Parameter Estimation for Asymptotic Regression Model by Particle Swarm Optimization
Conference Companion on Genetic and Evolutionary Computation(2009)
摘要
Asymptotic regression model (ARM) has been widely used in the field of agriculture, biology and engineering, especially in agriculture. Parameter estimation for ARM is a significant, challenging and difficult issue. The modern heuristic algorithm has been proved to be a highly effective and successful technique in parameter estimation of nonlinear models. As a novel evolutionary computation paradigm based on social behavior of bird flocking or fish schooling, particle swarm optimization (PSO) has shown outstanding performance in many real-world applications, for it is conceptually simple and practically easy to be implemented. In the present work, parameters of ARM are estimated on the basis of PSO for the first time. Firstly, PSO is compared with evolutionary algorithm (EA) on seven groups of actual data; PSO, while using less number of function evaluations, can find a parameter set as well as EA. Secondly, we estimate one-dimensional, two-dimensional and three-dimensional parameter by fixing two, one and zero of all parameters of ARM, respectively. Finally, how sampling range and data with Gaussian noise influence on the performance of PSO is considered. Experimental results show that PSO is a stable, reliable and effective method in parameter estimation for ARM and it's robust to noise.
更多查看译文
关键词
particle swarm optimization,parameter estimation,asymptotic regression model,nonlinear system
AI 理解论文
溯源树
样例
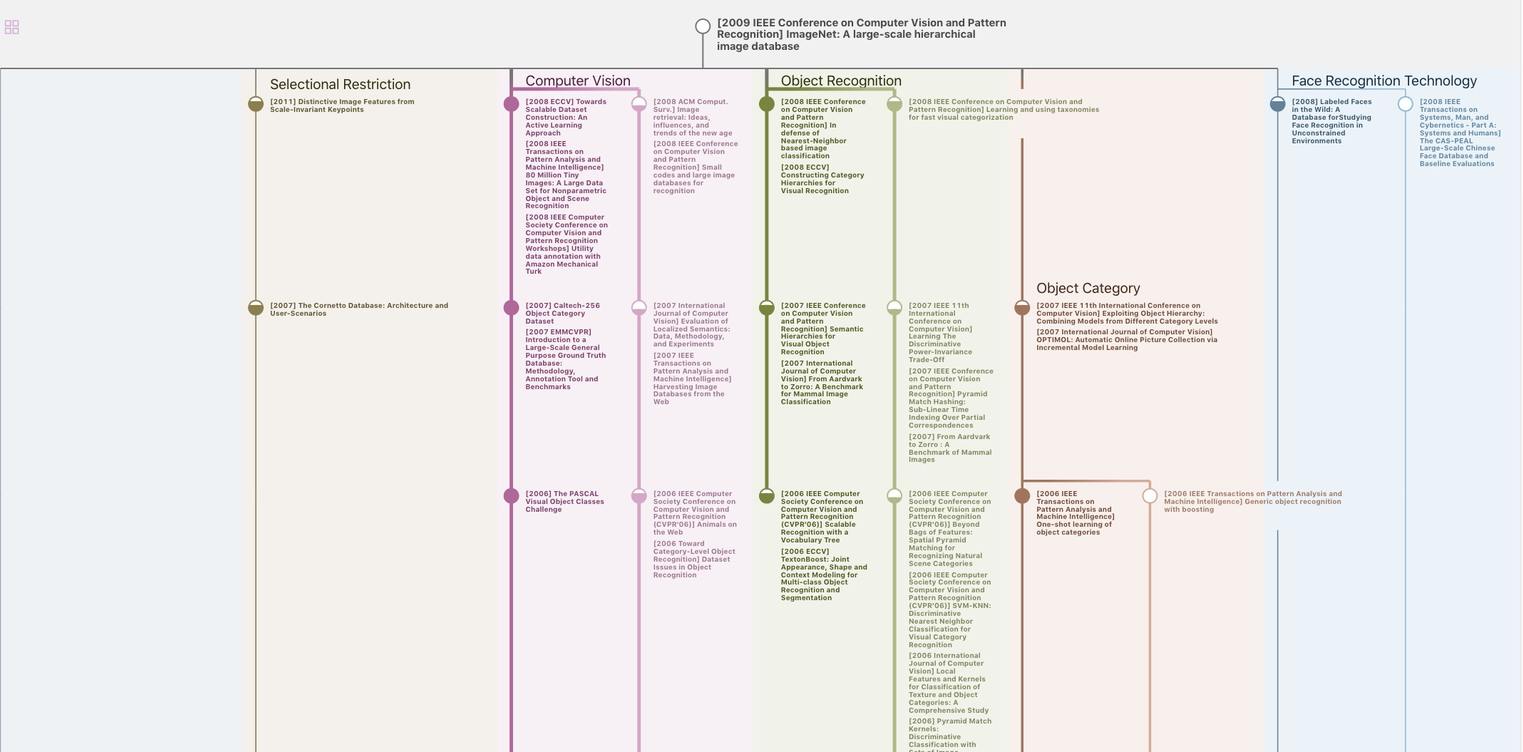
生成溯源树,研究论文发展脉络
Chat Paper
正在生成论文摘要