A Block Coordinate Descent Method for Regularized Multiconvex Optimization with Applications to Nonnegative Tensor Factorization and Completion
SIAM Journal on Imaging Sciences(2013)CCF BSCI 3区SCI 2区
- Pretraining has recently greatly promoted the development of natural language processing (NLP)
- We show that M6 outperforms the baselines in multimodal downstream tasks, and the large M6 with 10 parameters can reach a better performance
- We propose a method called M6 that is able to process information of multiple modalities and perform both single-modal and cross-modal understanding and generation
- The model is scaled to large model with 10 billion parameters with sophisticated deployment, and the 10 -parameter M6-large is the largest pretrained model in Chinese
- Experimental results show that our proposed M6 outperforms the baseline in a number of downstream tasks concerning both single modality and multiple modalities We will continue the pretraining of extremely large models by increasing data to explore the limit of its performance
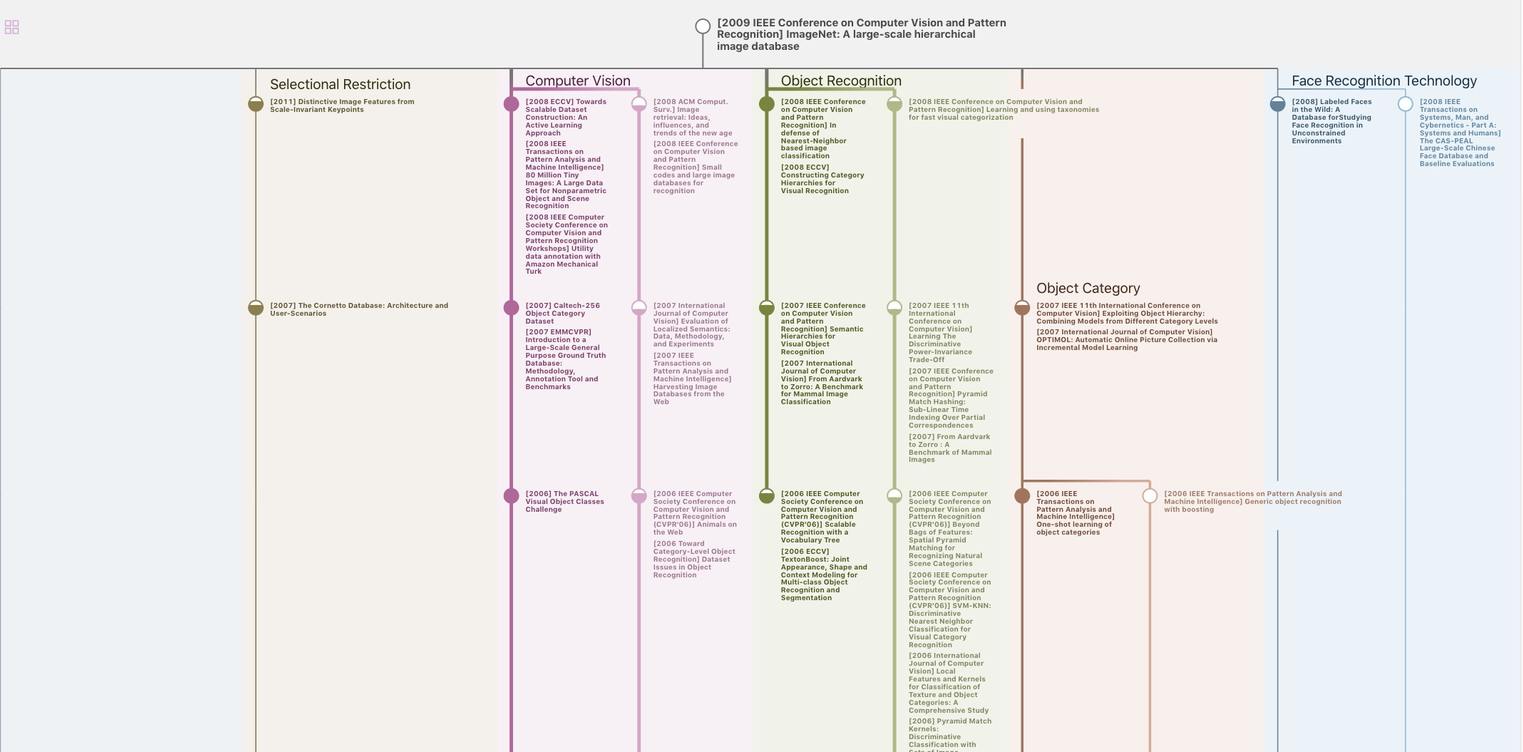
A Convergent Least-Squares Regularized Blind Deconvolution Approach
被引用9
Tag-Aware Ordinal Sparse Factor Analysis for Learning and Content Analytics.
被引用27
Ipiano: Inertial Proximal Algorithm for Nonconvex Optimization.
被引用422
A Hybrid Alternating Proximal Method for Blind Video Restoration
被引用9
Discovering Urban Spatio-temporal Structure from Time-Evolving Traffic Networks
被引用49
Matrix Recovery from Quantized and Corrupted Measurements
被引用47
Subgraph Augmented Non-Negative Tensor Factorization (SANTF) for Modeling Clinical Narrative Text
被引用63
被引用368
Sparse Regularization: Convergence of Iterative Jumping Thresholding Algorithm
被引用48
Global Optimality in Tensor Factorization, Deep Learning, and Beyond
被引用153
Block Stochastic Gradient Iteration for Convex and Nonconvex Optimization.
被引用149
A Compact Binary Aggregated Descriptor Via Dual Selection for Visual Search
被引用2
被引用52
被引用6
Structurally Regularized Non-negative Tensor Factorization for Spatio-Temporal Pattern Discoveries
被引用12
被引用23
Increasing Stability of EEG Components Extraction Using Sparsity Regularized Tensor Decomposition
被引用3
被引用22
Accelerating Nonnegative Matrix Factorization Algorithms Using Extrapolation
被引用44
PET-MRI Joint Reconstruction by Joint Sparsity Based Tight Frame Regularization
被引用7
被引用12
Tensor-Based Channel Estimation for Dual-Polarized Massive MIMO Systems
被引用46
被引用29
Sparse-spike Deconvolution Via Curvelet Transform and Sparse-Matrix Factorization
被引用0
Total Variation Regularization-Based Compressed Sensing Synthetic Aperture Radar Imaging
被引用10