Robust feature vector for efficient human detection
Applied Imagery Pattern Recognition Workshop: Sensing for Control and Augmentation, 2013 IEEE(2013)
摘要
This research presents a method for the automatic detection of a dismounted human at long range from a single, highly compressed image. The histogram of oriented gradients (HOG) method provides the feature vector, a support vector machine performs the classification, and the JPEG2000 standard compresses the image. This work presents an understanding of how HOG for human detection holds up as range and compression increases. The results indicate that HOG remains effective even at long distances: the average miss rate and false alarm rate are both kept to 5% for humans only 12 pixels tall and 4-5 pixels wide in uncompressed images. Next, classification performance for humans at close range (100 pixels tall) is evaluated for compressed and uncompressed versions of the same test images. Using a compression ratio of 32:1 (97% of each image's data is discarded and the image is reconstructed from only the 3% retained), the miss rates for the compressed and uncompressed images are equivalent at 0.5% while the 1.0% false alarm rate for the compressed images is only slightly higher than the 0.5% rate for the uncompressed images. Finally, this work depicts good detection performance for humans at long ranges in highly compressed images. Insights into important design issues-for example, the impact of the amount and type of training data needed to achieve this performance-are also discussed.
更多查看译文
关键词
data compression,image classification,image coding,object detection,standards,support vector machines,HOG,JPEG2000 standard,average miss rate,classification,compression ratio,dismounted human automatic detection,false alarm rate,feature vector,highly compressed image,histogram of oriented gradients,image compression,support vector machine,automatic human detection,histogram of oriented gradients,image compression
AI 理解论文
溯源树
样例
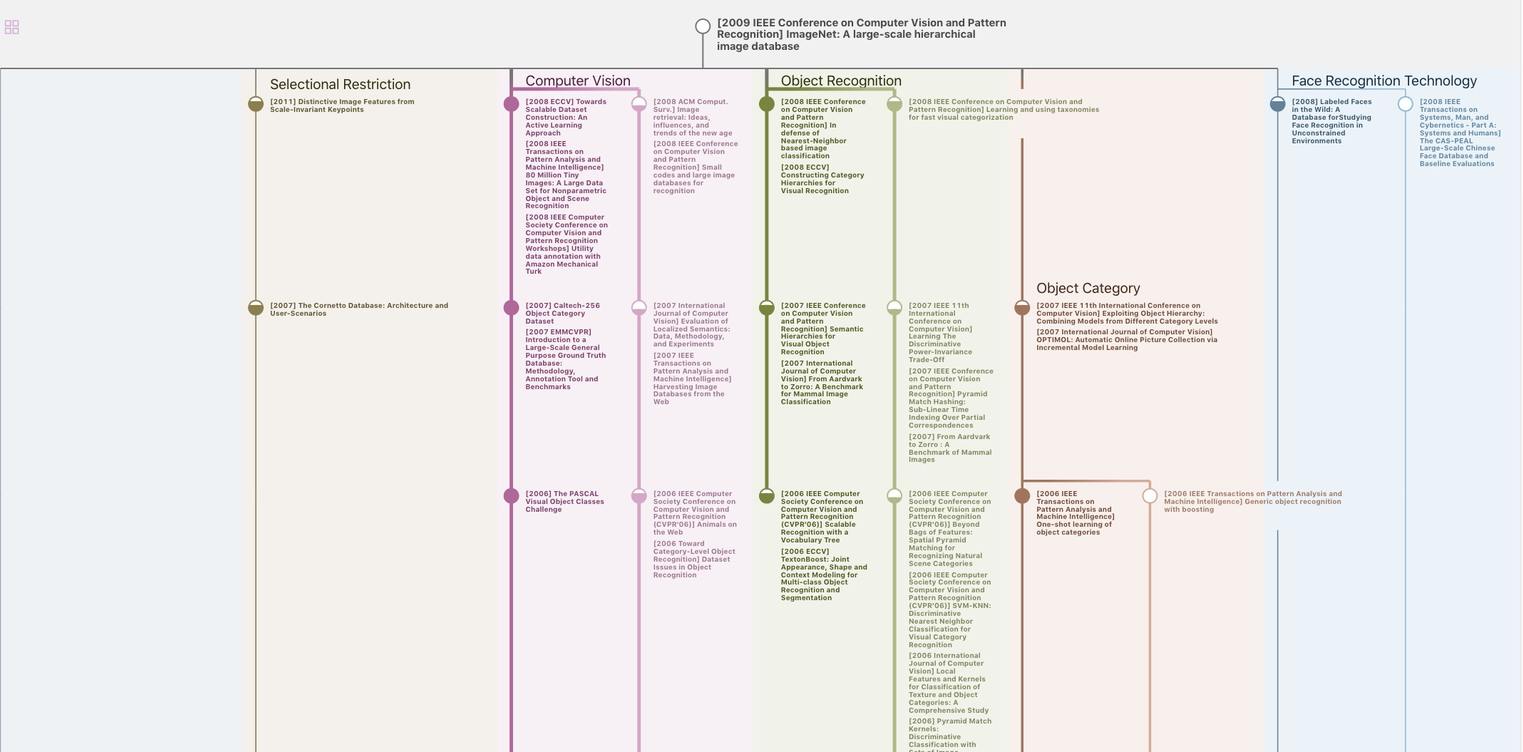
生成溯源树,研究论文发展脉络
Chat Paper
正在生成论文摘要