The Precision-Recall Plot is More Informative Than the ROC Plot when Evaluating Binary Classifiers on Imbalanced Datasets.
PLoS ONE(2015)SCI 3区
Abstract
Binary classifiers are routinely evaluated with performance measures such as sensitivity and specificity, and performance is frequently illustrated with Receiver Operating Characteristics (ROC) plots. Alternative measures such as positive predictive value (PPV) and the associated Precision/Recall (PRC) plots are used less frequently. Many bioinformatics studies develop and evaluate classifiers that are to be applied to strongly imbalanced datasets in which the number of negatives outweighs the number of positives significantly. While ROC plots are visually appealing and provide an overview of a classifier's performance across a wide range of specificities, one can ask whether ROC plots could be misleading when applied in imbalanced classification scenarios. We show here that the visual interpretability of ROC plots in the context of imbalanced datasets can be deceptive with respect to conclusions about the reliability of classification performance, owing to an intuitive but wrong interpretation of specificity. PRC plots, on the other hand, can provide the viewer with an accurate prediction of future classification performance due to the fact that they evaluate the fraction of true positives among positive predictions. Our findings have potential implications for the interpretation of a large number of studies that use ROC plots on imbalanced datasets.
MoreTranslated text
PDF
View via Publisher
AI Read Science
AI Summary
AI Summary is the key point extracted automatically understanding the full text of the paper, including the background, methods, results, conclusions, icons and other key content, so that you can get the outline of the paper at a glance.
Example
Background
Key content
Introduction
Methods
Results
Related work
Fund
Key content
- Pretraining has recently greatly promoted the development of natural language processing (NLP)
- We show that M6 outperforms the baselines in multimodal downstream tasks, and the large M6 with 10 parameters can reach a better performance
- We propose a method called M6 that is able to process information of multiple modalities and perform both single-modal and cross-modal understanding and generation
- The model is scaled to large model with 10 billion parameters with sophisticated deployment, and the 10 -parameter M6-large is the largest pretrained model in Chinese
- Experimental results show that our proposed M6 outperforms the baseline in a number of downstream tasks concerning both single modality and multiple modalities We will continue the pretraining of extremely large models by increasing data to explore the limit of its performance
Try using models to generate summary,it takes about 60s
Must-Reading Tree
Example
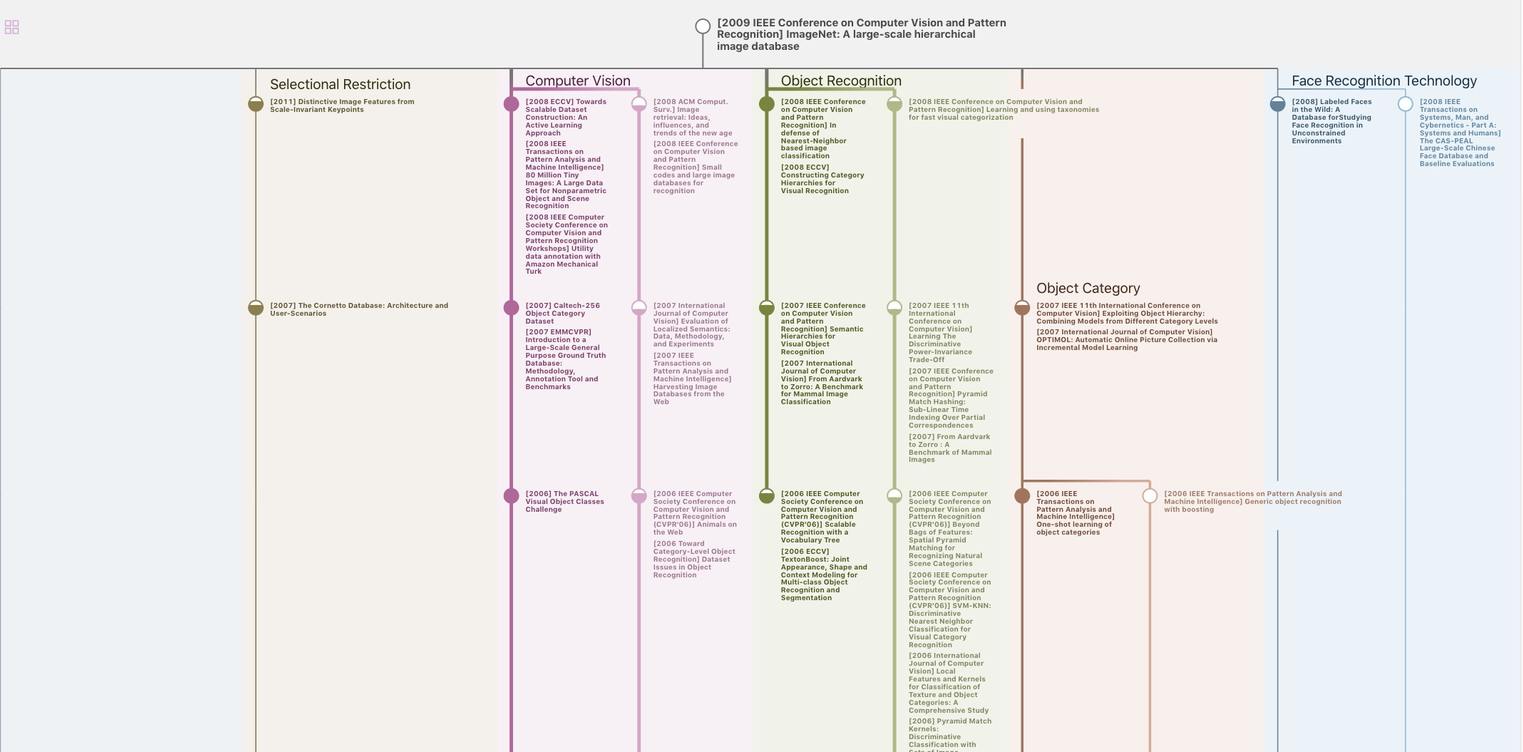
Generate MRT to find the research sequence of this paper
Related Papers
2017
被引用1 | 浏览
2017
被引用7 | 浏览
2017
被引用21 | 浏览
2017
被引用97 | 浏览
2018
被引用30 | 浏览
2018
被引用16 | 浏览
2018
被引用46 | 浏览
2018
被引用74 | 浏览
2018
被引用11 | 浏览
2018
被引用59 | 浏览
2018
被引用9 | 浏览
2019
被引用44 | 浏览
2019
被引用31 | 浏览
2018
被引用10 | 浏览
Data Disclaimer
The page data are from open Internet sources, cooperative publishers and automatic analysis results through AI technology. We do not make any commitments and guarantees for the validity, accuracy, correctness, reliability, completeness and timeliness of the page data. If you have any questions, please contact us by email: report@aminer.cn
Chat Paper
去 AI 文献库 对话