Fuzzy Neighborhood-Based Manifold Learning and Feature Weight Matrix for Multilabel Feature Selection
Knowledge-Based Systems(2024)
摘要
In recent years, it has been difficult to determine neighborhood radii with almost all feature selection methodologies, and the existing multilabel feature selection approaches neglect these correlations among labels, which results in poor classification efficiency. To address these flaws, a fuzzy neighborhood-based manifold learning model and a feature weight matrix are developed to study multilabel feature selection in this article. First, the difference coefficient of the given samples and the new classification margin of each sample are defined to construct an adaptive fuzzy neighborhood radius. While considering the influences of features and labels on the similarity relationships of samples, a novel fuzzy neighborhood similarity relationship is developed by combining it with the label similarity approach that uses the Jaccard similarity coefficient. Second, the least-squares regression model is introduced to design a loss function for learning the mapping relationship between the feature and label spaces. To constrain the objective function, a regularization term based on the fuzzy neighborhood similarity relationship matrix is developed to establish a manifold learning model. To reduce the complexity of the loss function and to reduce the induced structural risk, the final objective function is constructed by incorporating two graph Laplacian matrices. Third, positive and negative confidence values are presented to incorporate the frequency parameter and to form the dependencies between labels into a new label correlation matrix. A new feature weight matrix integrated with the dependencies between labels is defined to learn the correlations between features and labels. Finally, an embedded feature selection method with an objective function is designed, where the objective function is optimized by the gradient descent scheme. Experiments conducted on 12 multilabel datasets reveal that the proposed approach yields better classification results than do other algorithms.
更多查看译文
关键词
Feature selection,Fuzzy neighborhood,Manifold learning,Objective function,Multilabel learning
AI 理解论文
溯源树
样例
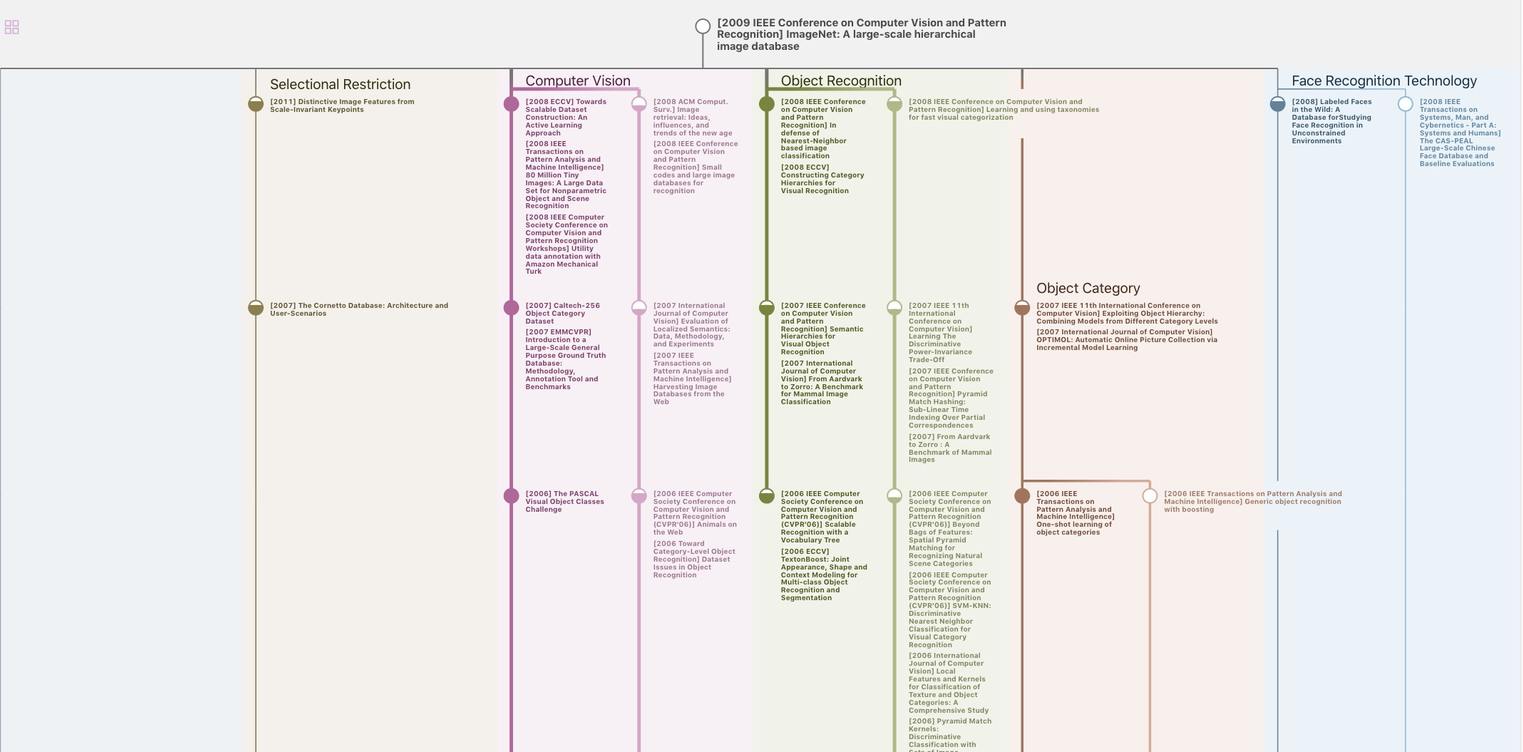
生成溯源树,研究论文发展脉络
Chat Paper
正在生成论文摘要