Limiting Over-Smoothing and Over-Squashing of Graph Message Passing by Deep Scattering Transforms
arxiv(2024)
摘要
Graph neural networks (GNNs) have become pivotal tools for processing graph-structured data, leveraging the message passing scheme as their core mechanism. However, traditional GNNs often grapple with issues such as instability, over-smoothing, and over-squashing, which can degrade performance and create a trade-off dilemma. In this paper, we introduce a discriminatively trained, multi-layer Deep Scattering Message Passing (DSMP) neural network designed to overcome these challenges. By harnessing spectral transformation, the DSMP model aggregates neighboring nodes with global information, thereby enhancing the precision and accuracy of graph signal processing. We provide theoretical proofs demonstrating the DSMP's effectiveness in mitigating these issues under specific conditions. Additionally, we support our claims with empirical evidence and thorough frequency analysis, showcasing the DSMP's superior ability to address instability, over-smoothing, and over-squashing.
更多查看译文
AI 理解论文
溯源树
样例
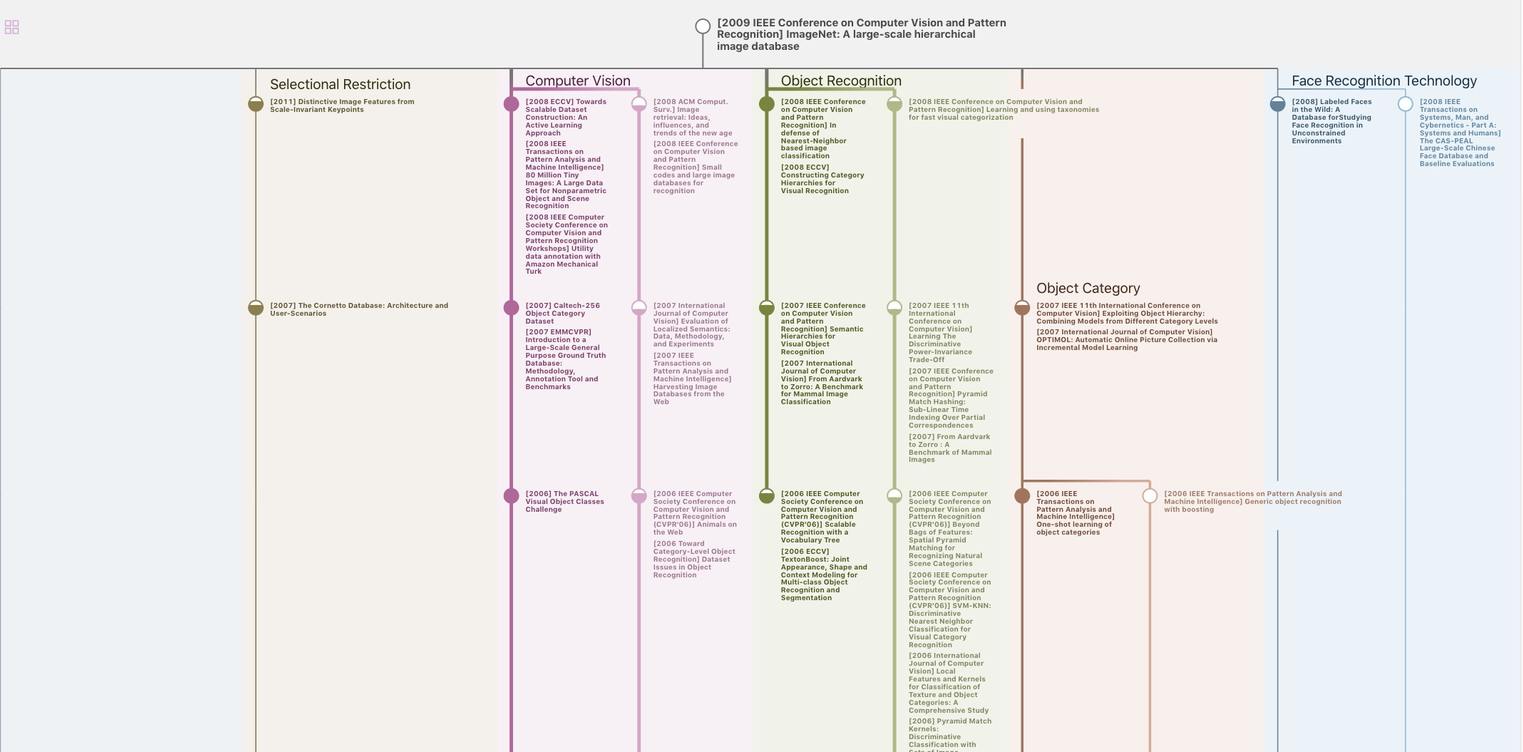
生成溯源树,研究论文发展脉络
Chat Paper
正在生成论文摘要