Psychological Flexibility Skills and Mental Wellbeing in Athletes: an Exploration of Associations and Gender Differences
Psychology and Behavioral Sciences(2024)
Department of Psychology | Karolinska Institute | Swedish School of Sport and Health Sciences | Faculty of Sport and Health Sciences | Finnish Institute of High Performance Sport KIHU
Abstract
Psychological flexibility skills (PFS) have shown to be an important aspect of wellbeing among the general population, however, there is a gap in research exploring flexibility skills among athletes. The present study examined the effects of PFS on young athletes’ (n = 106, M = 19.9) psychological wellbeing, symptomatology, and gender differences. Data was investigated using correlations and linear regression analyses. Higher PFS was associated with subjective wellbeing, recovery experiences, self-esteem, and lower levels of perceived stress, and depression symptoms (r = 0.30-0.53). Among the PFS, value-based actions acted as the strongest predictor for mental wellbeing and symptoms. In addition, avoidance of distressing thoughts and emotions related to sport was a significant predictor for self-esteem, symptoms of stress and depression but not for subjective wellbeing and recovery experience. Also, our results highlighted gender disparities, with female athletes reporting higher stress and depressive symptoms, while males exhibited better mental wellbeing, psychological recovery, self-esteem, and higher psychological flexibility skills. Overall, engagement in value-based actions may enhance athletes’ mental wellbeing. Further, it might be important to pay attention to individual differences related to gender both when assessing psychological flexibility skills and when applying interventions aimed at enhancing mental wellbeing among athletes.
MoreTranslated text
PDF
View via Publisher
AI Read Science
AI Summary
AI Summary is the key point extracted automatically understanding the full text of the paper, including the background, methods, results, conclusions, icons and other key content, so that you can get the outline of the paper at a glance.
Example
Background
Key content
Introduction
Methods
Results
Related work
Fund
Key content
- Pretraining has recently greatly promoted the development of natural language processing (NLP)
- We show that M6 outperforms the baselines in multimodal downstream tasks, and the large M6 with 10 parameters can reach a better performance
- We propose a method called M6 that is able to process information of multiple modalities and perform both single-modal and cross-modal understanding and generation
- The model is scaled to large model with 10 billion parameters with sophisticated deployment, and the 10 -parameter M6-large is the largest pretrained model in Chinese
- Experimental results show that our proposed M6 outperforms the baseline in a number of downstream tasks concerning both single modality and multiple modalities We will continue the pretraining of extremely large models by increasing data to explore the limit of its performance
Try using models to generate summary,it takes about 60s
Must-Reading Tree
Example
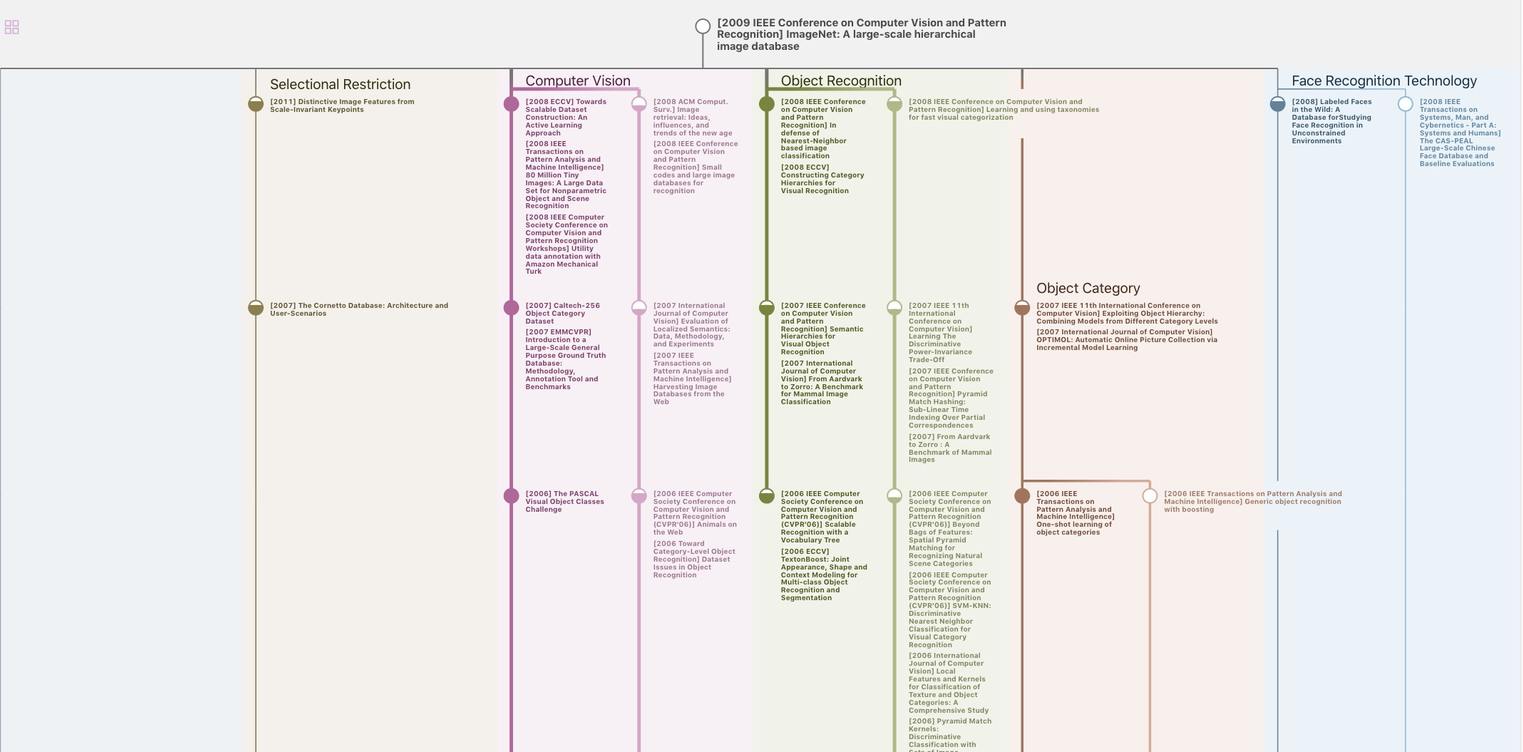
Generate MRT to find the research sequence of this paper
Related Papers
2011
被引用1651 | 浏览
2008
被引用192 | 浏览
2013
被引用128 | 浏览
2014
被引用33 | 浏览
2014
被引用74 | 浏览
2011
被引用219 | 浏览
2017
被引用177 | 浏览
Psychological Flexibility As A Malleable Public Health Target: Evidence from A Representative Sample
2017
被引用166 | 浏览
2018
被引用33 | 浏览
2019
被引用51 | 浏览
2019
被引用684 | 浏览
2020
被引用104 | 浏览
Data Disclaimer
The page data are from open Internet sources, cooperative publishers and automatic analysis results through AI technology. We do not make any commitments and guarantees for the validity, accuracy, correctness, reliability, completeness and timeliness of the page data. If you have any questions, please contact us by email: report@aminer.cn
Chat Paper
去 AI 文献库 对话