diderot
Abstract
Self-propelled particles include both self-phoretic synthetic colloids and various microorganisms. By continually consuming energy, they bypass the laws of equilibrium thermodynamics. These laws enforce the Boltzmann distribution in thermal equilibrium: The steady state is then independent of kinetic parameters. In contrast, self-propelled particles tend to accumulate where they move more slowly. They may also slow down at high density for either biochemical or steric reasons. This creates positive feedback, which can lead to motility-induced phase separation (MIPS) between dense and dilute fluid phases. At leading order in gradients, a mapping relates variable-speed, self-propelled particles to passive particles with attractions. This deep link to equilibrium phase separation is confirmed by simulations but generally breaks down at higher order in gradients: New effects, with no equilibrium counterpart, then emerge. We give a selective overview of the fast-developing field of MIPS, focusing on theory and simulation but including a brief speculative survey of its experimental implications.
MoreTranslated text
Key words
self-propelled particles,bacteria,phase separation,motility,active Brownian,run-and-tumble
PDF
View via Publisher
AI Read Science
AI Summary
AI Summary is the key point extracted automatically understanding the full text of the paper, including the background, methods, results, conclusions, icons and other key content, so that you can get the outline of the paper at a glance.
Example
Background
Key content
Introduction
Methods
Results
Related work
Fund
Key content
- Pretraining has recently greatly promoted the development of natural language processing (NLP)
- We show that M6 outperforms the baselines in multimodal downstream tasks, and the large M6 with 10 parameters can reach a better performance
- We propose a method called M6 that is able to process information of multiple modalities and perform both single-modal and cross-modal understanding and generation
- The model is scaled to large model with 10 billion parameters with sophisticated deployment, and the 10 -parameter M6-large is the largest pretrained model in Chinese
- Experimental results show that our proposed M6 outperforms the baseline in a number of downstream tasks concerning both single modality and multiple modalities We will continue the pretraining of extremely large models by increasing data to explore the limit of its performance
Try using models to generate summary,it takes about 60s
Must-Reading Tree
Example
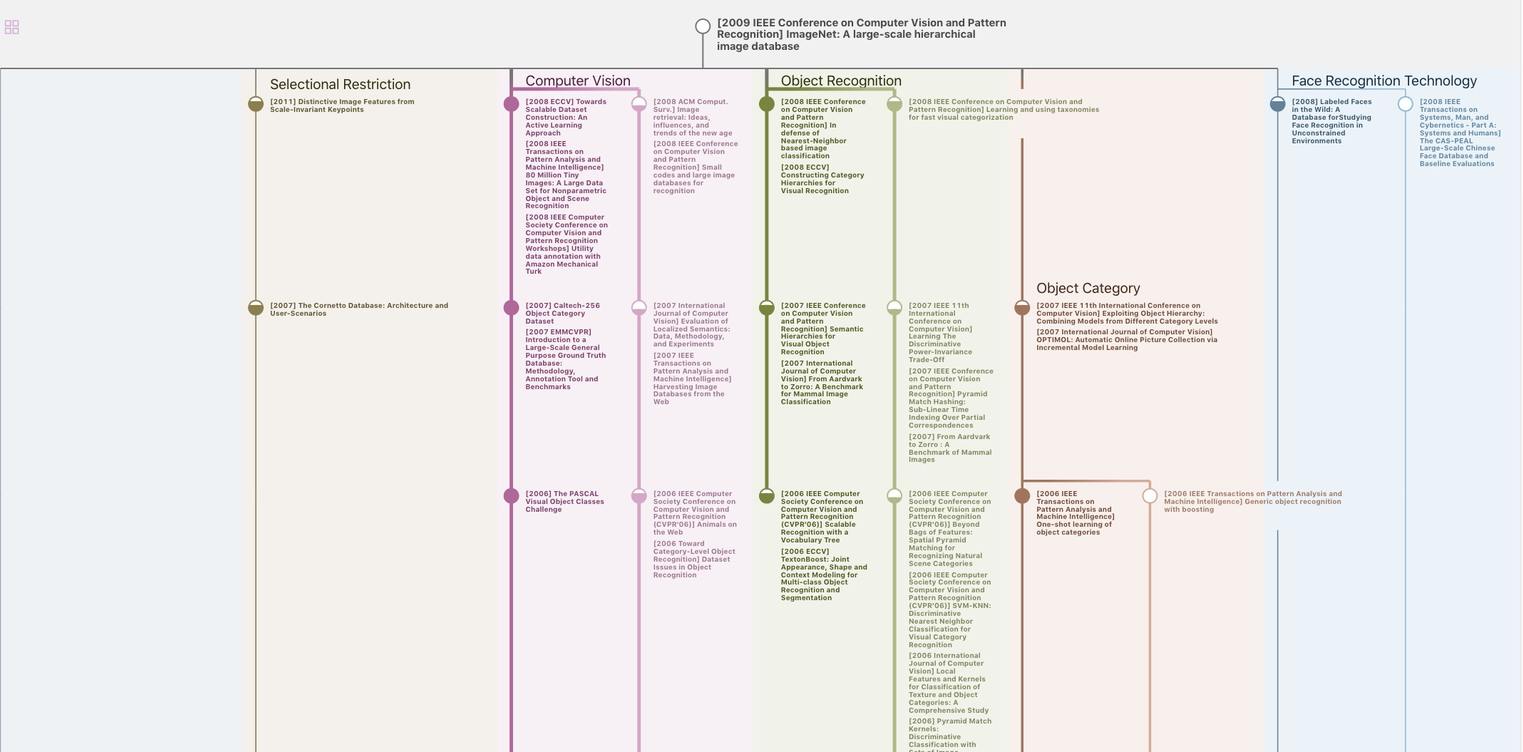
Generate MRT to find the research sequence of this paper
Related Papers
2002
被引用166 | 浏览
2002
被引用75 | 浏览
2007
被引用10 | 浏览
2009
被引用64 | 浏览
2006
被引用101 | 浏览
2012
被引用65 | 浏览
2018
被引用50 | 浏览
2019
被引用24 | 浏览
Data Disclaimer
The page data are from open Internet sources, cooperative publishers and automatic analysis results through AI technology. We do not make any commitments and guarantees for the validity, accuracy, correctness, reliability, completeness and timeliness of the page data. If you have any questions, please contact us by email: report@aminer.cn
Chat Paper
去 AI 文献库 对话