基本信息
浏览量:112
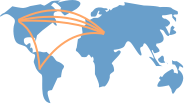
个人简介
Our research areas are theoretical neuroscience and neuroscience-guided machine learning. We seek to uncover the algorithms of the brain and their implementation at the network and cellular levels. But, how can we infer what the brain computes from the large datasets of modern neuroscience? For that, we need a new “algorithmic” theory that bridges computation and its biological realization.
We have been working on an algorithmic theory for learning in the sensory domain. Sensory cortices learn from stimuli to build behaviorally relevant representations, with little or no supervision. Our theory starts by posing computational goals of unsupervised learning as mathematical optimization problems. Then, from these problems, we systematically derive algorithms and neural circuit implementations of these algorithms, linking computation to biological realization. Our approach answers how efficient self-organization for learning happens with local synaptic plasticity, provides new circuit motifs and mechanisms that could be in use in the brain, predicts computations performed in specific circuits, and provides computational interpretations of salient features of such circuits.
We have been working on an algorithmic theory for learning in the sensory domain. Sensory cortices learn from stimuli to build behaviorally relevant representations, with little or no supervision. Our theory starts by posing computational goals of unsupervised learning as mathematical optimization problems. Then, from these problems, we systematically derive algorithms and neural circuit implementations of these algorithms, linking computation to biological realization. Our approach answers how efficient self-organization for learning happens with local synaptic plasticity, provides new circuit motifs and mechanisms that could be in use in the brain, predicts computations performed in specific circuits, and provides computational interpretations of salient features of such circuits.
研究兴趣
论文共 106 篇作者统计合作学者相似作者
按年份排序按引用量排序主题筛选期刊级别筛选合作者筛选合作机构筛选
时间
引用量
主题
期刊级别
合作者
合作机构
CoRR (2024)
引用0浏览0EI引用
0
0
arxiv(2024)
引用0浏览0引用
0
0
CoRR (2024)
引用0浏览0EI引用
0
0
加载更多
作者统计
#Papers: 106
#Citation: 2045
H-Index: 23
G-Index: 43
Sociability: 5
Diversity: 2
Activity: 106
合作学者
合作机构
D-Core
- 合作者
- 学生
- 导师
数据免责声明
页面数据均来自互联网公开来源、合作出版商和通过AI技术自动分析结果,我们不对页面数据的有效性、准确性、正确性、可靠性、完整性和及时性做出任何承诺和保证。若有疑问,可以通过电子邮件方式联系我们:report@aminer.cn