TANE: an Efficient Algorithm for Discovering Functional and Approximate Dependencies
Computer Journal(1999)CCF BSCI 4区
- Pretraining has recently greatly promoted the development of natural language processing (NLP)
- We show that M6 outperforms the baselines in multimodal downstream tasks, and the large M6 with 10 parameters can reach a better performance
- We propose a method called M6 that is able to process information of multiple modalities and perform both single-modal and cross-modal understanding and generation
- The model is scaled to large model with 10 billion parameters with sophisticated deployment, and the 10 -parameter M6-large is the largest pretrained model in Chinese
- Experimental results show that our proposed M6 outperforms the baseline in a number of downstream tasks concerning both single modality and multiple modalities We will continue the pretraining of extremely large models by increasing data to explore the limit of its performance
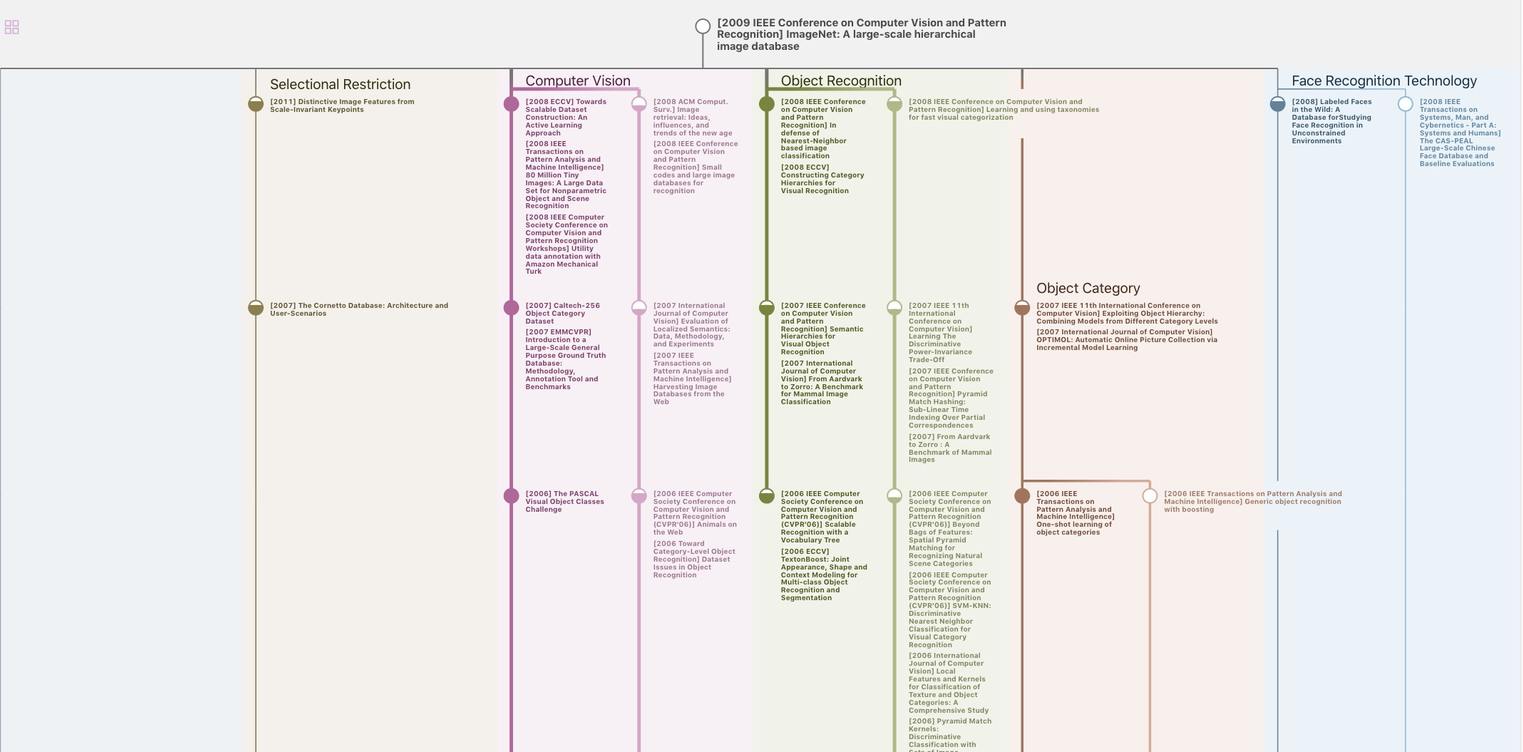
SQL-based Discovery of Exact and Approximate Functional Dependencies
被引用14
An Optimized Approach for Extracting Approximate Functional Dependencies in XML Documents
被引用0
INF ERENCE INCR EMENTALE DES D EPENDANCES FONCTIONNELLES DANS UNE BASE DE DONN EES RELATIONNELLE
被引用23
被引用24
Functional Dependency Generation and Applications in Pay-As-You-Go Data Integration Systems.
被引用71
Using Transversals for Discovering XML Functional Dependencies
被引用12
Defining and Mining Functional Dependencies in Probabilistic Databases
被引用25
被引用3
Extracting Functional Dependencies in Large Datasets Using MapReduce Model
被引用13
被引用23
Defining Key Semantics for the RDF Datasets: Experiments and Evaluations
被引用18
SAKey: Scalable Almost Key Discovery in RDF Data
被引用72
Efficient Keyword Search Across Heterogeneous Relational Databases
被引用161
Relaxed Functional Dependencies—A Survey of Approaches
被引用141
FD/spl I.bar/Mine: Discovering Functional Dependencies in a Database Using Equivalences
被引用57
CrowdMD: Crowdsourcing-based Approach for Deduplication
被引用7