Exemplar-based human action pose correction.
IEEE T. Cybernetics(2014)
摘要
The launch of Xbox Kinect has built a very successful computer vision product and made a big impact on the gaming industry. This sheds lights onto a wide variety of potential applications related to action recognition. The accurate estimation of human poses from the depth image is universally a critical step. However, existing pose estimation systems exhibit failures when facing severe occlusion. In this paper, we propose an exemplar-based method to learn to correct the initially estimated poses. We learn an inhomogeneous systematic bias by leveraging the exemplar information within a specific human action domain. Furthermore, as an extension, we learn a conditional model by incorporation of pose tags to further increase the accuracy of pose correction. In the experiments, significant improvements on both joint-based skeleton correction and tag prediction are observed over the contemporary approaches, including what is delivered by the current Kinect system. Our experiments for the facial landmark correction also illustrate that our algorithm can improve the accuracy of other detection/estimation systems.
更多查看译文
关键词
random forest,exemplar-based human action pose correction,pose tags,xbox kinect,face recognition,facial landmark correction,joint-based skeleton correction,pose estimation system,kinect,gaming industry,skeleton,estimation theory,inhomogeneous systematic bias,pose estimation,exemplar information,pose tag,computer vision product,pose correction,detection system,computer vision,action recognition,gesture recognition,exemplar-based method,depth image,contemporary approach,occlusion,tag prediction,human pose estimation,human action domain,estimation,vegetation,systematics
AI 理解论文
溯源树
样例
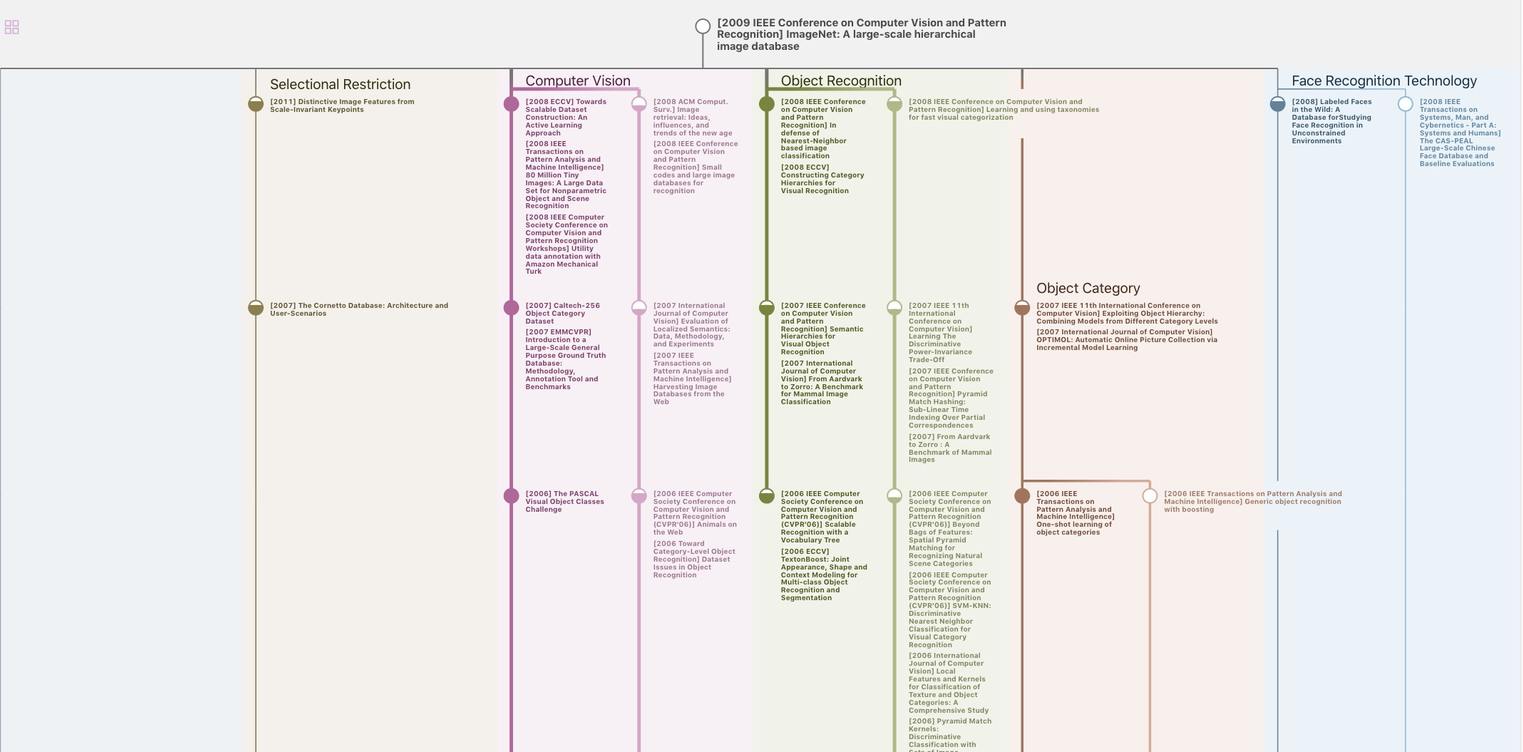
生成溯源树,研究论文发展脉络
Chat Paper
正在生成论文摘要