Prediction of Circulation Flow Rate in the Rh Degasser Using Discrete Phase Particle Modeling
ISIJ International(2009)SCI 3区
Abstract
Conservation equations for mass and momentum with a two equation k-epsilon model are solved for the continuous phase along with a discrete phase particle modeling (representing gas bubbles) in the RH degasser to predict the circulation flow rate of water in a scaled down model and then the numerical solution has been extended to the real plant case for the prediction of steel circulation flow rate in the actual RH vessel. The prediction of the circulation flow rate of water from the present numerical solution matches reasonably well with that of the experimental observation, taking into account various uncertainties those have been imbedded in the numerical model. RH operation for multi up legs and single down leg for a water model shows that the circulation flow rate falls with the number of up legs and there is an optimum number of down legs for which the circulation flow rate is the maximum for the case of a single up leg. For the actual RH operation in plant it was seen that the circulation flow rate increases with the increase in snorkel diameter and snorkel immersion depth (SID). However, it is apparent that there is existence of optimum SID for maximum circulation flow rate. For different down leg immersion depth the circulation flow rate in the RH depends heavily on the up leg immersion depth. The actual RH operation of the plant for the multi up leg and down leg cases was found to be exactly similar in nature to that of the water model cases.
MoreTranslated text
Key words
circulation flow rate,multi leg RH,discrete particle modeling
PDF
View via Publisher
AI Read Science
AI Summary
AI Summary is the key point extracted automatically understanding the full text of the paper, including the background, methods, results, conclusions, icons and other key content, so that you can get the outline of the paper at a glance.
Example
Background
Key content
Introduction
Methods
Results
Related work
Fund
Key content
- Pretraining has recently greatly promoted the development of natural language processing (NLP)
- We show that M6 outperforms the baselines in multimodal downstream tasks, and the large M6 with 10 parameters can reach a better performance
- We propose a method called M6 that is able to process information of multiple modalities and perform both single-modal and cross-modal understanding and generation
- The model is scaled to large model with 10 billion parameters with sophisticated deployment, and the 10 -parameter M6-large is the largest pretrained model in Chinese
- Experimental results show that our proposed M6 outperforms the baseline in a number of downstream tasks concerning both single modality and multiple modalities We will continue the pretraining of extremely large models by increasing data to explore the limit of its performance
Try using models to generate summary,it takes about 60s
Must-Reading Tree
Example
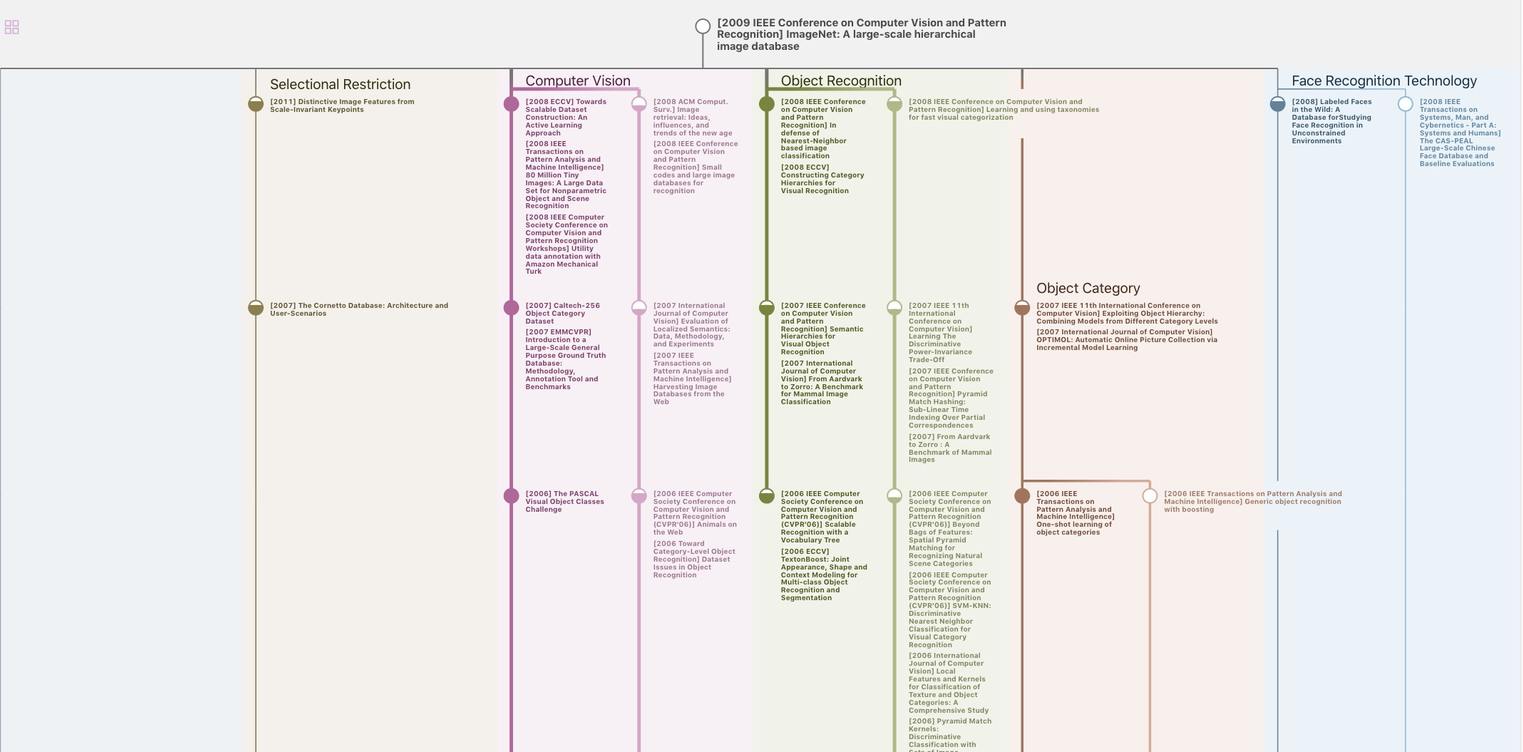
Generate MRT to find the research sequence of this paper
Related Papers
2001
被引用62 | 浏览
2000
被引用80 | 浏览
2006
被引用18 | 浏览
1983
被引用81 | 浏览
Data Disclaimer
The page data are from open Internet sources, cooperative publishers and automatic analysis results through AI technology. We do not make any commitments and guarantees for the validity, accuracy, correctness, reliability, completeness and timeliness of the page data. If you have any questions, please contact us by email: report@aminer.cn
Chat Paper
GPU is busy, summary generation fails
Rerequest