1-D Laterally Constrained Inversion of Em34 Profiling Data
Journal of Applied Geophysics(2004)SCI 3区
Univ Lisbon
- Pretraining has recently greatly promoted the development of natural language processing (NLP)
- We show that M6 outperforms the baselines in multimodal downstream tasks, and the large M6 with 10 parameters can reach a better performance
- We propose a method called M6 that is able to process information of multiple modalities and perform both single-modal and cross-modal understanding and generation
- The model is scaled to large model with 10 billion parameters with sophisticated deployment, and the 10 -parameter M6-large is the largest pretrained model in Chinese
- Experimental results show that our proposed M6 outperforms the baseline in a number of downstream tasks concerning both single modality and multiple modalities We will continue the pretraining of extremely large models by increasing data to explore the limit of its performance
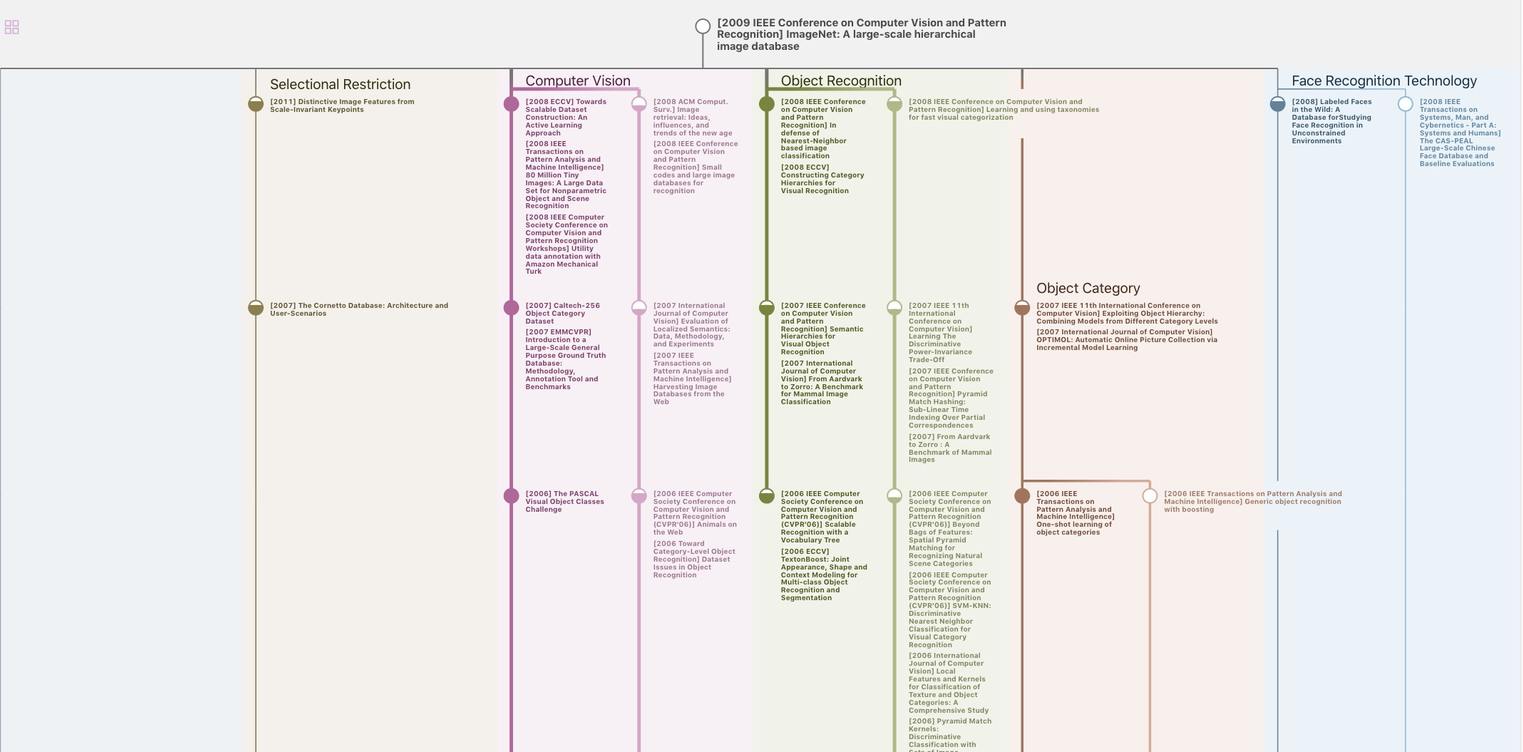
Laterally Constrained Inversion of Helicopter-Borne Frequency-Domain Electromagnetic Data
被引用84
Laterally Filtered 1D Inversions of Small-Loop, Frequency-Domain EMI Data from a Chemical Waste Site
被引用26
被引用21
A Lateral Model Parameter Correlation Procedure for One‐dimensional Inverse Modelling
被引用22
Robust 1D Inversion of Large Towed Geo-Electric Array Datasets Used for Hydrogeological Studies
被引用22
被引用28
被引用62
被引用23
被引用22
A Bayesian Trans-Dimensional Approach for the Fusion of Multiple Geophysical Datasets
被引用19
被引用61
Laterally constrained inversion for CSAMT data interpretation
被引用20
Electromagnetic Conductivity Imaging of Soil Salinity in an Estuarine–Alluvial Landscape
被引用19
被引用31
Preserving Horizontality Using Topography Corrected Laterally Constrained Inversion
被引用0
被引用26
被引用69
被引用5
被引用21
A Matlab-Based Frequency-Domain Electromagnetic Inversion Code (FEMIC) with Graphical User Interface
被引用23
被引用33
被引用26
被引用21
Modelling Soil Salinity Across a Gilgai Landscape by Inversion of EM38 and EM31 Data
被引用29