ANSWERING THE SKEPTICS: YES, STANDARD VOLATILITY MODELS DO PROVIDE ACCURATE FORECASTS*
International Economic Review(1998)SCI 3区
Abstract
A voluminous literature has emerged for modeling the temporal dependencies in financial market Volatility using ARCH and stochastic volatility models. While most of these studies have documented highly significant in-sample parameter estimates and pronounced intertemporal volatility persistence, traditional ex-post forecast evaluation criteria suggest that the models provide seemingly poor volatility forecasts. Contrary to this contention, we show that volatility models produce strikingly accurate interdaily forecasts for the latent volatility factor that would be of interest in most financial applications. New methods for improved ex-post interdaily volatility measurements based on high-frequency intradaily data are also discussed.
MoreTranslated text
求助PDF
上传PDF
View via Publisher
AI Read Science
AI Summary
AI Summary is the key point extracted automatically understanding the full text of the paper, including the background, methods, results, conclusions, icons and other key content, so that you can get the outline of the paper at a glance.
Example
Background
Key content
Introduction
Methods
Results
Related work
Fund
Key content
- Pretraining has recently greatly promoted the development of natural language processing (NLP)
- We show that M6 outperforms the baselines in multimodal downstream tasks, and the large M6 with 10 parameters can reach a better performance
- We propose a method called M6 that is able to process information of multiple modalities and perform both single-modal and cross-modal understanding and generation
- The model is scaled to large model with 10 billion parameters with sophisticated deployment, and the 10 -parameter M6-large is the largest pretrained model in Chinese
- Experimental results show that our proposed M6 outperforms the baseline in a number of downstream tasks concerning both single modality and multiple modalities We will continue the pretraining of extremely large models by increasing data to explore the limit of its performance
Upload PDF to Generate Summary
Must-Reading Tree
Example
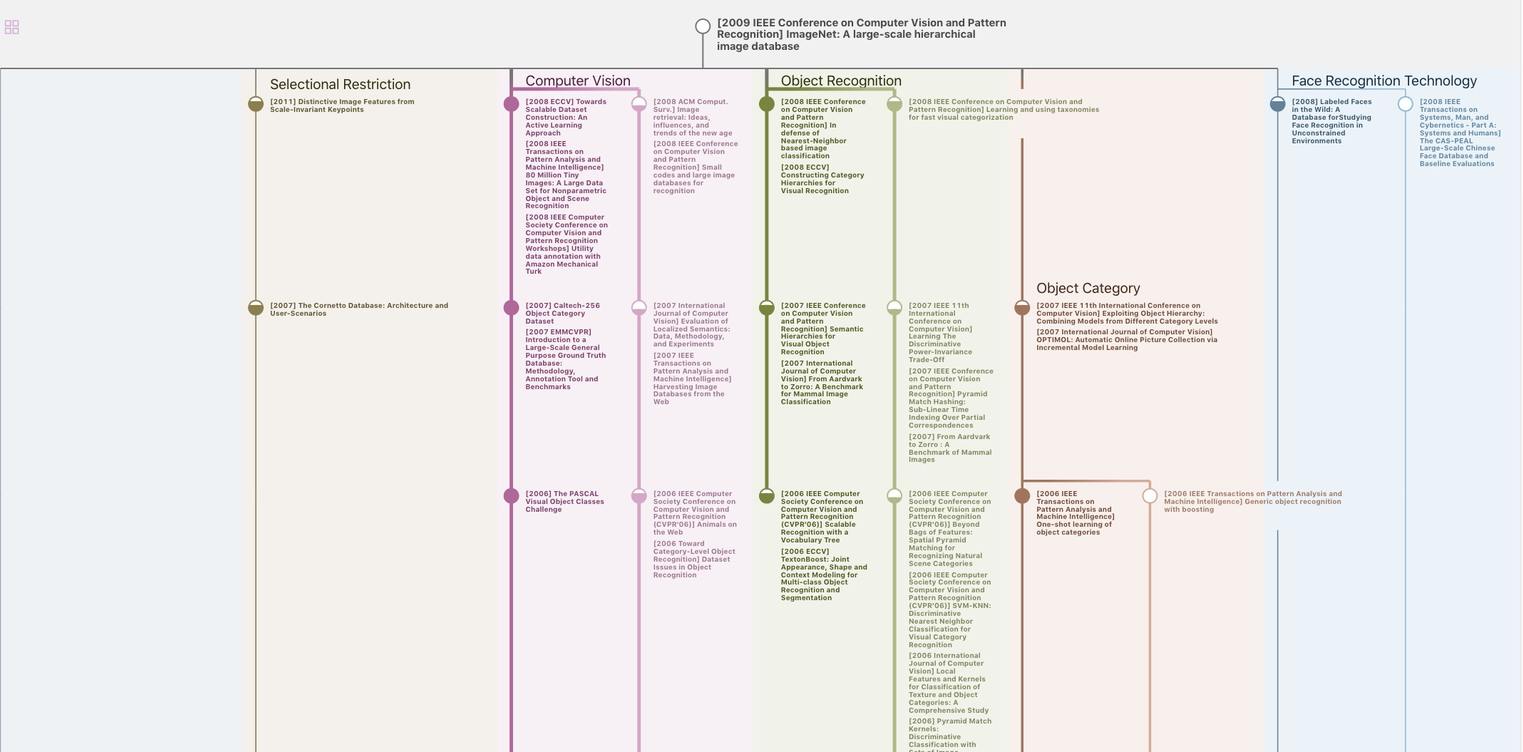
Generate MRT to find the research sequence of this paper
Related Papers
Foreign Exchange, Fractional Cointegration and the Implied-Realized Volatility Relation
Journal of Banking & Finance 2009
被引用32
International Journal of Forecasting 2009
被引用148
Journal of International Financial Markets, Institutions and Money 2009
被引用82
RePEc: Research Papers in Economics 2004
被引用1
Explanations of the Inconsistencies in Survey Respondents'forecasts
European Economic Review 2009
被引用57
Economics Letters 2004
被引用58
EMERGING MARKETS FINANCE AND TRADE 2007
被引用17
Neural Network Load Forecasting with Weather Ensemble Predictions
IEEE Power Engineering Review 2002
被引用572
Forecasting the Comovements of Spot Interest Rates
JOURNAL OF INTERNATIONAL MONEY AND FINANCE 2005
被引用14
Long Memory Dynamics for Multivariate Dependence under Heavy Tails
Journal of Empirical Finance 2014
被引用40
Forecasting with the Standardized Self-Perturbed Kalman Filter
JOURNAL OF APPLIED ECONOMETRICS 2017
被引用9
Data Disclaimer
The page data are from open Internet sources, cooperative publishers and automatic analysis results through AI technology. We do not make any commitments and guarantees for the validity, accuracy, correctness, reliability, completeness and timeliness of the page data. If you have any questions, please contact us by email: report@aminer.cn
Chat Paper