PARAMETRIC-PEARSON-BASED INDEPENDENT COMPONENT ANALYSIS FOR FREQUENCY-DOMAIN BLIND SPEECH SEPARATION
European Signal Processing Conference(2006)
摘要
Separation performance is improved in frequency-domain blind source separation (BSS) of speech with independent component analysis (ICA) by applying a parametric Pear- son distribution system. ICA adaptation rules include a score function determined by approximated source distribu- tion, and better approximation improves separation per- formance. Previously, conventional hyperbolic tangent (tanh) or generalized Gaussian distribution (GGD) was uniformly applied to the score function for all frequency bins, despite the fact that a wideband speech signal has different distributions at different frequencies. To obtain better score functions, we propose the integration of a parametric Pear- son distribution system with ICA learning rules. The score function is estimated by using appropriate Pearson distribu- tion parameters for each frequency bin. We consider three estimation methods with Pearson distribution parameters and conduct separation experiments with real speech sig- nals convolved with actual room impulse responses. Conse- quently, the signal-to-interference ratio (SIR) of the pro- posed methods significantly improve over 3 dB compared to conventional methods.
更多查看译文
关键词
Gaussian distribution,blind source separation,frequency-domain analysis,independent component analysis,speech processing,ICA learning rule,approximated source distribution,frequency-domain blind source separation,frequency-domain blind speech separation,generalized Gaussian distribution,hyperbolic tangent,independent component analysis,parametric Pearson distribution system,parametric-Pearson-based ICA,signal-to-interference ratio,speech signal,
AI 理解论文
溯源树
样例
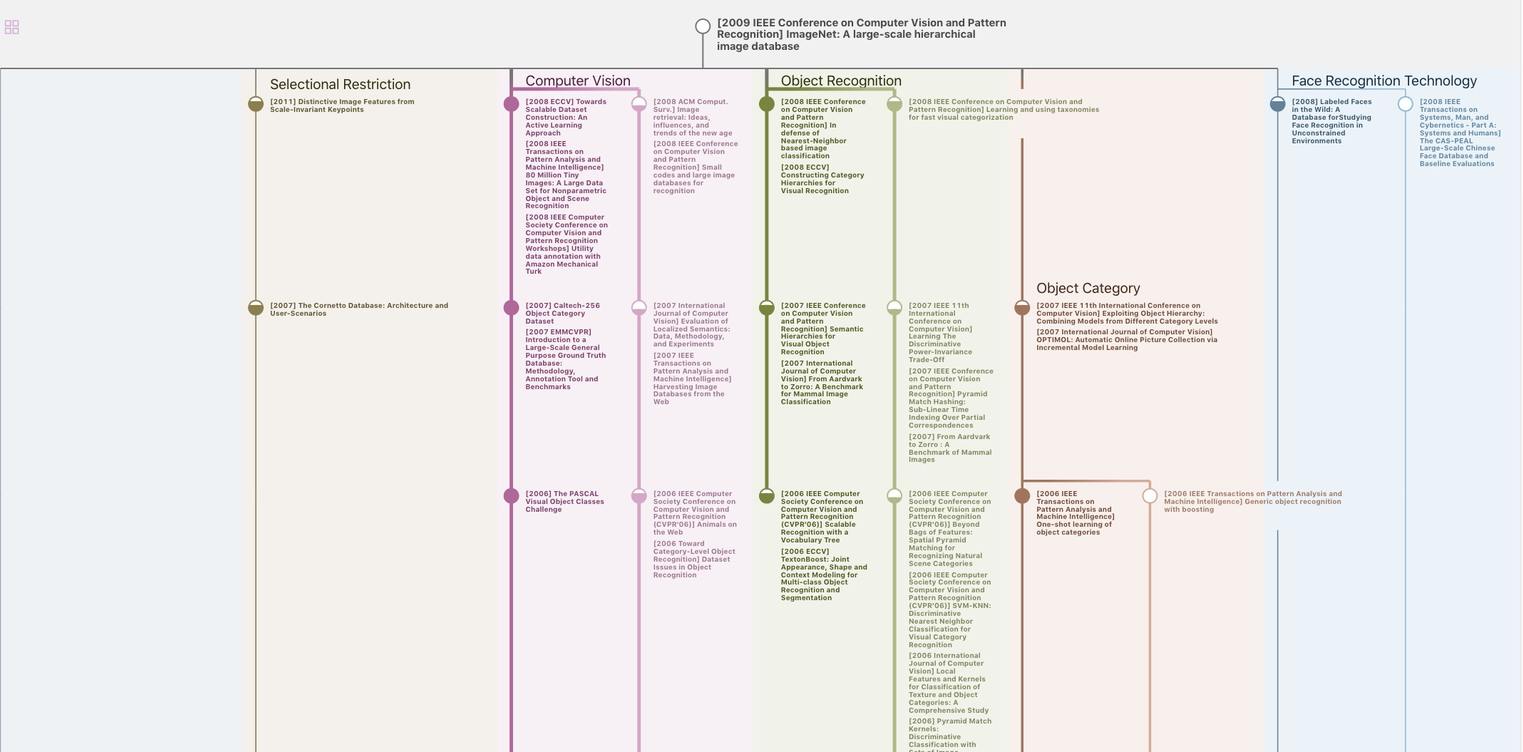
生成溯源树,研究论文发展脉络
Chat Paper
正在生成论文摘要