Interpretable Image Classification Model Using Formal Concept Analysis Based Classifier
EPiC Series in Computing(2022)
摘要
Massive amounts of data gathered over the last decade have contributed significantly to the applicability of deep neural networks. Deep learning is a good technique to process huge amounts of data because they get better as we feed more data into them. However, in the existing literature, a deep neural classifier is often treated as a ”black box” technique because the process is not transparent and the researchers cannot gain information about how the input is associated to the output. In many domains like medicine, interpretability is very critical because of the nature of the application. Our research focuses on adding interpretability to the black box by integrating Formal Concept Analysis (FCA) into the image classification pipeline and convert it into a glass box. Our proposed approach pro- duces a low dimensional feature vector for an image dataset using autoencoder followed by a supervised fine-tuning of features using a deep neural classifier and Linear Discriminant Analysis (LDA). The low dimensional feature vector produced is then processed by FCA based classifier. The FCA framework helps us develop a glass box classifier from which the relationship between the target class and the low dimensional feature set can be derived. Further, it helps the researchers to understand the classification task and refine it. We use the MNIST dataset to test the interfacing between deep neural networks and the FCA classifier. The classifier achieves an accuracy of 98.7% for binary classification and 97.38% for multi-class classification. We compare the performance of the proposed classifier with Convolutional neural networks (CNN) and Random forest.
更多查看译文
关键词
interpretable image classification model,formal concept analysis,classifier
AI 理解论文
溯源树
样例
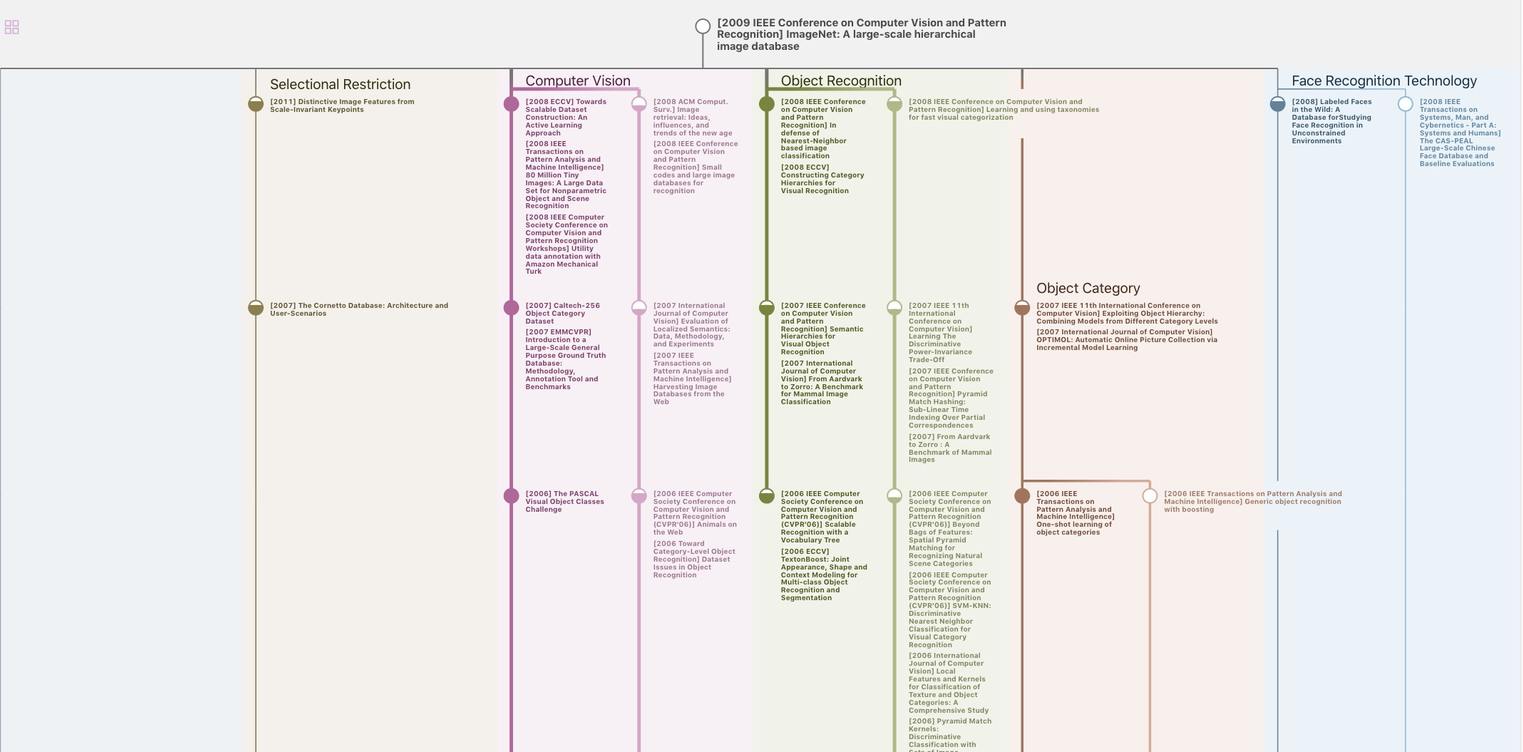
生成溯源树,研究论文发展脉络
Chat Paper
正在生成论文摘要