Harvest Initiated Volatile Organic Compound Emissions from In-Field Tall Wheatgrass
ACS EARTH AND SPACE CHEMISTRY(2024)
Pacific Northwest Natl Lab PNNL
Abstract
While crop and grassland usage continues to increase, the full diversity of plant-specific volatile organic compounds (VOCs) emitted from these ecosystems, including their implications for atmospheric chemistry and carbon cycling, remains poorly understood. It is particularly important to investigate VOCs in the context of potential biofuels: aside from the implications of large-scale land use, harvest may shift both the flux and speciation of emitted VOCs. To this point, we evaluate the diversity of VOCs emitted both pre and postharvest from "Alkar" tall wheatgrass (Thinopyrum ponticum), a candidate biofuel that exhibits greater tolerance to frost and saline land compared to other grass varieties. Mature plants grown under field conditions (n = 6) were sampled for VOCs both pre- and postharvest (October 2022). Via hierarchical clustering of emitted VOCs from each plant, we observe distinct "volatilomes" (diversity of VOCs) specific to the pre- and postharvest conditions despite plant-to-plant variability. In total, 50 VOCs were found to be unique to the postharvest tall wheatgrass volatilome, and these unique VOCs constituted a significant portion (26%) of total postharvest signal. While green leaf volatiles (GLVs) dominate the speciation of postharvest emissions (e.g., 54% of unique postharvest VOC signal was due to 1-penten-3-ol), we demonstrate novel postharvest VOCs from tall wheatgrass that are under characterized in the context of carbon cycling and atmospheric chemistry (e.g., 3-octanone). Continuing evaluations will quantitatively investigate tall wheatgrass VOC fluxes, better informing the feasibility and environmental impact of tall wheatgrass as a biofuel.
MoreTranslated text
Key words
volatile organic compounds,tall wheatgrass,biofuel,carbon cycling,green leaf volatiles
求助PDF
上传PDF
View via Publisher
AI Read Science
AI Summary
AI Summary is the key point extracted automatically understanding the full text of the paper, including the background, methods, results, conclusions, icons and other key content, so that you can get the outline of the paper at a glance.
Example
Background
Key content
Introduction
Methods
Results
Related work
Fund
Key content
- Pretraining has recently greatly promoted the development of natural language processing (NLP)
- We show that M6 outperforms the baselines in multimodal downstream tasks, and the large M6 with 10 parameters can reach a better performance
- We propose a method called M6 that is able to process information of multiple modalities and perform both single-modal and cross-modal understanding and generation
- The model is scaled to large model with 10 billion parameters with sophisticated deployment, and the 10 -parameter M6-large is the largest pretrained model in Chinese
- Experimental results show that our proposed M6 outperforms the baseline in a number of downstream tasks concerning both single modality and multiple modalities We will continue the pretraining of extremely large models by increasing data to explore the limit of its performance
Upload PDF to Generate Summary
Must-Reading Tree
Example
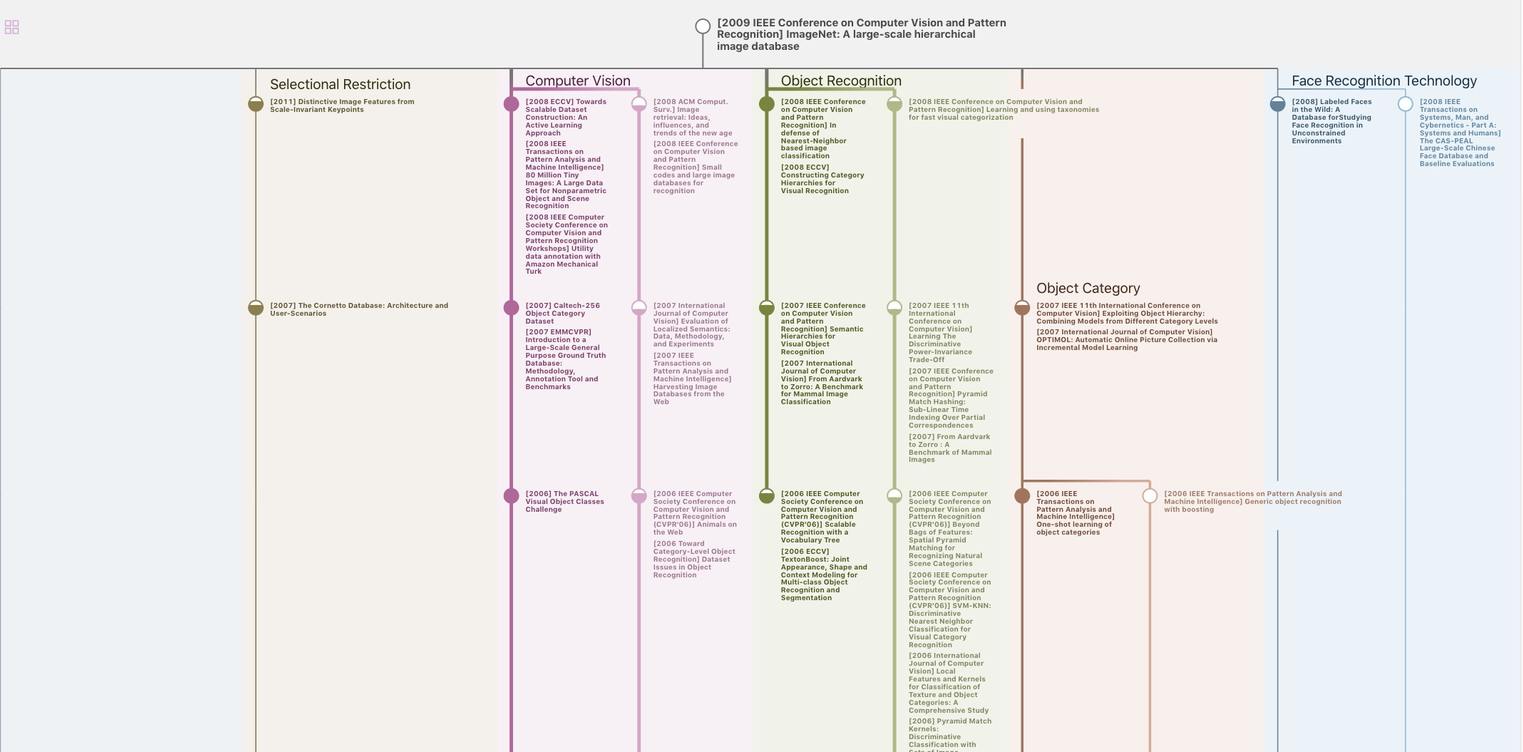
Generate MRT to find the research sequence of this paper
Related Papers
1999
被引用311 | 浏览
2009
被引用81 | 浏览
Qualitative and Quantitative Characterization of Volatile Organic Compound Emissions from Cut Grass.
2012
被引用55 | 浏览
2013
被引用25 | 浏览
2017
被引用7 | 浏览
2017
被引用43 | 浏览
2019
被引用35 | 浏览
2020
被引用17 | 浏览
2021
被引用88 | 浏览
2021
被引用13 | 浏览
2021
被引用290 | 浏览
2022
被引用57 | 浏览
2023
被引用2 | 浏览
2022
被引用5 | 浏览
Data Disclaimer
The page data are from open Internet sources, cooperative publishers and automatic analysis results through AI technology. We do not make any commitments and guarantees for the validity, accuracy, correctness, reliability, completeness and timeliness of the page data. If you have any questions, please contact us by email: report@aminer.cn
Chat Paper