Gaia Broad Band Photometry
Astronomy and Astrophysics(2010)SCI 2区
Univ Barcelona | European Space Agcy | ICC UB | Univ Copenhagen | INAF
Abstract
The scientific community needs to be prepared to analyse the data from Gaia, one of the most ambitious ESA space missions, to be launched in 2012. The purpose of this paper is to provide data and tools in order to predict in advance how Gaia photometry is expected to be. To do so, we provide relationships among colours involving Gaia magnitudes and colours from other commonly used photometric systems (Johnson-Cousins, SDSS, Hipparcos and Tycho). The most up-to-date information from industrial partners has been used to define the nominal passbands and based on the BaSeL3.1 stellar spectral energy distribution library, relationships were obtained for stars with different reddening values, ranges of temperatures, surface gravities and metallicities. The transformations involving Gaia and Johnson-Cousins V-I_C and Sloan DSS g-z colours have the lowest residuals. A polynomial expression for the relation between the effective temperature and the colour G_BP-G_RP was derived for stars with T > 4500 K. Transformations involving two Johnson or two Sloan DSS colours yield lower residuals than using only one colour. We also computed several ratios of total-to-selective absorption including absorption A_G in the G band and colour excess E(G_BP-G_RP) for our sample stars. A relationship, involving A_G/A_V and the intrinsic (V-I_C) colour, is provided. The derived Gaia passbands have been used to compute tracks and isochrones using the Padova and BASTI models. Finally, the performances of the predicted Gaia magnitudes have been estimated according to the magnitude and the celestial coordinates of the star. The provided dependencies among colours can be used for planning scientific exploitation of Gaia data, performing simulations of the Gaia-like sky, planning ground-based complementary observations and for building catalogues with auxiliary data for the Gaia data processing and validation.
MoreTranslated text
Key words
instrumentation: photometers,techniques: photometric,Galaxy: general,dust, extinction,stars: evolution
PDF
View via Publisher
AI Read Science
AI Summary
AI Summary is the key point extracted automatically understanding the full text of the paper, including the background, methods, results, conclusions, icons and other key content, so that you can get the outline of the paper at a glance.
Example
Background
Key content
Introduction
Methods
Results
Related work
Fund
Key content
- Pretraining has recently greatly promoted the development of natural language processing (NLP)
- We show that M6 outperforms the baselines in multimodal downstream tasks, and the large M6 with 10 parameters can reach a better performance
- We propose a method called M6 that is able to process information of multiple modalities and perform both single-modal and cross-modal understanding and generation
- The model is scaled to large model with 10 billion parameters with sophisticated deployment, and the 10 -parameter M6-large is the largest pretrained model in Chinese
- Experimental results show that our proposed M6 outperforms the baseline in a number of downstream tasks concerning both single modality and multiple modalities We will continue the pretraining of extremely large models by increasing data to explore the limit of its performance
Try using models to generate summary,it takes about 60s
Must-Reading Tree
Example
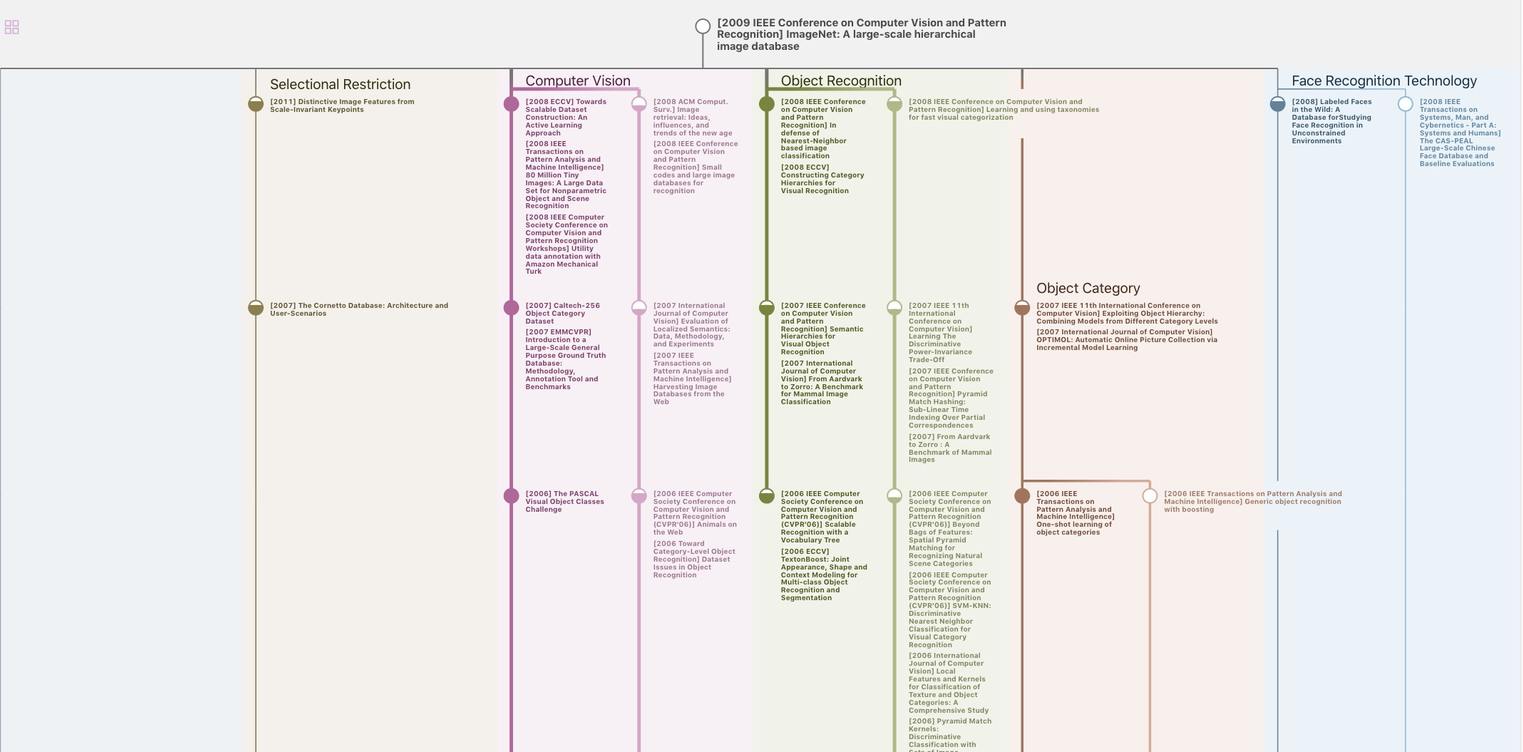
Generate MRT to find the research sequence of this paper
Related Papers
2019
被引用38 | 浏览
2021
被引用17 | 浏览
2021
被引用21 | 浏览
Data Disclaimer
The page data are from open Internet sources, cooperative publishers and automatic analysis results through AI technology. We do not make any commitments and guarantees for the validity, accuracy, correctness, reliability, completeness and timeliness of the page data. If you have any questions, please contact us by email: report@aminer.cn
Chat Paper
去 AI 文献库 对话