The Proposition Bank: an Annotated Corpus of Semantic Roles
Computational Linguistics(2005)CCF BSCI 3区SCI 4区
Univ Penn | university of pennsylvania
Abstract
The Proposition Bank project takes a practical approach to semantic representation, adding a layer of predicate-argument information, or semantic role labels, to the syntactic structures of the Penn Treebank. The resulting resource can be thought of as shallow, in that it does not represent coreference, quantification, and many other higher-order phenomena, but also broad, in that it covers every instance of every verb in the corpus and allows representative statistics to be calculated. We discuss the criteria used to define the sets of semantic roles used in the annotation process and to analyze the frequency of syntactic/semantic alternations in the corpus. We describe an automatic system for semantic role tagging trained on the corpus and discuss the effect on its performance of various types of information, including a comparison of full syntactic parsing with a flat representation and the contribution of the empty “trace” categories of the treebank.
MoreTranslated text
PDF
View via Publisher
AI Read Science
AI Summary
AI Summary is the key point extracted automatically understanding the full text of the paper, including the background, methods, results, conclusions, icons and other key content, so that you can get the outline of the paper at a glance.
Example
Background
Key content
Introduction
Methods
Results
Related work
Fund
Key content
- Pretraining has recently greatly promoted the development of natural language processing (NLP)
- We show that M6 outperforms the baselines in multimodal downstream tasks, and the large M6 with 10 parameters can reach a better performance
- We propose a method called M6 that is able to process information of multiple modalities and perform both single-modal and cross-modal understanding and generation
- The model is scaled to large model with 10 billion parameters with sophisticated deployment, and the 10 -parameter M6-large is the largest pretrained model in Chinese
- Experimental results show that our proposed M6 outperforms the baseline in a number of downstream tasks concerning both single modality and multiple modalities We will continue the pretraining of extremely large models by increasing data to explore the limit of its performance
Try using models to generate summary,it takes about 60s
Must-Reading Tree
Example
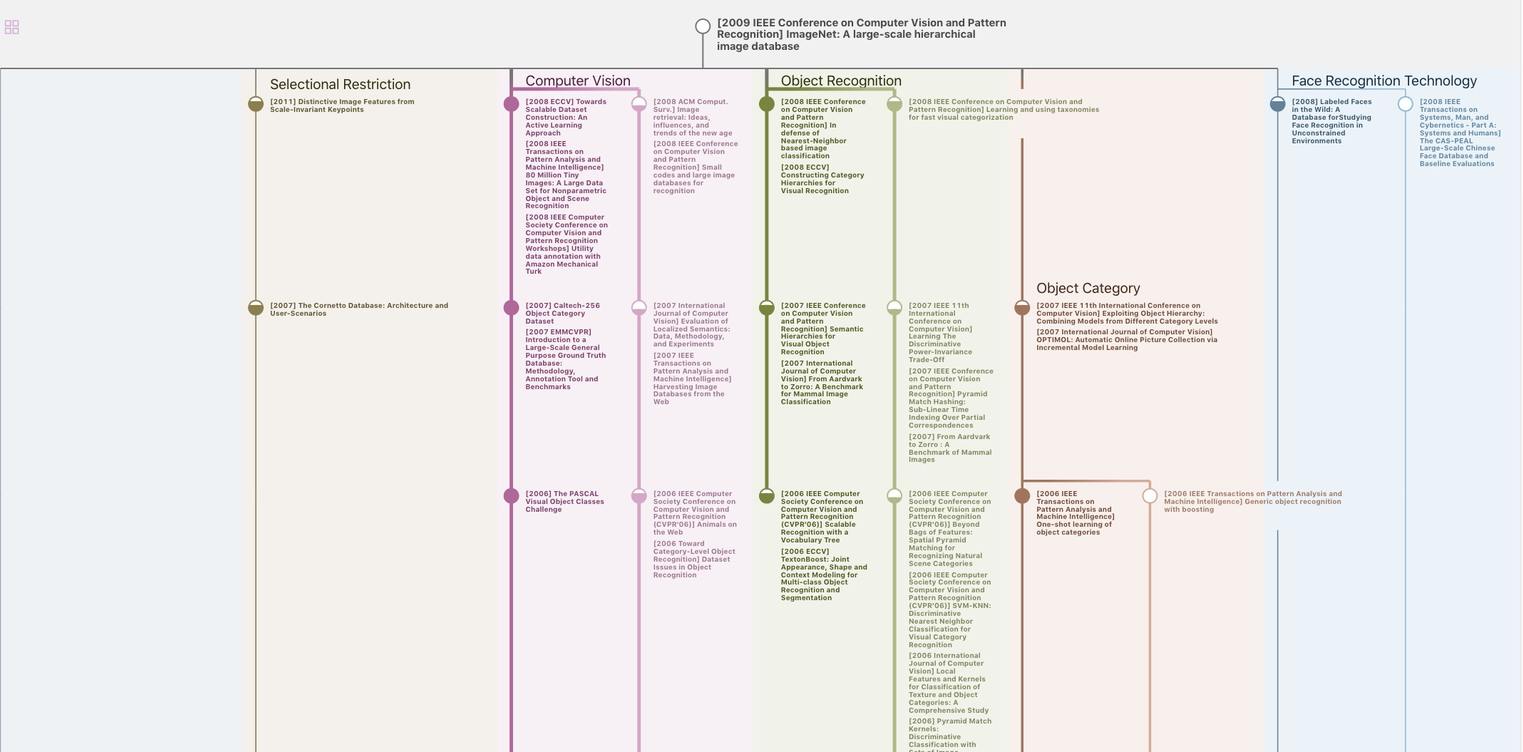
Generate MRT to find the research sequence of this paper
Related Papers
2021
被引用302 | 浏览
Data Disclaimer
The page data are from open Internet sources, cooperative publishers and automatic analysis results through AI technology. We do not make any commitments and guarantees for the validity, accuracy, correctness, reliability, completeness and timeliness of the page data. If you have any questions, please contact us by email: report@aminer.cn
Chat Paper
去 AI 文献库 对话