Learning Models for Electron Densities with Bayesian Regression
Computational Materials Science(2018)SCI 3区
Abstract
The Hohenberg-Kohn theorems posit the ground state electron density as a property of fundamental importance in condensed matter physics, finding widespread application in much of solid state physics in the form of density functional theory (DFT) and, at least in principle, in semi-empirical potentials such as the Embedded Atom Method (EAM). Using machine learning algorithms based on parametric linear models, we propose a systematic approach to developing such potentials for binary alloys based on DFT electron densities, as well as energies and forces. The approach is demonstrated on the technologically important Al-Ni alloy system. We further demonstrate how ground state electron densities, obtained with DFT, can be predicted such that total energies have an accuracy of order meV atom(-1) for crystalline structures. The set of crystalline structures includes a range of materials representing different phases and bonding types, from Al structures to single-wall carbon nanotubes.
MoreTranslated text
Key words
Bayesian linear regression,Relevance vector machine,Density functional theory,Embedded atom method,Genetic algorithm
PDF
View via Publisher
AI Read Science
AI Summary
AI Summary is the key point extracted automatically understanding the full text of the paper, including the background, methods, results, conclusions, icons and other key content, so that you can get the outline of the paper at a glance.
Example
Background
Key content
Introduction
Methods
Results
Related work
Fund
Key content
- Pretraining has recently greatly promoted the development of natural language processing (NLP)
- We show that M6 outperforms the baselines in multimodal downstream tasks, and the large M6 with 10 parameters can reach a better performance
- We propose a method called M6 that is able to process information of multiple modalities and perform both single-modal and cross-modal understanding and generation
- The model is scaled to large model with 10 billion parameters with sophisticated deployment, and the 10 -parameter M6-large is the largest pretrained model in Chinese
- Experimental results show that our proposed M6 outperforms the baseline in a number of downstream tasks concerning both single modality and multiple modalities We will continue the pretraining of extremely large models by increasing data to explore the limit of its performance
Try using models to generate summary,it takes about 60s
Must-Reading Tree
Example
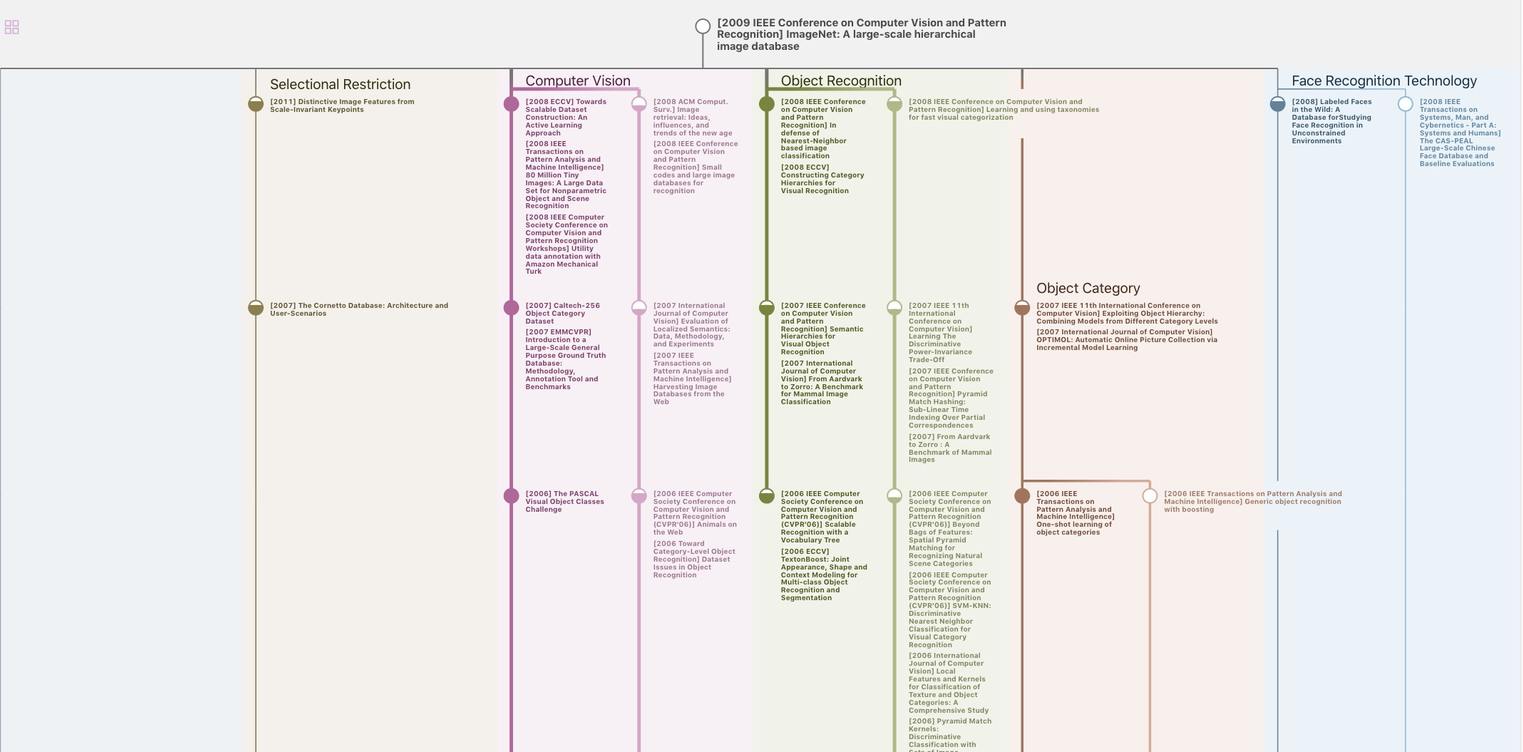
Generate MRT to find the research sequence of this paper
Related Papers
2009
被引用1862 | 浏览
2005
被引用278 | 浏览
2014
被引用531 | 浏览
2008
被引用99 | 浏览
1984
被引用9617 | 浏览
2013
被引用11443 | 浏览
2012
被引用942 | 浏览
Data Disclaimer
The page data are from open Internet sources, cooperative publishers and automatic analysis results through AI technology. We do not make any commitments and guarantees for the validity, accuracy, correctness, reliability, completeness and timeliness of the page data. If you have any questions, please contact us by email: report@aminer.cn
Chat Paper
去 AI 文献库 对话