CC+: a Relational Database of Coiled-Coil Structures
Nucleic Acids Research(2009)SCI 2区
Abstract
We introduce the CC+ Database, a detailed, searchable repository of coiled-coil assignments, which is freely available at http://coiledcoils.chm.bris.ac.uk/ccplus. Coiled coils were identified using the program SOCKET, which locates coiled coils based on knobs-into-holes packing of side chains between α-helices. A method for determining the overall sequence identity of coiled-coil sequences was introduced to reduce statistical bias inherent in coiled-coil data sets. There are two points of entry into the CC+ Database: the ‘Periodic Table of Coiled-coil Structures’, which presents a graphical path through coiled-coil space based on manually validated data, and the ‘Dynamic Interface’, which allows queries of the database at different levels of complexity and detail. The latter entry level, which is the focus of this article, enables the efficient and rapid compilation of subsets of coiled-coil structures. These can be created and interrogated with increasingly sophisticated pull-down, keyword and sequence-based searches to return detailed structural and sequence information. Also provided are means for outputting the retrieved coiled-coil data in various formats, including PyMOL and RasMol scripts, and Position-Specific Scoring Matrices (or amino-acid profiles), which may be used, for example, in protein-structure prediction.
MoreTranslated text
Key words
Support Vector Machines
PDF
View via Publisher
AI Read Science
AI Summary
AI Summary is the key point extracted automatically understanding the full text of the paper, including the background, methods, results, conclusions, icons and other key content, so that you can get the outline of the paper at a glance.
Example
Background
Key content
Introduction
Methods
Results
Related work
Fund
Key content
- Pretraining has recently greatly promoted the development of natural language processing (NLP)
- We show that M6 outperforms the baselines in multimodal downstream tasks, and the large M6 with 10 parameters can reach a better performance
- We propose a method called M6 that is able to process information of multiple modalities and perform both single-modal and cross-modal understanding and generation
- The model is scaled to large model with 10 billion parameters with sophisticated deployment, and the 10 -parameter M6-large is the largest pretrained model in Chinese
- Experimental results show that our proposed M6 outperforms the baseline in a number of downstream tasks concerning both single modality and multiple modalities We will continue the pretraining of extremely large models by increasing data to explore the limit of its performance
Try using models to generate summary,it takes about 60s
Must-Reading Tree
Example
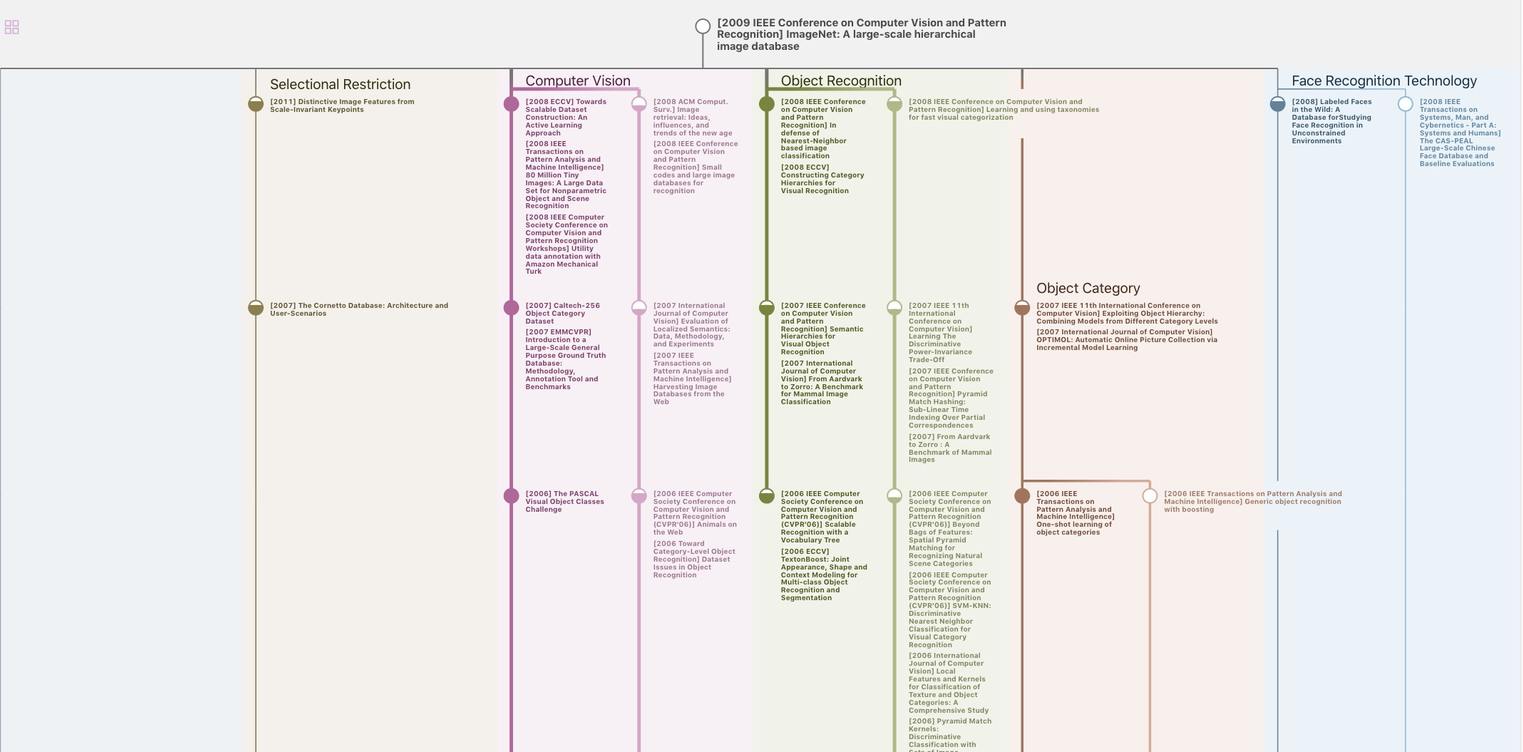
Generate MRT to find the research sequence of this paper
Related Papers
2006
被引用29 | 浏览
1990
被引用173 | 浏览
2001
被引用350 | 浏览
1983
被引用18001 | 浏览
1991
被引用90 | 浏览
2004
被引用74 | 浏览
1993
被引用1534 | 浏览
Data Disclaimer
The page data are from open Internet sources, cooperative publishers and automatic analysis results through AI technology. We do not make any commitments and guarantees for the validity, accuracy, correctness, reliability, completeness and timeliness of the page data. If you have any questions, please contact us by email: report@aminer.cn
Chat Paper
去 AI 文献库 对话