Using Rule-Based Context Knowledge To Model Table-Top Scenes
ICRA(2014)
摘要
In this paper, we propose a probabilistic method to generate abstract scene graphs for table-top scenes from 6D object pose estimates. We explicitly make use of task-specific context knowledge by encoding this knowledge as descriptive rules in Markov logic networks. Our approach to generate scene graphs is probabilistic: Uncertainty in the object poses is addressed by a probabilistic sensor model that is embedded in a data driven MCMC process. We apply Markov logic inference to reason about hidden objects and to detect false estimates of object poses. The effectiveness of our approach is demonstrated and evaluated in real world experiments.
更多查看译文
关键词
Markov processes,graph theory,knowledge based systems,pose estimation,6D object pose estimates,Markov logic inference,Markov logic networks,abstract scene graphs,data driven MCMC process,probabilistic sensor model,rule-based context knowledge,table-top scene model,task-specific context knowledge,
AI 理解论文
溯源树
样例
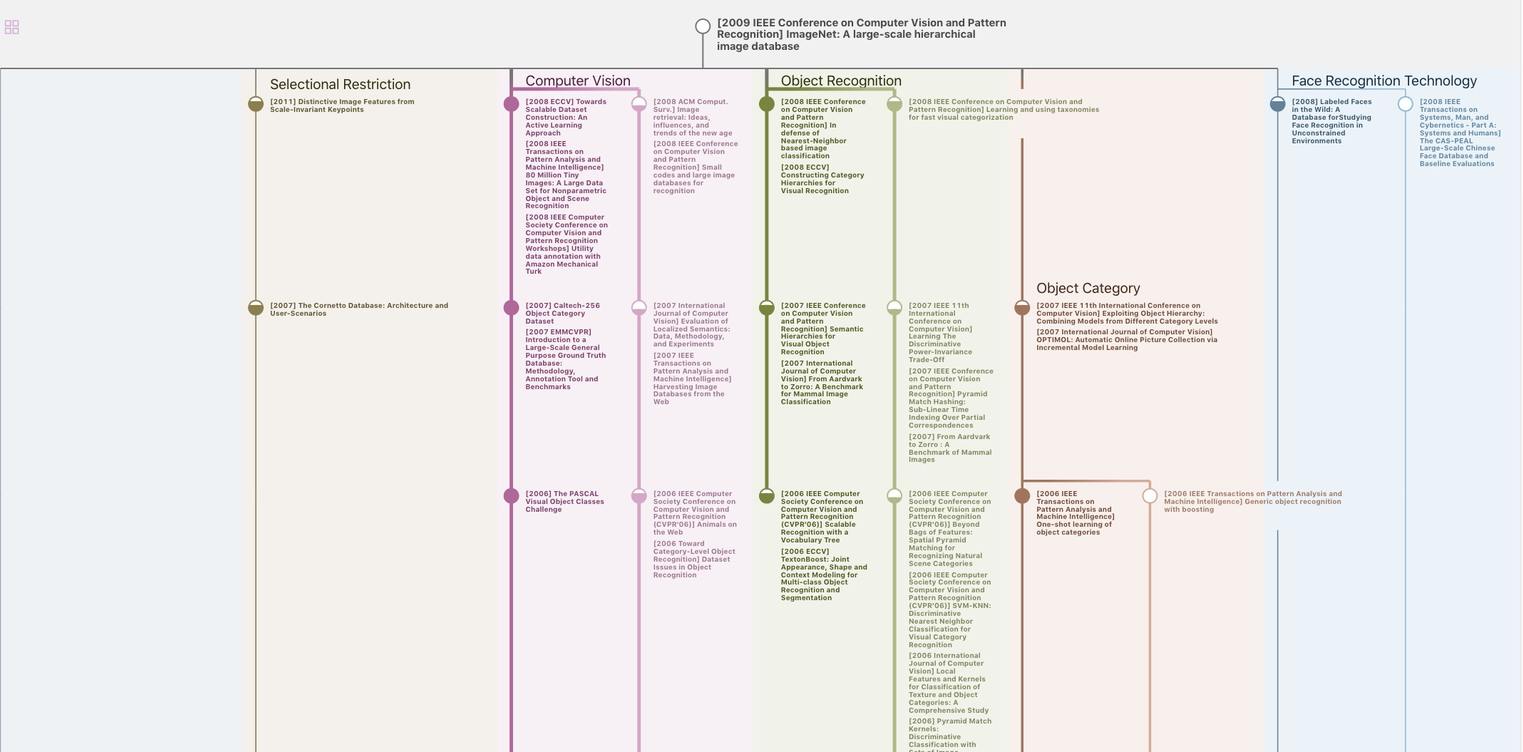
生成溯源树,研究论文发展脉络
Chat Paper
正在生成论文摘要