Person Re-identification by Efficient Impostor-Based Metric Learning
2012 IEEE NINTH INTERNATIONAL CONFERENCE ON ADVANCED VIDEO AND SIGNAL-BASED SURVEILLANCE (AVSS)(2012)
摘要
Recognizing persons over a system of disjunct cameras is a hard task for human operators and even harder for automated systems. In particular, realistic setups show difficulties such as different camera angles or different camera properties. Additionally, also the appearance of exactly the same person can change dramatically due to different views (e.g., frontal/back) of carried objects. In this paper, we mainly address the first problem by learning the transition from one camera to the other. This is realized by learning a Mahalanobis metric using pairs of labeled samples from different cameras. Building on the ideas of Large Margin Nearest Neighbor classification, we obtain a more efficient solution which additionally provides much better generalization properties. To demonstrate these benefits, we run experiments on three different publicly available datasets, showing state-of-the-art or even better results, however, on much lower computational efforts. This is in particular interesting since we use quite simple color and texture features, whereas other approaches build on rather complex image descriptions!
更多查看译文
关键词
different camera,different camera angle,different camera property,different view,disjunct camera,better result,Large Margin,Nearest Neighbor classification,Recognizing person,automated system,Efficient Impostor-Based Metric Learning,Person Re-identification
AI 理解论文
溯源树
样例
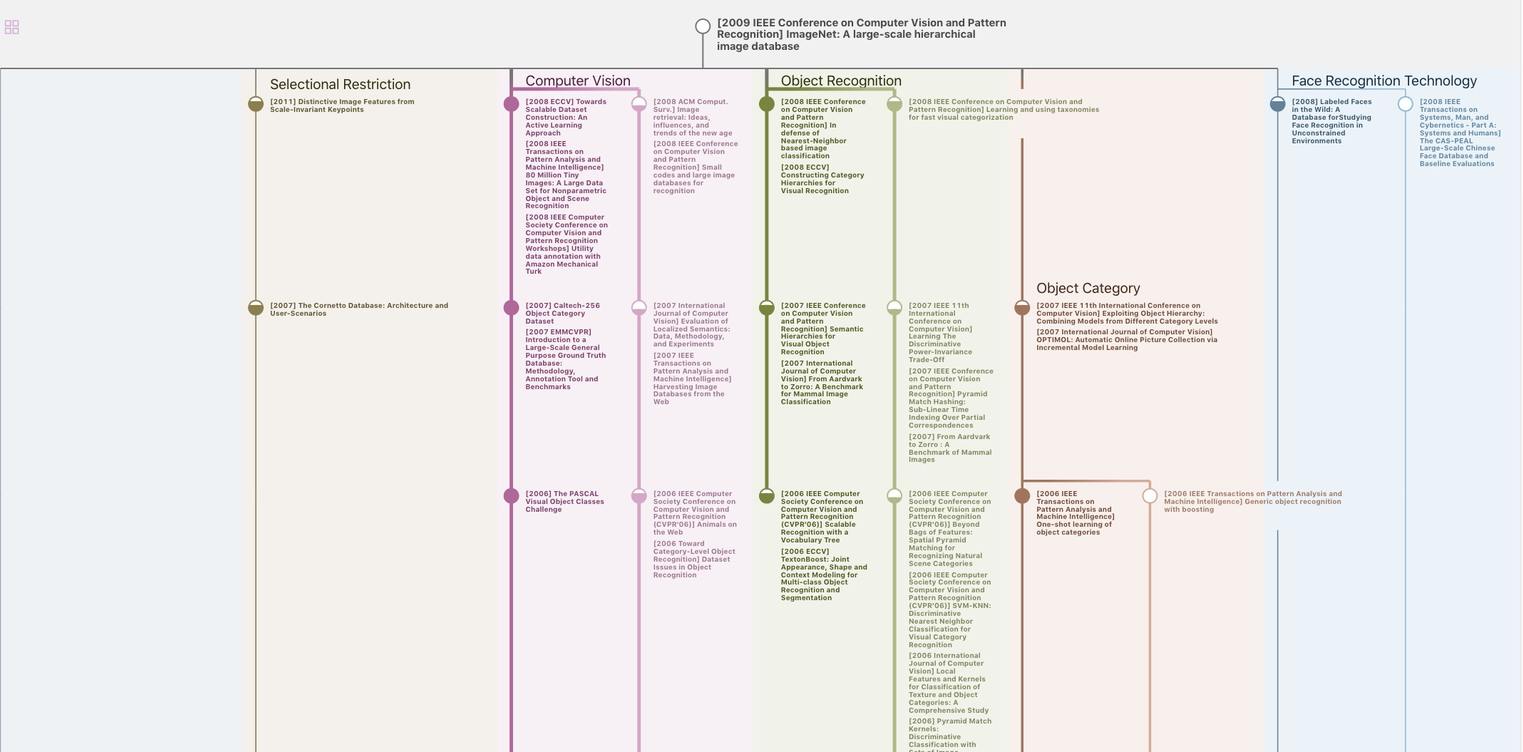
生成溯源树,研究论文发展脉络
Chat Paper
正在生成论文摘要