On the sensor choice and data analysis for classification of elementary upper limb movements
Biomedical and Health Informatics(2014)
摘要
In this paper we present a systematic exploration for determining the appropriate type of inertial sensor and the associated data processing techniques for classifying four fundamental movements of the upper limb. Our motivation was to explore classification techniques that are of low computational complexity enabling low power processing on body-worn sensor nodes for unhindered operation over a prolonged time. Kinematic data was collected from 18 healthy subjects, repeating 20 trials of each movement, using tri-axial accelerometers and tri-axial rate gyroscopes located near the wrist. Ten time-domain features extracted from data from individual sensor streams, their modulus and specific fused signals, were used to train classifiers based on three learning algorithms: LDA, QDA and SVM. Each classifier was evaluated using a leave-one-subject-out strategy. Our results show that we can correctly identify the four arm movements, with sensitivities in the range of 83-96%, using data from just a tri-axial gyroscope located near the wrist, and requiring only 12 features in combination with the lower complexity LDA learning algorithm.
更多查看译文
关键词
accelerometers,biomechanics,biomedical telemetry,body area networks,data analysis,gyroscopes,medical signal processing,signal classification,statistical analysis,support vector machines,wireless sensor networks,LDA learning algorithm,QDA learning algorithm,SVM learning algorithm,body worn sensor nodes,data analysis,data processing techniques,elementary upper limb movement classification,inertial sensor type,kinematic data,linear discriminant analysis,low power processing,quadratic discriminant analysis,support vector machine,time domain features,triaxial accelerometers,triaxial rate gyroscopes,upper limb fundamental movements
AI 理解论文
溯源树
样例
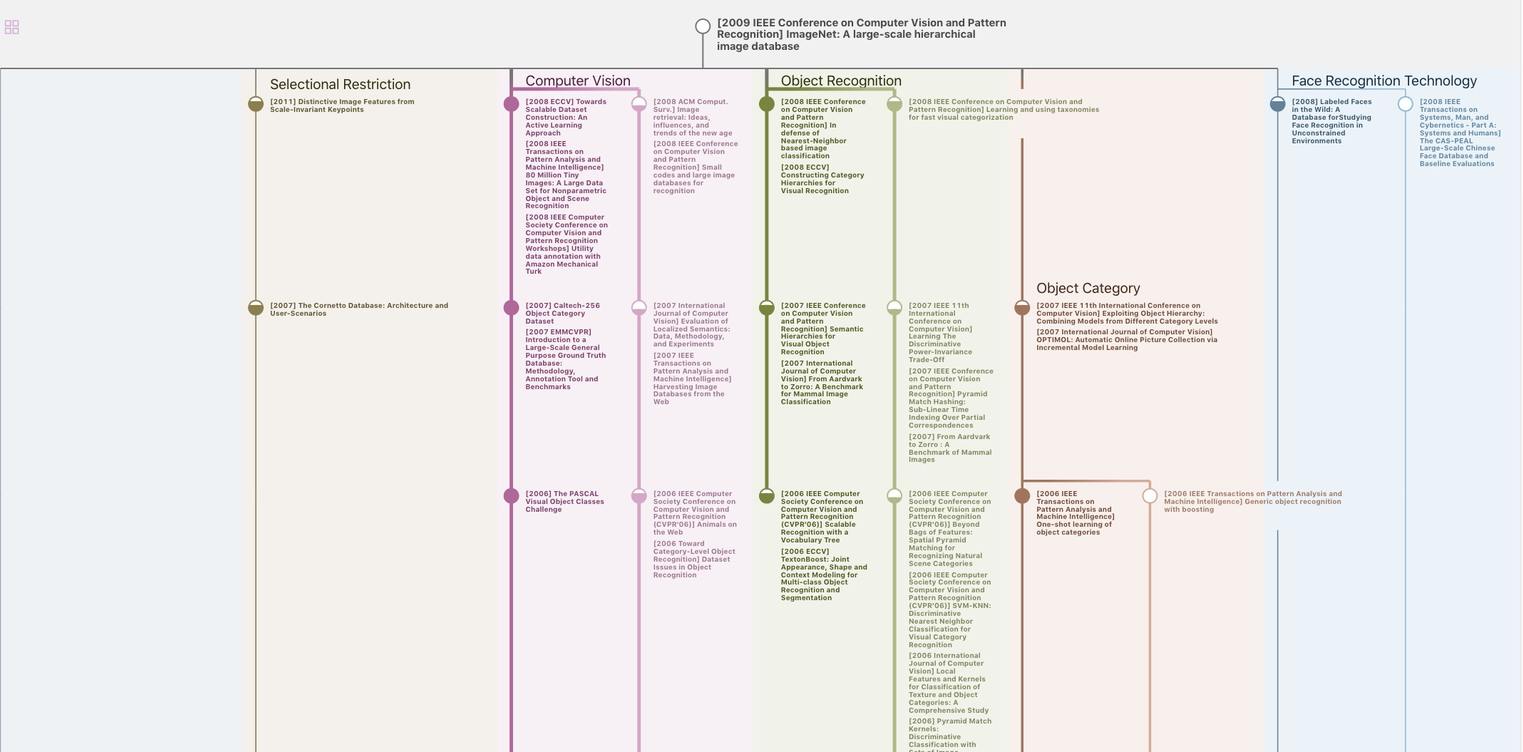
生成溯源树,研究论文发展脉络
Chat Paper
正在生成论文摘要