Estimating the Granularity Coefficient of a Potts-Markov Random Field Within a Markov Chain Monte Carlo Algorithm
IEEE Transactions on Image Processing(2013)CCF ASCI 1区
Univ Bristol | Univ Toulouse
Abstract
This paper addresses the problem of estimating the Potts parameter β jointly with the unknown parameters of a Bayesian model within a Markov chain Monte Carlo (MCMC) algorithm. Standard MCMC methods cannot be applied to this problem because performing inference on β requires computing the intractable normalizing constant of the Potts model. In the proposed MCMC method, the estimation of β is conducted using a likelihood-free Metropolis-Hastings algorithm. Experimental results obtained for synthetic data show that estimating β jointly with the other unknown parameters leads to estimation results that are as good as those obtained with the actual value of β. On the other hand, choosing an incorrect value of β can degrade estimation performance significantly. To illustrate the interest of this method, the proposed algorithm is successfully applied to real bidimensional SAR and tridimensional ultrasound images.
MoreTranslated text
Key words
Bayesian estimation,Gibbs sampler,intractable normalizing constants,mixture model,Potts-Markov field
PDF
View via Publisher
AI Read Science
AI Summary
AI Summary is the key point extracted automatically understanding the full text of the paper, including the background, methods, results, conclusions, icons and other key content, so that you can get the outline of the paper at a glance.
Example
Background
Key content
Introduction
Methods
Results
Related work
Fund
Key content
- Pretraining has recently greatly promoted the development of natural language processing (NLP)
- We show that M6 outperforms the baselines in multimodal downstream tasks, and the large M6 with 10 parameters can reach a better performance
- We propose a method called M6 that is able to process information of multiple modalities and perform both single-modal and cross-modal understanding and generation
- The model is scaled to large model with 10 billion parameters with sophisticated deployment, and the 10 -parameter M6-large is the largest pretrained model in Chinese
- Experimental results show that our proposed M6 outperforms the baseline in a number of downstream tasks concerning both single modality and multiple modalities We will continue the pretraining of extremely large models by increasing data to explore the limit of its performance
Try using models to generate summary,it takes about 60s
Must-Reading Tree
Example
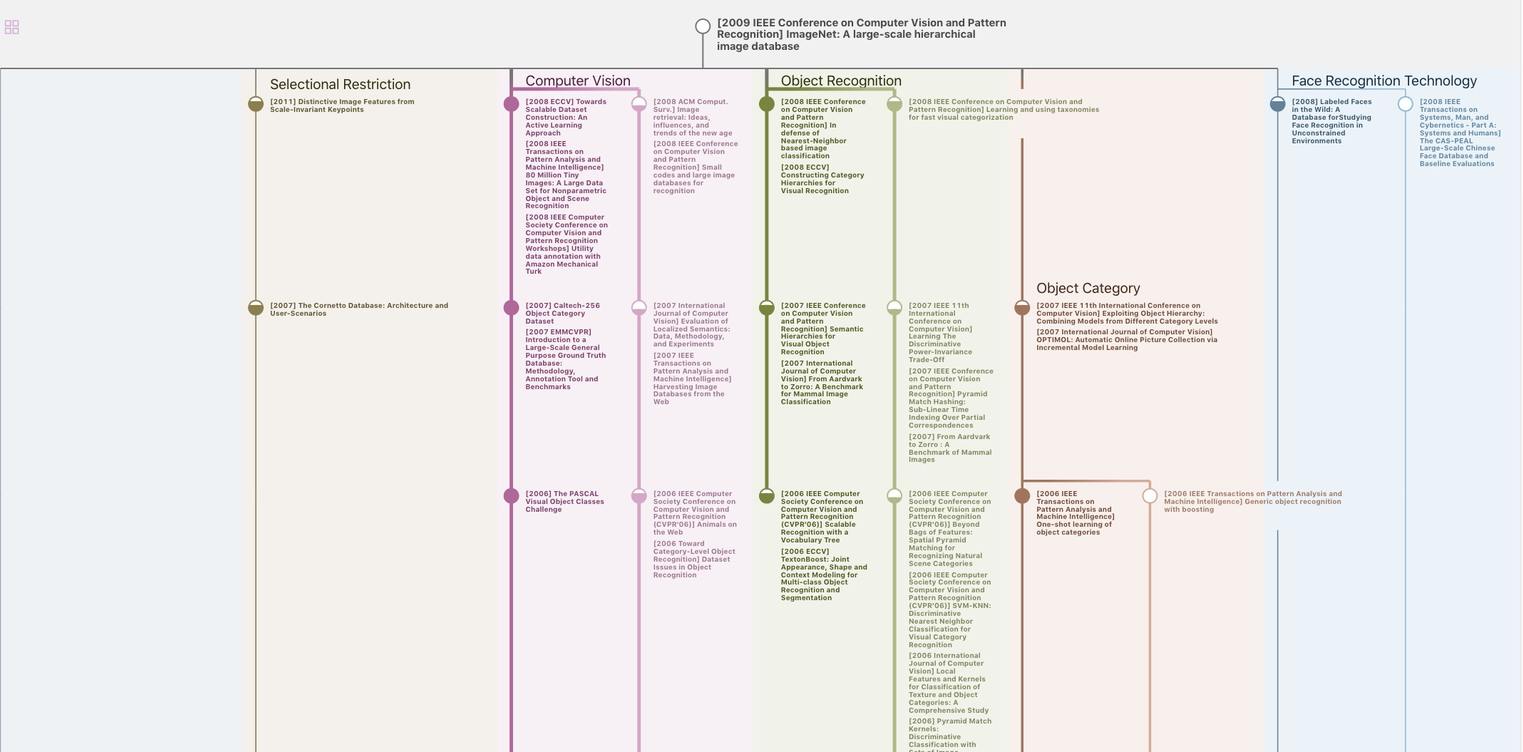
Generate MRT to find the research sequence of this paper
Related Papers
2003
被引用166 | 浏览
2003
被引用37 | 浏览
A Label Field Fusion Bayesian Model and Its Penalized Maximum Rand Estimator for Image Segmentation.
2010
被引用108 | 浏览
2007
被引用36 | 浏览
2008
被引用91 | 浏览
2011
被引用101 | 浏览
2012
被引用64 | 浏览
2004
被引用462 | 浏览
2006
被引用384 | 浏览
2011
被引用31 | 浏览
2003
被引用418 | 浏览
2010
被引用51 | 浏览
2010
被引用116 | 浏览
Data Disclaimer
The page data are from open Internet sources, cooperative publishers and automatic analysis results through AI technology. We do not make any commitments and guarantees for the validity, accuracy, correctness, reliability, completeness and timeliness of the page data. If you have any questions, please contact us by email: report@aminer.cn
Chat Paper
GPU is busy, summary generation fails
Rerequest