基本信息
views: 177
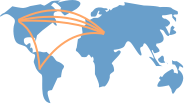
Bio
Research Overview
During the course of development, human infants gather information about the external world without the benefit of an extensive base of knowledge that adults automatically bring to bear on perceptual, motor, cognitive, and language tasks. What mechanisms allow infants to acquire this initial level of information and how does that information guide subsequent learning? Clearly, most learning that occurs in infancy, and a substantial amount of learning in adulthood, is performed without instruction—it is implicit and based on an analysis of the distributional properties of environmental stimulation.
For over two decades, my research has been directed at exploring and understanding these implicit learning mechanisms, which are typically referred to as “statistical learning”. Although initially studied in the task of word segmentation from fluent speech, statistical learning has been extended to other domains, such as musical tones, phonetic categories, sequences of visual shapes, sequences of motor responses, and combinations of objects (or object parts) in complex visual scenes. An important goal of these studies is to reveal the computational constraints that enable statistical learning to be tractable given the complexity of the input and the infinite number of statistical computations that are possible over any set of inputs. Initial computational models of statistical learning focused on bi-gram statistics and conditional probabilities, but more recent work has broadened to include Bayesian ideal learning models. Empirical studies of statistical learning have also evolved to explore order effects in learning multiple structures and to understand how statistical patterns trigger the formation of categories.
During the course of development, human infants gather information about the external world without the benefit of an extensive base of knowledge that adults automatically bring to bear on perceptual, motor, cognitive, and language tasks. What mechanisms allow infants to acquire this initial level of information and how does that information guide subsequent learning? Clearly, most learning that occurs in infancy, and a substantial amount of learning in adulthood, is performed without instruction—it is implicit and based on an analysis of the distributional properties of environmental stimulation.
For over two decades, my research has been directed at exploring and understanding these implicit learning mechanisms, which are typically referred to as “statistical learning”. Although initially studied in the task of word segmentation from fluent speech, statistical learning has been extended to other domains, such as musical tones, phonetic categories, sequences of visual shapes, sequences of motor responses, and combinations of objects (or object parts) in complex visual scenes. An important goal of these studies is to reveal the computational constraints that enable statistical learning to be tractable given the complexity of the input and the infinite number of statistical computations that are possible over any set of inputs. Initial computational models of statistical learning focused on bi-gram statistics and conditional probabilities, but more recent work has broadened to include Bayesian ideal learning models. Empirical studies of statistical learning have also evolved to explore order effects in learning multiple structures and to understand how statistical patterns trigger the formation of categories.
Research Interests
Papers共 338 篇Author StatisticsCo-AuthorSimilar Experts
By YearBy Citation主题筛选期刊级别筛选合作者筛选合作机构筛选
时间
引用量
主题
期刊级别
合作者
合作机构
BRAIN RESEARCH (2024)
crossref(2024)
Trends in Neurosciences (2024)
Trends in Cognitive Sciencesno. 8 (2023): 692-693
openalex(2023)
Cognition (2023): 105601-105601
Neuropsychology reviewno. 3 (2023): 860-881
Benjamin D. Zinszer,Laura Pirazzoli, Charles A. Nelson,Lauren Emberson,Richard Aslin,Vikranth R Bejjanki
crossref(2023)
openalex(2023)
Load More
Author Statistics
#Papers: 338
#Citation: 33259
H-Index: 77
G-Index: 181
Sociability: 6
Diversity: 3
Activity: 35
Co-Author
Co-Institution
D-Core
- 合作者
- 学生
- 导师
Data Disclaimer
The page data are from open Internet sources, cooperative publishers and automatic analysis results through AI technology. We do not make any commitments and guarantees for the validity, accuracy, correctness, reliability, completeness and timeliness of the page data. If you have any questions, please contact us by email: report@aminer.cn