Impact of chronic inflammation on the pharmacokinetic–pharmacodynamic relationship of naproxen
European Journal of Pain(2010)
摘要
Methods Rats were treated with Freund’s complete adjuvant (FCA) by intraplantar injection. On post-inoculation days (PID) 7–21, animals received single or chronic (qd until day 21) doses of naproxen (10 mg/kg). Blood samples were collected at various intervals after dosing to characterise naproxen pharmacokinetics and its effects on PGE 2 and TXB 2 production. PK-PD modelling was performed using nonlinear mixed effects in NONMEM. Results The inhibition of PGE 2 and TXB 2 could be described by a sigmoid E max model. A decrease in the potency estimates of both biomarkers was observed under chronic inflammation, as compared to healthy animals. IC 50 values for PGE 2 inhibition showed a shift from 2840 ± 510 to 4000 ± 677 ng / ml ( mean ± SD ) , whilst IC 50 values for TXB 2 inhibition increased from 1180 ± 323 to 3360 ± 453 ng / ml in healthy and FCA-inoculated animals, respectively. Conclusions Our results show that chronic inflammation causes a significant change in the potency estimates for COX-inhibition. These findings illustrate the implications of pathophysiological processes on pharmacodynamics and consequently on the required exposure levels for achieving response during chronic treatment. Keywords NSAIDs Biomarkers PKPD modelling Naproxen Freund’s complete adjuvant 1 Introduction Blockade of cyclo-oxygenase (COX) activity by cyclo-oxygenase inhibitors results in direct suppression of the formation of pro-inflammatory mediators such as prostaglandins (PG) and thromboxanes (TXB) ( Vane, 1971 ). In fact, the selectivity of action with regard to COX isoforms has substantiated the extensive use of these drugs to achieve analgesia in acute and chronic inflammation. Yet, the screening and dose rationale for such compounds remain empirical, in that dose estimates are usually derived from behavioural measures in animal models for which understanding of the underlying inflammatory processes is rather limited. This approach presupposes that behavioural measures are predictive of the clinical dose, irrespective of the extent and duration of drug effect on biomarkers of inflammatory response. Hence, no effective evaluation has been performed to establish how biomarkers in these models of inflammation correlate with human inflammatory conditions. From a clinical pharmacology perspective, systematic ways to identify regimens that produce clinically relevant analgesia should be in place during pre-clinical drug development ( Derendorf et al., 2000 ). An important question that needs to be answered is how much and how long COX-2 and COX-1 should be inhibited to ensure an optimal risk-benefit ratio, allowing for a sustained analgesic response and an appropriate safety margin in the treatment of chronic inflammatory conditions. Unfortunately, at present the dose selection of COX-inhibitors is primarily based on evidence from clinical analgesia, disregarding whether maximum, long-lasting blockade of either enzyme systems is strictly required for response ( Huntjens et al., 2005 ). In fact, continuous COX-1 inhibition following prolonged administration of non-selective COX-inhibitors is known to induce gastrointestinal adverse effects, rather than analgesia. On the other hand, whilst selective sub-maximal COX-2 inhibition accounts for the anti-inflammatory and analgesic efficacy ( Vane, 1994 ), it is likely that prolonged suppression of COX-2 activity may underlie the cardiovascular events observed after chronic administration of selective COX-2 inhibitors. In that sense, the use of biomarkers may represent an important step in the development of anti-inflammatory compounds, enabling bridging of pharmacokinetics to the analgesic effect and providing a proxy for safety. Moreover, it would facilitate the extrapolation of pre-clinical findings in vivo , yielding earlier and more accurate predictions of the doses in phase I and II studies ( Huntjens et al., 2005 ). Given the mechanism of COX-inhibition and the nature of the inflammatory response, primary candidates for biomarkers of analgesia are PG and TXB. Using a model-based approach, it is possible to establish the relationship between these biological markers, pain measurement and safety. In this respect, we have recently shown the value of PKPD modelling to quantitatively characterise the inhibition of TXB 2 and PGE 2 by naproxen in vitro and in vivo in rats and healthy volunteers ( Huntjens et al., 2006 ). In the current investigation, we evaluate the impact of chronic inflammation on the effect of COX-inhibitors on PGE 2 and TXB 2 and its implications for the dosing regimen during chronic treatment. We attempt to address the aforementioned question using naproxen as a paradigm compound in the Freund’s complete adjuvant (FCA) model of chronic inflammation in rats. 2 Methods 2.1 Animals The experimental protocol was approved by the Ethical Committee on Animal Experimentation of the University of Leiden. Experiments were performed on male Sprague-Dawley (SD) rats (Charles River B.V., Maastricht, The Netherlands) weighing 322 ± 5 g ( Mean ± SEM, n = 34 ) . The animals, six per cage, were housed in standard plastic cages with a normal 12-h day/night schedule (lights on at 07.00 a.m.) and temperature of 21 °C. The animals had access to standard laboratory chow (RMH-TM; Hope Farms, Woerden, The Netherlands) and acidified water ad libitum . 2.2 Drug administration Naproxen (Sigma Aldrich BV, Zwijndrecht, The Netherlands) was dissolved in sterile 0.9% NaCl solution and administered intraperitoneally as a bolus at a dose of 10 mg/kg. 2.3 Experimental model of inflammation Freund’s complete adjuvant (FCA) ( 100 μ l of a 1 mg/kg M. tuberculosis (1 mg/kg), Sigma Aldrich BV, Zwijndrecht, The Netherlands) was injected intraplantarly with a 27-gauge needle into the left hind paw. The FCA injection produced an area of localised erythema and oedema that did not disturb grooming, sleep-wake rhythm or social interactions. Animals were divided into two groups for the evaluation of acute and chronic treatment effects. The first group of animals (acute treatment) was treated with a single i.p. dose of naproxen at post-inoculation day (PID) 7 and PID 21 (10 mg/kg), whilst the second group of animals (chronic treatment) received daily i.p. doses of naproxen (10 mg/kg) from PID 7 until PID 21. Naproxen was given in a dose volume of 1 ml/kg. Blood samples of 250 μ l were taken from the tail vein at pre-defined time points for the determination of drug, TXB 2 and PGE 2 concentrations. Blood was drawn prior to FCA administration and on PID 1, 7 or 8 and 14 or 15 for the determination of PGE 2 and TXB 2 concentrations under acute and chronic inflammatory conditions. Sampling from the tail vein was limited to seven blood samples per animal on the experiment day. Experiments were started in the morning (08.00 a.m.) or in the evening (06.00 p.m.) to account for eventual circadian variation in pharmacodynamics. Prior to drug administration, a baseline measurement was obtained to assess biomarker levels and drug concentrations at day 7 and day 21. Six post-dose samples were taken for the experiments starting in the morning, namely between 3 and 20 min (sample 1), 3 and 5 h (sample 2), 6 and 8 h (sample 3), 8 and 11 h (sample 4), 11 and 14 h (sample 5) and approximately 24 h (sample 6). Six post-dose samples were taken for the experiments starting in the evening, namely between 3 and 20 min (sample 1), 14 and 16 h (sample 2), 16 and 18 h (sample 3), 18 and 20 (sample 4), 21 and 23 h (sample 5) and approximately 24 h (sample 6). Blood samples were split into aliquots of 100 μ l (for PK and PGE 2 ) and 50 μ l (for TXB 2 ). Blood samples for PK were placed into heparinised tubes and centrifuged at 5000 rpm for 10 min. Plasma was stored at −20 °C until analysis. Blood samples for TXB 2 analysis were placed into tubes and allowed to clot for 1 h at 37 °C in a stirring water bath. Serum was collected after centrifugation and stored at −20 °C until analysis. Tubes for the analysis of PGE 2 were prepared by evaporating aspirin ( 10 μ g / ml in methanol) and heparin (10 I.U.) Blood samples were placed in tubes and 10 μ g / ml lipopolysaccharide (LPS) was added. Samples were incubated and stirred for 24 h at 37 °C in a water bath. Plasma was separated by centrifugation and stored at −20 °C until analysis. 2.4 Drug analysis Drug concentrations were analysed according to the method described by Satterwhite and Boudinot ( Satterwhite and Boudinot, 1988 ). Briefly, plasma samples were spiked with 50 μ l of internal standard (1000 mg/ml ketoprofen in methanol). The pH was adjusted by addition of 0.2 ml 1 M phosphate solution at pH 2. After extraction with 5 ml diethyl ether, the residue was dissolved in 100 μ l mobile phase, of which a volume of 50 μ l was injected into the HPLC system. The HPLC system consisted of a Waters 501 Solvent pump, a Waters 717plus autosampler (both Millipore-Waters, Milford, MA, USA), Superflow 757 Kratus UV absorbance detector (Shimadzu, Kyoto, Japan). Chromatography was performed on a C 18 3 μ m cartridge column (100 × 4.6 mm i.d., Chrompack, Bergen op Zoom, The Netherlands) equipped with a guard column. The mobile phase consisted of 0.02 M phosphate buffer (pH 7.0) and acetonitrile (82:18 v/v) with a flow rate of 1 ml/min. Detection was achieved by measuring the ultraviolet absorbance at a wavelength of 258 nm. Data acquisition and processing was performed using a Chromatopac C-R3A integrator (Shimadzu, Kyoto, Japan). The signal showed linearity over the range of 50–100,000 ng/ml. The within- and between-day coefficients of variation of the assay were 1.82% and 8.21%, respectively. 2.5 Analysis of TXB 2 and PGE 2 PGE 2 and TXB 2 were quantified by in vitro whole blood assay (WBA) using a validated enzyme immunoassay (Amersham Biosciences Europe GmbH, Freiburg, Germany). Briefly, samples were diluted in assay buffer (2–50 times for PGE 2 , 200-2000 times for TXB 2 ) and a 50 μ l aliquot was transferred into a coated well plate. After addition of 50 μ l antibody and 50 μ l peroxidase conjugate, samples were incubated for 1 h, washed four times and incubated for 15 min ( TXB 2 ) or 30 min ( PGE 2 ) , after which 150 μ l substrate was added. The enzyme reaction was halted by addition of 100 μ l 1 M sulphuric acid and optical density was measured in a plate reader at 450 nm. 2.6 Data analysis The pharmacokinetics and pharmacodynamics of naproxen were assessed by nonlinear mixed effects modelling, as implemented in NONMEM version V, level 1.1 (Globomax, Ellicott City, USA). Modelling of TXB 2 and PGE 2 inhibition was based on sparse pharmacokinetic and pharmacodynamic sampling in non-cannulated animals under acute and chronic inflammatory conditions. To ensure accurate estimation and identifiability of random effects on relevant model parameters, data published previously by Huntjens et al. on the pharmacokinetics and pharmacodynamics of naproxen in healthy animals were included in the analysis ( Huntjens et al., 2006 ). Final model parameters were estimated by the first order conditional estimation method with η – ε interaction (FOCE interaction). This approach allows the estimation of inter- and intraindividual variability in the model parameters. All fitting procedures were performed on a computer (AMD-Athlon XP-M 3000+) running under Windows XP with a FORTRAN compiler (Compaq Visual Fortran, version 6.1). An in-house interface for S-Plus 6.0 (Insightful Corp., Seattle, WA, USA) – NONMEM was used for data processing, management and graphical display. 2.7 Naproxen pharmacokinetic analysis A standard two-compartment pharmacokinetic model published by Huntjens et al. was used for the simultaneous analysis of the data presented in this paper ( Huntjens et al., 2006 ). Due to practical limitations, no plasma samples could be collected during the absorption phase after intraperitoneal injection. Given the exceptionally rapid absorption rate, this problem was circumvented by treating the absorption phase as intravenous data. The influence of chronic inflammation by FCA on naproxen disposition was tested by estimating different fixed and random parameters for healthy and disease conditions. Model selection and identification was based on the likelihood ratio test, parameter point estimates and their respective 95% confidence intervals, parameter correlations and goodness-of-fit plots. For the likelihood ratio test, the significance level for the inclusion of one parameter was set at p < 0.01 , which corresponds with a decrease of 6.63 points, in the minimum value of the objective function (MVOF) under the assumption that the difference in MVOF between two nested models is χ 2 distributed. The following goodness-of-fit plots were subjected to visual inspection to detect systemic deviations from the model fits: individual observed versus population or individual predicted values and weighted residuals versus time or population predicted values. Based on model selection criteria, a two-compartment model was appropriate to describe the pharmacokinetics of naproxen under healthy and disease conditions. The pharmacokinetic analysis was performed using the ADVAN6 routine in NONMEM. Model parameterisation included clearance (CL), inter-compartmental clearance (Q), and volumes of distribution in the central ( V 1 ) and peripheral compartment ( V 2 ). Variability in pharmacokinetic parameters was assumed to be log-normally distributed in the population. Therefore an exponential distribution model was used to account for inter-individual variability: (1) P i = θ i · exp ( η i ) where θ is the population estimate for parameter P , P i is the individual estimate and η i is the normally distributed interindividual random variable with mean zero and variance ω 2 . The coefficient of variation (CV %) of the structural model parameters is expressed as percentage of the root mean square of the interindividual variability term. Selection of an appropriate residual error model was based on inspection of the goodness-of-fit plots. A proportional error model was then proposed to describe the residual error in plasma drug concentrations: (2) C obs , ij = C pred , ij · ( 1 + ε ij , 1 ) where C obs , ij is the j th observed concentration in the i th individual, C pred , ij is the predicted concentration, and ε ij is the normally distributed residual random variable with mean zero and variance σ 2 . The residual error term contains all the error terms that cannot be explained by other fixed effects including experimental error (e.g. error in recording sampling times) and structural model misspecification. 2.8 Pharmacodynamic analysis As no specific covariate was found for pharmacokinetics, population parameter estimates were used as input for estimating plasma concentration at the sampling times for pharmacodynamics. PGE 2 and TXB 2 concentrations are used in this study as a measure of drug response. The sigmoid I max model was used to relate naproxen plasma concentration (C) to the drug response by the equation: (3) Effect = I 0 - ( I 0 - I max ) * ( C n / ( C n + IC 50 n ) ) where I - max represents the maximal inhibitory response to naproxen, I 0 the baseline production of PGE2 or TXB 2 and n the Hill coefficient. This equation is an adaptation from the E max model in order to obtain the absolute values for I 0 and I max for the direct calculation of maximal inhibition in percentages. For the final analysis, instead of estimating drug potency ( IC 50 ) , the model was re-parameterised in terms of IC 80 . The relationship between IC 50 and IC 80 was implemented in NONMEM by the following equation: (4) IC 80 = IC 50 · 4 n Exploratory graphical analysis showed a correlation between clock time and I max for PGE 2 inhibition, which was described by the following equation: (5) I max = θ i + θ j · clock time where I max is the maximal inhibitory response, θ i and θ j are intercept and slope of model parameter I max versus clock time relationship, respectively. 2.9 Covariates The role of potential covariate factors on model parameters was screened by univariate analysis. Covariates were then incorporated into the model by stepwise forward inclusion. A significance level of p < 0.01 was used for inclusion, which represented a drop of least 6.63 units in the objective function for each additional parameter A final evaluation of the statistical significance of all factors identified during the previous step was performed by subtracting each covariate individually (backward elimination). The final structural model (i.e., fixed effects model) included only those covariates whose subtraction resulted in a decrease of at least 10.83 units in the objective function ( p < 0.001 ) . 2.10 Visual predictive check The performance of the population PK and PKPD models were assessed by simulating 1000 data sets with the final model parameter estimates. The mean and the 95% confidence intervals were calculated for naproxen, PGE 2 and TXB 2 concentrations at the pre-defined sampling time points for the PK and PKPD models, respectively. 2.11 Statistical analysis Comparisons of the observed responses between pre- and post-FCA injection of PGE 2 and TXB 2 productions were made by a paired t -test. Statistical significance was set at p < 0.05 . 3 Results 3.1 Baseline biomarkers A summary of the systemic levels of biomarkers before and at various days after FCA inoculation is shown in Table 1 . No significant differences were observed in PGE 2 ( p = 0.66 ) and TXB 2 ( p = 0.32 ) levels before and after FCA injection. 3.2 Pharmacokinetics A two-compartment model with proportional error best described the pharmacokinetics of naproxen under disease conditions. The time course of observed and predicted naproxen concentrations is depicted in Fig. 1 . Additional modelling diagnostic plots reveal no bias or trends that might propagate into the PKPD analysis, for which predicted naproxen concentrations were used ( Fig. S1 , see the online version at doi:10.1016/j.ejpain.2009.05.017 ). The influence of potential disease processes on pharmacokinetics was investigated but no major effect was evident. Pharmacokinetic model parameter estimates are summarised in Table 2 . Clearance was found to be independent of the disease status. The only difference found between healthy and FCA-inoculated rats was an increase in the volume of distribution ( V 1 ) . Inter-individual variability for clearance could be estimated in healthy and disease conditions, but only in healthy rats for volume of distribution. 3.3 PKPD relationships Large variability was observed in the systemic levels of PGE 2 and TXB 2 . Nevertheless, maximum inhibition of PGE 2 and TXB 2 was immediate, with trough values being achieved at the first sampling time point after administration of naproxen. The PKPD relationship was best described using a sigmoid I max model. The population and individual predictions together with observed PGE 2 and TXB 2 inhibition are depicted in Fig. 2 . Diagnostic plots of model fitting are shown in Fig. S2 , see the online version at doi:10.1016/j.ejpain.2009.05.017 . Whilst baseline levels of biomarkers were found to be unaltered under disease conditions, drug effect on PGE 2 and TXB 2 was significantly different during chronic inflammation. For PGE 2 inhibition different estimates were obtained for potency, Hill coefficient and baseline PGE 2 in healthy and FCA-inoculated rats ( Table 3 ). For TXB 2 inhibition, potency, Hill coefficient and maximal inhibition differed between healthy and disease conditions ( Table 3 ). Inter-individual variability was found on IC 80 for PGE 2 inhibition and on IC 80 , I max and I 0 for TXB 2 inhibition. All parameters were accurately estimated as indicated by their CV%. IC 50 estimates for PGE 2 inhibition in healthy and FCA-inoculated rats were 2840 ± 510 and 4000 ± 677 ng / ml ( mean ± SD, p = 0.25 ) , respectively. IC 50 estimates for TXB 2 inhibition in healthy and diseased rats were 1180 ± 323 and 3360 ± 453 ng / ml ( p < 0.05 ) , respectively. The maximum inhibitory effects of naproxen resulted in more than 95 % suppression of PGE 2 and TXB 2 . In addition, exploratory graphical analysis showed a correlation between clock time and I max for PGE 2 inhibition, indicating the influence of circadian variation on this parameter. The incorporation of clock time as a function of I max significantly improved the fit ( p < 0.001 ) . A clock time cycle of 24 h was defined with zero being set at 08.00 a.m. In contrast, there was no correlation between clock time and baseline levels of PGE 2 . Other procedural and time-related covariates such as time after FCA injection (PID 7 versus PID 21) were not statistically significant. The implications of these factors are shown by simulations of the time course of inhibition of PGE 2 ( Fig. 3 ). Given the degree of COX-inhibition required for the analgesic and anti-inflammatory effects of COX-inhibitors, IC 80 values were also calculated. The IC 80 values for PGE 2 inhibition were 13400 and 12200 ng/ml for healthy and FCA-inoculated rats, respectively. IC 80 values for TXB 2 inhibition were 3450 and 4830 ng/ml for healthy and FCA injected rats, respectively. 4 Discussion and conclusions In the current study we have investigated the impact of chronic inflammatory conditions on the PKPD relationships of naproxen as a paradigm compound. The inhibition of PGE 2 and TXB 2 was selected as biomarker of drug effect in vivo . These endpoints are proposed as basis for the selection of clinical dosing regimen instead of the currently accepted behavioural measures of pain and inflammation, an approach which may enable accurate interspecies scaling of the anti-inflammatory response and provide a proxy for the reputed toxicity associated with the chronic use of COX-inhibitors. 4.1 Pharmacokinetics Thus far, few publications have discussed the pharmacology of analgesic drugs in animals and tried to correlate them to clinical findings ( Blackburn-Munro and Erichsen, 2005 ; Pullar and Palmer, 2003 ). Yet, none of these investigations have considered the impact of pharmacokinetics or disease processes on such a correlation. The present study was designed to assess the impact of inflammatory conditions on the pharmacological response to naproxen in animals and correlate our results with clinical findings taking into account and excluding confounding by pharmacokinetic factors. The differences observed in volume of distribution observed in FCA-inoculated rats may not be relevant for drug activity ( Josa et al., 2001 ). In fact, they may explained by the increase in blood vessel wall permeability due to inflammation. We have also found larger residual variability in the FCA treated rats (17% in healthy rats versus 36% in FCA rats), which could be due to the disease condition itself. Interestingly, thorough suppression of PGE 2 and TXB 2 was observed at concentrations higher than 50 μ g / ml . This exposure is equivalent to analgesic plasma levels in humans achieved after a 500 mg dose of naproxen ( Sevelius et al., 1980 ). Furthermore, our results clearly show that whilst systemic exposure is not altered upon chronic dosing with naproxen, pharmacodynamic changes do occur, which cannot be attributed to pharmacokinetic factors. Similarly, differences exist in the dosing regimen for analgesia in chronic pain conditions (e.g., osteoarthritis and rheumatoid arthritis) in humans, which are unrelated to the pharmacokinetics of naproxen ( Shi and Klotz, 2008 ). 4.2 Inflammatory markers and COX-activity under disease conditions The relevance of systemic markers of inflammation depends upon whether COX-activity in plasma can be linked to the target tissue and whether drug disposition does not differ significantly between tissues. In our experiments, local levels of inflammatory mediators have not been assessed. However, the absence of significant differences in the blood levels of PGE 2 and TXB 2 before and after FCA inoculation does not suggest that inflammation is not systemic ( Kumar and Roy, 2007 ). In this sense, we acknowledge that the use LPS to stimulate monocytes ex vivo may hamper the detection of changes in PGE 2 concentrations due to inflammation itself. In addition, slight differences in baseline values for the TXB 2 production were found between mean baseline values ( Table 1 ) and the I 0 value from the PKPD model ( Table 3 ). This can be explained by the way baseline values are computed in the model, which takes into account the inter-individual variability in I 0 . On the other hand, the rationale for the dose selection of an anti-inflammatory drug must account for the dynamics of the inflammatory process, which leads to up and down-regulation of various mediators at different time points and ultimately yields variation in drug response ( Egan et al., 2004 ). Such changes also influence pain processing and subsequently how behavioural measures vary over time. In this context, one may need to reconsider the emphasis on COX-2 as the critical factor for the modulation of hyperalgesia. The existence of COX-1 in tissues associated with nociceptive response and the fact that it can be induced means that a role for COX-1 is far from ruled out. The data available suggest a role for COX-1 in acute nociception, with inducible COX-2 mechanisms prevailing in chronic conditions. Such activity explains the efficacy of selective COX-1 inhibitors ( Gilroy et al., 1998 ). Another important consequence of the use of biomarkers for dose selection is the ability to assess the relative contribution of different targets to the overall analgesic effect, which could enhance the development of drug combinations. An example of this approach has recently been highlighted by Kortotkova et al. who have shown how glucocorticoids can lead to a significant down-regulation of mPGE synthase ( Korotkova et al., 2005 ), COX-1 and COX-2. Most importantly, the use of biomarkers circumvents the lack of sensitivity of behavioural measures of pain, which disregard differences in pharmacokinetics and pharmacodynamics or may not be relevant to the target mechanism ( Huntjens et al., 2009 ). 4.3 PKPD modelling and disease processes In pain research, considerable weight is given to extrapolation of pre-clinical data in models of acute pain without much attention to the impact of chronic diseases on drug effect ( Huang et al., 2007 ). In addition, results are often expressed in terms of effective dose per unit body weight (e.g., ED 50 / kg ), which makes scaling between species very empirical. In contrast, the use of biomarkers as basis for predicting the analgesic dose in humans may facilitate further understanding of adverse events associated with chronic use of NSAIDs, which cannot be fully characterised in pre-clinical experiments. Moreover, it prevents misinterpretation of results due to poorly designed experiments in vivo . Recently, Whiteside et al. have evaluated the predictive validity of pain models by comparing the pharmacokinetic–pharmacodynamic relationship for pain drugs in rats and humans. A discrepancy range varying from 4- to 37-fold was observed between species in inflammatory pain and neuropathic pain, respectively. However, their findings do not explain how differences in pharmacological properties determine downstream response in either species. The authors also fail to explain the variation in dose requirements for efficacy in chronic pain conditions ( Whiteside et al., 2004 ). We anticipate that the characterisation pharmacokinetic–pharmacodynamic relationships for markers of inflammatory response may contribute to overcoming the empiricism in dosing rationale for pain treatment. In particular, PKPD relationships can be useful if parameterisation accounts for the nature of the ligand–receptor interaction, for instance by assessing IC 80 instead of IC 50 for enzyme inhibitors. In fact, the relevance of the hWBA in vitro IC 80 estimates from the human WBA to predict the analgesic dose in patients has been highlighted previously ( Huntjens et al., 2005 ). The advantages of such an approach are exemplified by our findings with naproxen. On a dose basis, empirical comparison with other COX-inhibitors show large difference between estimates from animal data and clinically prescribed doses (see Table 4 ). Such discrepancies are difficult to interpret without further evidence of how each drug actually interacts with the target and to what extent the downstream cascade is inhibited. The importance of such an evaluation has been illustrated by Capone et al. who compared the therapeutic concentrations of various non-selective and selective COX-2 inhibitors with the concentrations inhibiting by 80% COX-1 activity and 80% COX-2 activity ( Capone et al., 2007 ). Interestingly, successful application of a pharmacokinetic–pharmacodynamic approach for the determination of dosing regimens has been reported in veterinary medicine. Dose selection of nimesulide based on PKPD relationships allowed appropriate control of pain and inflammation, but could not fully explain their findings since data on biomarkers were not collected during the experiment ( Toutain et al., 2001 ). Unfortunately, there is resistance for a similar reasoning in drug development and clinical medicine. The impact of disease status on drug effect is revealed by the differences in parameter estimates after acute and chronic inflammation. The potency and Hill coefficient for the inhibition of PGE 2 and TXB 2 , as well as baseline TXB 2 values were altered by chronic inflammatory conditions. Yet, the selectivity based on the log IC 80 COX-2/COX-1 ratio remained unaltered. IC 80 values for PGE 2 inhibition showed less than 2-fold difference between healthy and chronic inflamed rats (8.5 versus 15.8 μ g / ml , respectively). IC 80 values for TXB 2 inhibition did not vary significantly between healthy and chronic inflamed rats (3.2 versus 5.5 μ g / ml , respectively). These differences cannot be assigned to pharmacokinetic factors and are likely to be driven by the pathophysiological mechanisms described above. 4.4 Potential limitations Unfortunately, limited data exists for chronic inflammation in the FCA model to allow further generalisation of these findings ( Kumar and Roy, 2007 ). Reduced potency in the adjuvant arthritis model was also observed for glucosamine ( Hua et al., 2005 ). Our experiment does not permit complete exclusion of a methodological artefact of the FCA model itself as cause of the differences between acute and chronic inflammation, but we do not expect this to be the case. More data is required to discriminate how COX-inhibitors affect inflammatory markers in different animal models of pain. In conclusion, our results show the value of biomarkers instead of behavioural measures of pain as basis for the prediction of analgesic dose range in humans. These findings also explain why different dosing regimens of COX-inhibitors may be required for acute and chronic pain indications. A mechanism-based description of drug-disease interaction is essential to ensure appropriate evaluation of drug safety and efficacy in chronic inflammatory conditions. Given the rather unscientific way in which clinical findings and the underlying risk:benefit ratio for COX-2 inhibitors has been assessed, it is clear that without a radical change in the rationale for dose selection, patients may be prevented from getting access to valuable treatment options. Acknowledgements We gratefully acknowledge the technical assistance of Margret Blom-Roosemalen. The authors are also grateful to Dorien Groenendaal, Lia Liefaard and Kasper Rouschop for their generous cooperation throughout the in vivo studies. The work presented in this paper was supported by a fellowship from GlaxoSmithKline, Harlow, United Kingdom. References Blackburn-Munro and Erichsen, 2005 G. Blackburn-Munro H.K. Erichsen Antiepileptics and the treatment of neuropathic pain: evidence from animal models Curr Pharm Des 11 2005 2961 2976 Capone et al., 2007 M.L. Capone S. Tacconelli L. Di Francesco A. Sacchetti M.G. Sciulli P. Patrignani Pharmacodynamic of cyclooxygenase inhibitors in humans Prostag Other Lipid Mediat 82 2007 85 94 Chan et al., 1999 C.C. Chan S. Boyce C. Brideau S. Charleson W. Cromlish D. Ethier Rofecoxib [Vioxx, MK-0966; 4-(4′-methylsulfonylphenyl)-3-phenyl-2-(5H)-furanone]: a potent and orally active cyclooxygenase-2 inhibitor. Pharmacological and biochemical profiles J Pharmacol Exp Ther 290 1999 551 560 Derendorf et al., 2000 H. Derendorf L.J. Lesko P. Chaikin W.A. Colburn P. Lee R. Miller Pharmacokinetic/pharmacodynamic modeling in drug research and development J Clin Pharmacol 40 2000 1399 1418 Egan et al., 2004 C.G. Egan J.C. Lockhart W.R. Ferrell Pathophysiology of vascular dysfunction in a rat model of chronic joint inflammation J Physiol 557 2004 635 643 Esser et al., 2005 R. Esser C. Berry Z. Du J. Dawson A. Fox R.A. Fujimoto Preclinical pharmacology of lumiracoxib: a novel selective inhibitor of cyclooxygenase-2 Br J Pharmacol 144 2005 538 550 Gierse et al., 2005 J.K. Gierse Y. Zhang W.F. Hood M.C. Walker J.S. Trigg T.J. Maziasz Valdecoxib: assessment of cyclooxygenase-2 potency and selectivity J Pharmacol Exp Ther 312 2005 1206 1212 Gilroy et al., 1998 D.W. Gilroy A. Tomlinson D.A. Willoughby Differential effects of inhibition of isoforms of cyclooxygenase (COX-1, COX-2) in chronic inflammation Inflamm Res 47 1998 79 85 Hua et al., 2005 J. Hua S. Suguro S. Hirano K. Sakamoto I. Nagaoka Preventive actions of a high dose of glucosamine on adjuvant arthritis in rats Inflamm Res 54 2005 127 132 Huang et al., 2007 C. Huang M. Zheng Z. Yang A.D. Rodrigues P. Marathe Projection of exposure and efficacious dose prior to first-in-human studies: how successful have we been? Pharm Res 2007 Huntjens et al., 2005 D.R. Huntjens M. Danhof O.E. Della Pasqua Pharmacokinetic–pharmacodynamic correlations and biomarkers in the development of COX-2 inhibitors Rheumatology (Oxford) 44 2005 846 859 Huntjens et al., 2006 D.R. Huntjens D.J. Spalding M. Danhof O.E. Della Pasqua Correlation between in vitro and in vivo concentration–effect relationships of naproxen in rats and healthy volunteers Br J Pharmacol 148 2006 396 404 Huntjens et al., 2009 D.R. Huntjens D.J. Spalding M. Danhof O.E. Della Pasqua Differences in the sensitivity of behavioural measures of pain to the selectivity of cyclo-oxygenase inhibitors Eur J Pain 13 2009 448 457 Josa et al., 2001 M. Josa J.P. Urizar J. Rapado C. Dios-Vieitez G. Castaneda-Hernandez F. Flores-Murrieta Pharmacokinetic/pharmacodynamic modeling of antipyretic and anti-inflammatory effects of naproxen in the rat J Pharmacol Exp Ther 297 2001 198 205 Korotkova et al., 2005 M. Korotkova M. Westman K.R. Gheorghe K.E. af C. Trollmo A.K. Ulfgren Effects of antirheumatic treatments on the prostaglandin E2 biosynthetic pathway Arthritis Rheum 52 2005 3439 3447 Kumar and Roy, 2007 V.L. Kumar S. Roy Calotropis procera latex extract affords protection against inflammation and oxidative stress in Freund’s complete adjuvant-induced monoarthritis in rats Mediators Inflamm 2007 2007 47523 Pullar and Palmer, 2003 S. Pullar A.M. Palmer Pharmacotherapy for neuropathic pain: progress and prospects Drug News Perspect 16 2003 622 630 Satterwhite and Boudinot, 1988 J.H. Satterwhite F.D. Boudinot High-performance liquid chromatographic determination of ketoprofen and naproxen in rat plasma J Chromatogr 431 1988 444 449 Sevelius et al., 1980 H. Sevelius R. Runkel E. Segre S.S. Bloomfield Bioavailability of naproxen sodium and its relationship to clinical analgesic effects Br J Clin Pharmacol 10 1980 259 263 Shi and Klotz, 2008 S. Shi U. Klotz Clinical use and pharmacological properties of selective COX-2 inhibitors Eur J Clin Pharmacol 64 2008 233 252 Toutain et al., 2001 P.L. Toutain C.C. Cester T. Haak V. Laroute A pharmacokinetic/pharmacodynamic approach vs. a dose titration for the determination of a dosage regimen: the case of nimesulide, a Cox-2 selective nonsteroidal anti-inflammatory drug in the dog J Vet Pharmacol Ther 24 2001 43 55 Vane, 1971 J.R. Vane Inhibition of prostaglandin synthesis as a mechanism of action for aspirin-like drugs Nat New Biol 231 1971 232 235 Vane, 1994 J. Vane Towards a better aspirin Nature 367 1994 215 216 Whiteside et al., 2004 G.T. Whiteside J. Harrison J. Boulet L. Mark M. Pearson S. Gottshall Pharmacological characterisation of a rat model of incisional pain Br J Pharmacol 141 2004 85 91
更多查看译文
关键词
NSAIDs,Biomarkers,PKPD modelling,Naproxen,Freund’s complete adjuvant
AI 理解论文
溯源树
样例
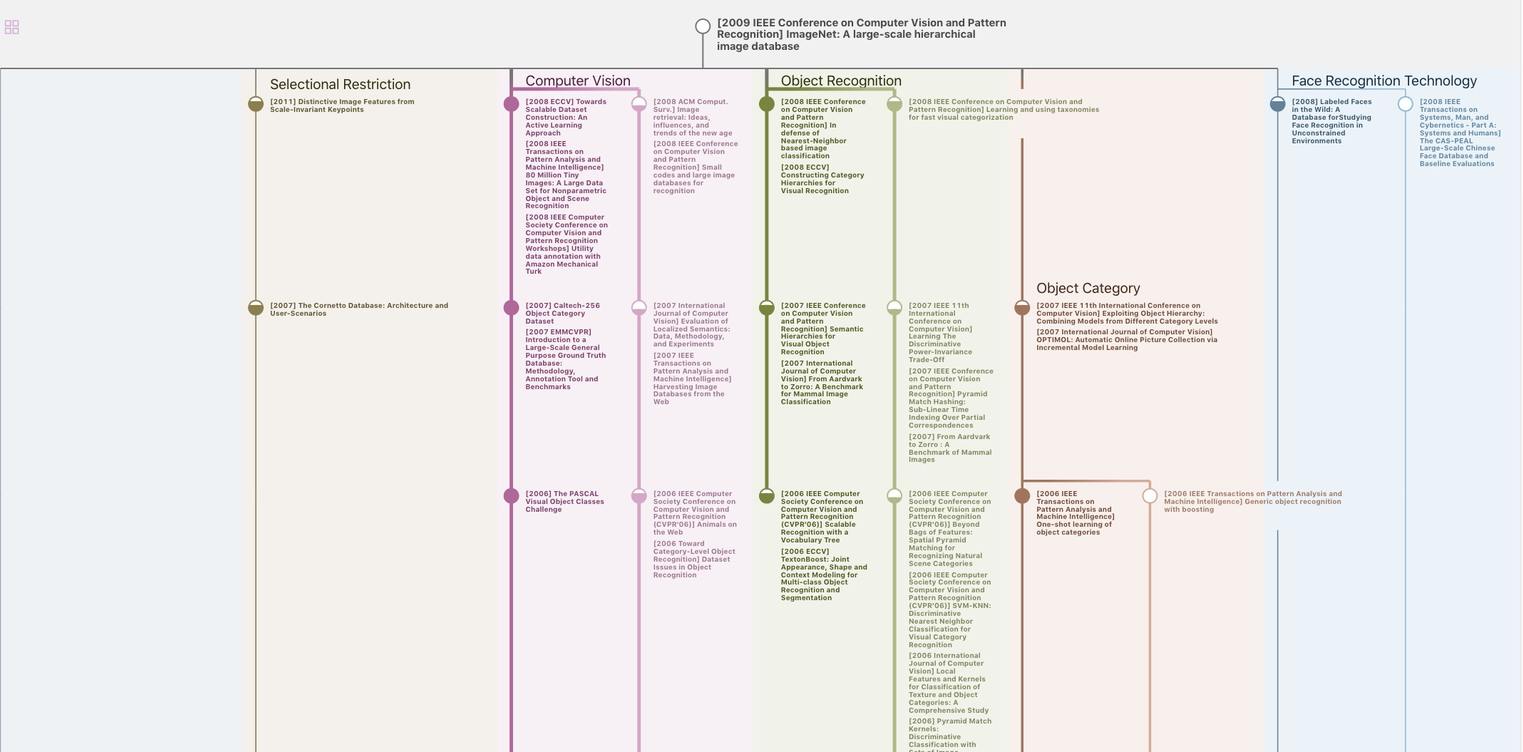
生成溯源树,研究论文发展脉络
Chat Paper
正在生成论文摘要