Perturbation Defocusing for Adversarial Defense
ICLR 2023(2023)
摘要
Recent research indicates adversarial attacks are likely to deceive neural systems, including large-scale, pre-trained language models. Given a natural sentence, an attacker replaces a subset of words to fool objective models. To defend against adversarial attacks, existing works aim to reconstruct the adversarial examples. However, these methods show limited defense performance on the adversarial examples whilst also damaging the clean performance on natural examples. To achieve better defense performance, our finding indicates that the reconstruction of adversarial examples is not necessary. More specifically, we inject non-toxic perturbations into adversarial examples, which can disable almost all malicious perturbations. In order to minimize performance sacrifice, we employ an adversarial example detector to distinguish and repair detected adversarial examples, which alleviates the mis-defense on natural examples. Our experimental results on three datasets, two objective models and a variety of adversarial attacks show that the proposed method successfully repairs up to ∼ 97% correctly identified adversarial examples with ≤∼ 2% performance sacrifice. We provide an anony-mus demonstration of adversarial detection and repair based on our work.
更多查看译文
关键词
text adversarial defense,perturbation defocusing
AI 理解论文
溯源树
样例
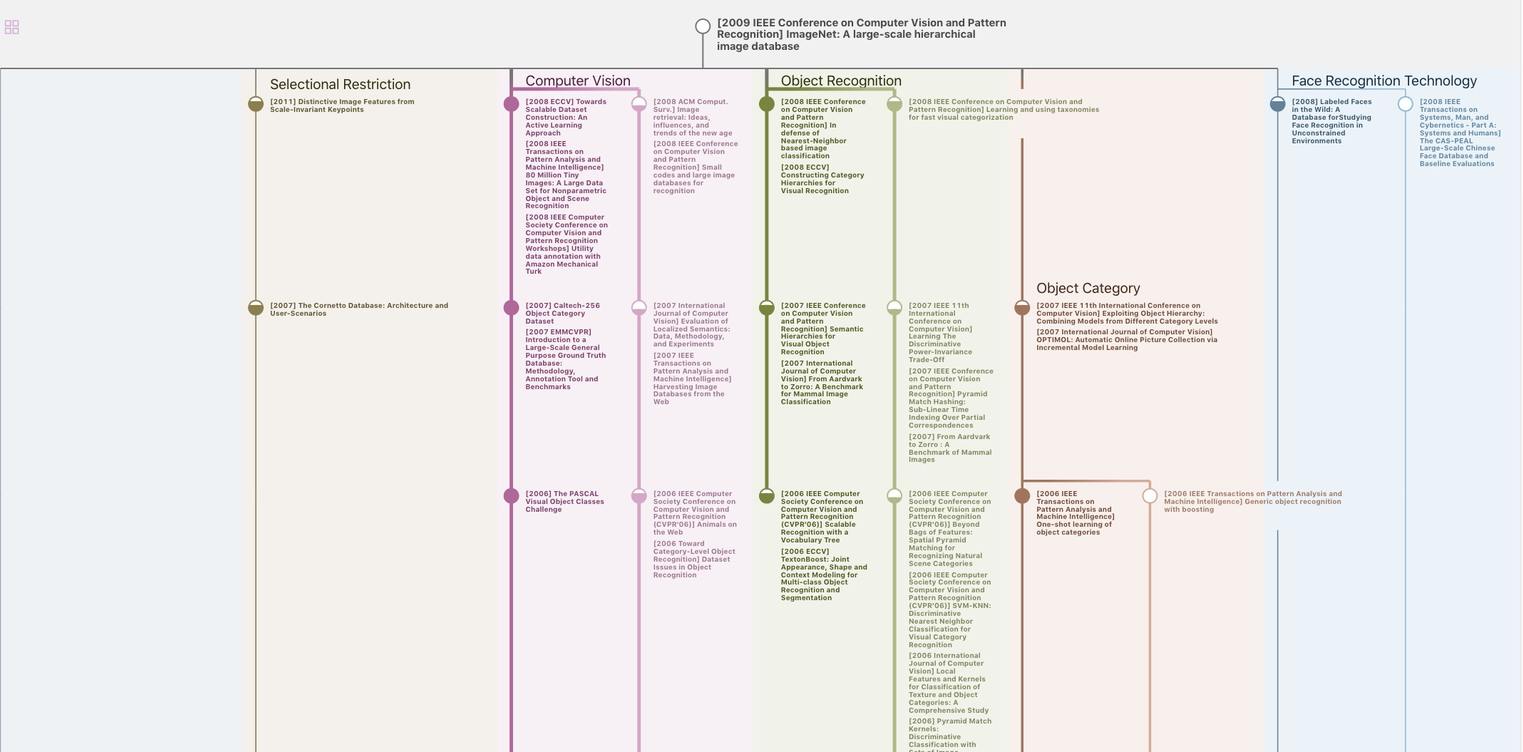
生成溯源树,研究论文发展脉络
Chat Paper
正在生成论文摘要