PHD-Store: an Adaptive SPARQL Engine with Dynamic Partitioning for Distributed RDF Repositories.
CoRR(2014)
摘要
Many repositories utilize the versatile RDF model to publish data. Repositories are typically distributed and geographically remote, but data are interconnected (e.g., the Semantic Web) and queried globally by a language such as SPARQL. Due to the network cost and the nature of the queries, the execution time can be prohibitively high. Current solutions attempt to minimize the network cost by redistributing all data in a preprocessing phase, but here are two drawbacks: (i) redistribution is based on heuristics that may not benefit many of the future queries; and (ii) the preprocessing phase is very expensive even for moderate size datasets. In this paper we propose PHD-Store, a SPARQL engine for distributed RDF repositories. Our system does not assume any particular initial data placement and does not require prepartitioning; hence, it minimizes the startup cost. Initially, PHD-Store answers queries using a potentially slow distributed semi-join algorithm, but adapts dynamically to the query load by incrementally redistributing frequently accessed data. Redistribution is done in a way that future queries can benefit from fast hash-based parallel execution. Our experiments with synthetic and real data verify that PHD-Store scales to very large datasets; many repositories; converges to comparable or better quality of partitioning than existing methods; and executes large query loads 1 to 2 orders of magnitude faster than our competitors.
更多查看译文
AI 理解论文
溯源树
样例
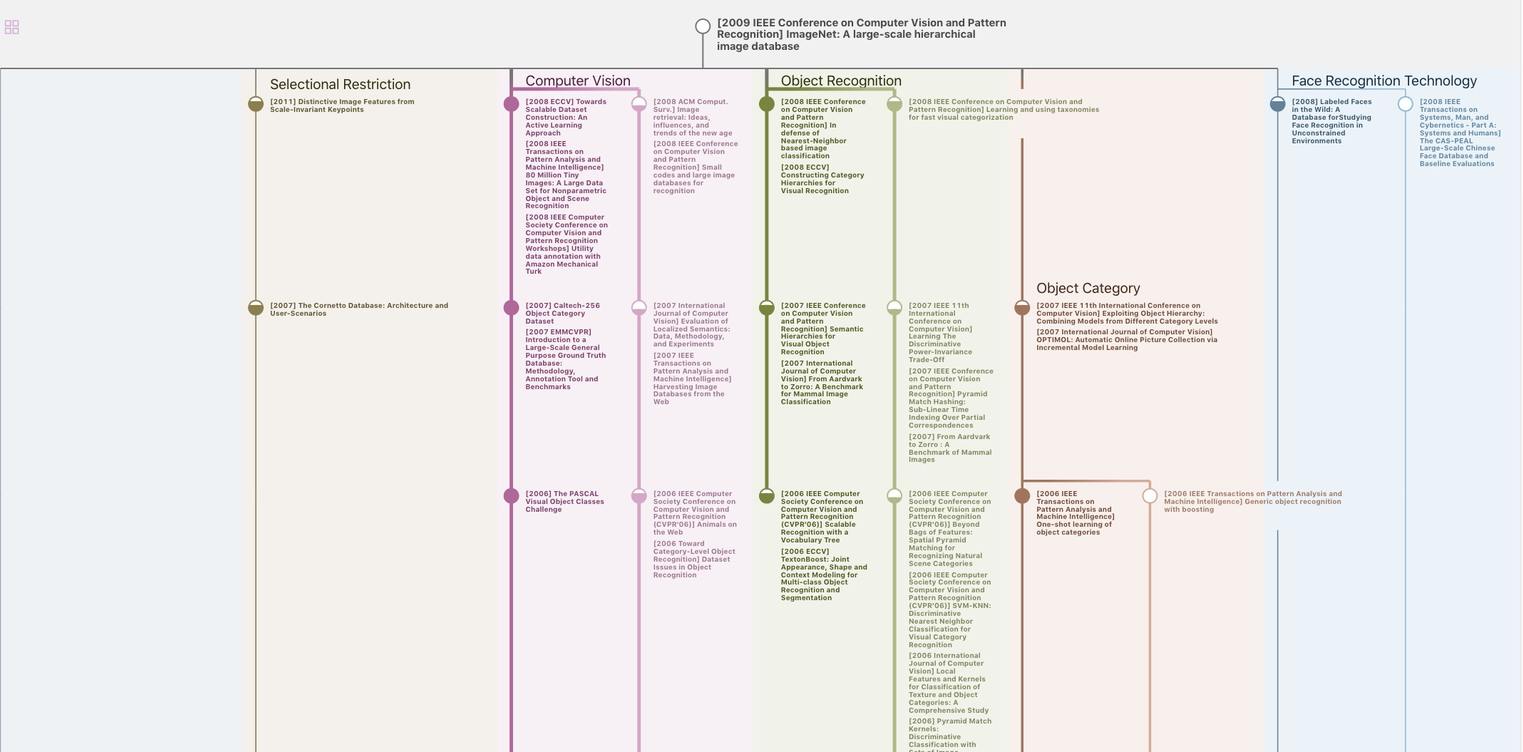
生成溯源树,研究论文发展脉络
Chat Paper
正在生成论文摘要