Network Control by Bayesian Broadcast
IEEE transactions on information theory(1987)
MIT
Abstract
A transmission control strategy is described for slotted-ALOHA-type broadcast channels with ternary feedback. At each time slot, each station estimates the probability that n stations are ready to transmit a packet for eachn, using Bayes' rule and the observed history of collisions, successful transmissions, and holes (empty slots). A station transmits a packet in a probabilistic manner based on these estimates. This strategy is called Bayesian broadcast. An elegant and very practical strategy--pseudo-Bayesian broadcast--is then derived by approximating the probability estimates with a Poisson distribution with meannuand further simplifying. Each station keeps a copy ofnu, transmits a packet with probability1/nu, and then updatesnuin two steps: For collisions, incrementnuby(e-2)^{-l}=1.39221 cdots. For successes and holes, decrementnuby1. Setnutomax (nu + hat{lambda}, 1), wherehat{lambda}is an estimate of the arrival ratelambdaof new packets into the system. Simulation results are presented showing that pseudo-Bayesian broadcast performs well in practice, and methods that can be used to prove that certain versions of pseudo-Bayesian broadcast are stable forlambda < e^{-1}are discussed.
MoreTranslated text
Key words
pseudo-Bayesian broadcast,Bayesian broadcast,slotted-ALOHA-type broadcast channel,arrival ratelambdaof new packet,n station,practical strategy,transmission control strategy,probability estimate,Poisson distribution,certain version,bayesian broadcast,network control
求助PDF
上传PDF
View via Publisher
AI Read Science
AI Summary
AI Summary is the key point extracted automatically understanding the full text of the paper, including the background, methods, results, conclusions, icons and other key content, so that you can get the outline of the paper at a glance.
Example
Background
Key content
Introduction
Methods
Results
Related work
Fund
Key content
- Pretraining has recently greatly promoted the development of natural language processing (NLP)
- We show that M6 outperforms the baselines in multimodal downstream tasks, and the large M6 with 10 parameters can reach a better performance
- We propose a method called M6 that is able to process information of multiple modalities and perform both single-modal and cross-modal understanding and generation
- The model is scaled to large model with 10 billion parameters with sophisticated deployment, and the 10 -parameter M6-large is the largest pretrained model in Chinese
- Experimental results show that our proposed M6 outperforms the baseline in a number of downstream tasks concerning both single modality and multiple modalities We will continue the pretraining of extremely large models by increasing data to explore the limit of its performance
Upload PDF to Generate Summary
Must-Reading Tree
Example
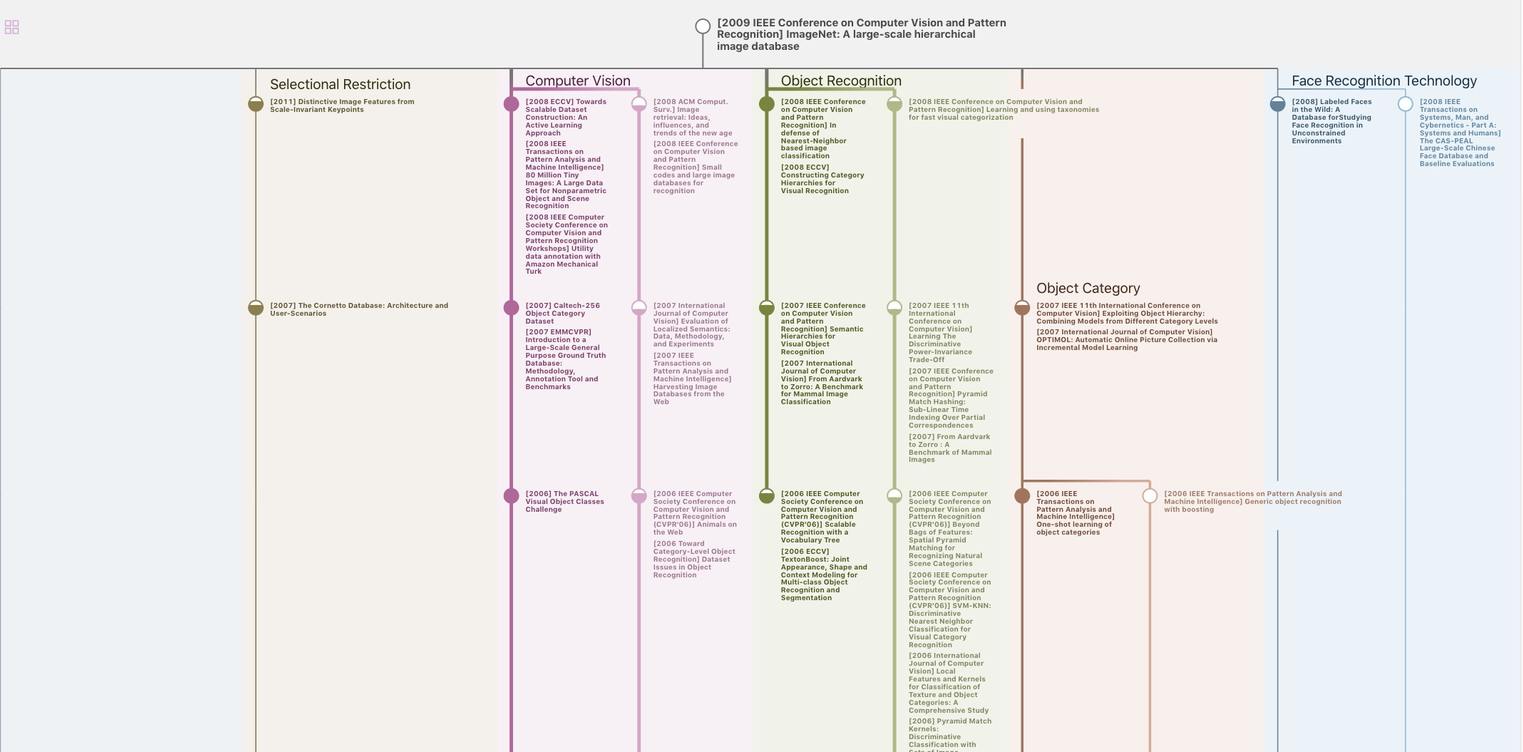
Generate MRT to find the research sequence of this paper
Related Papers
Data Disclaimer
The page data are from open Internet sources, cooperative publishers and automatic analysis results through AI technology. We do not make any commitments and guarantees for the validity, accuracy, correctness, reliability, completeness and timeliness of the page data. If you have any questions, please contact us by email: report@aminer.cn
Chat Paper