Distributed and Adaptive Workload Prediction for In-Network Computing
NOMS 2024-2024 IEEE Network Operations and Management Symposium(2024)
摘要
Workload prediction is the key to improving the quality of service (QoS) of various user applications running in autonomous large-scale networks. Machine learning (ML) technologies contribute to workload prediction because they provide fully automated data analytics. However, no ML algorithm can simultaneously provide perfect, timely, and generalized solutions at the same time. In this paper, we propose an adaptive optimized ML algorithm selection and installation (OMASI) method for workload prediction and predictive autoscaling to maintain the QoS of user applications. With OMASI, a central repository server collects information about the analytical objectives and optimization preferences of multiple distributively deployed ML engines and provides them with the best-suited ML algorithms for their data-analytics. We implemented and demonstrated OMASI on an experimental in-network computing testbed and evaluated the workload prediction accuracy of several available ML algorithms in terms of content downloading latency and throughput. The results show that OMASI can maintain the QoS of user applications, such as latency and throughput, by reducing the CPU usage prediction error by up to 86%.
更多查看译文
关键词
In-network Computing,In-network Function,Data Analytics,Machine Learning,ML Repository,QoS
AI 理解论文
溯源树
样例
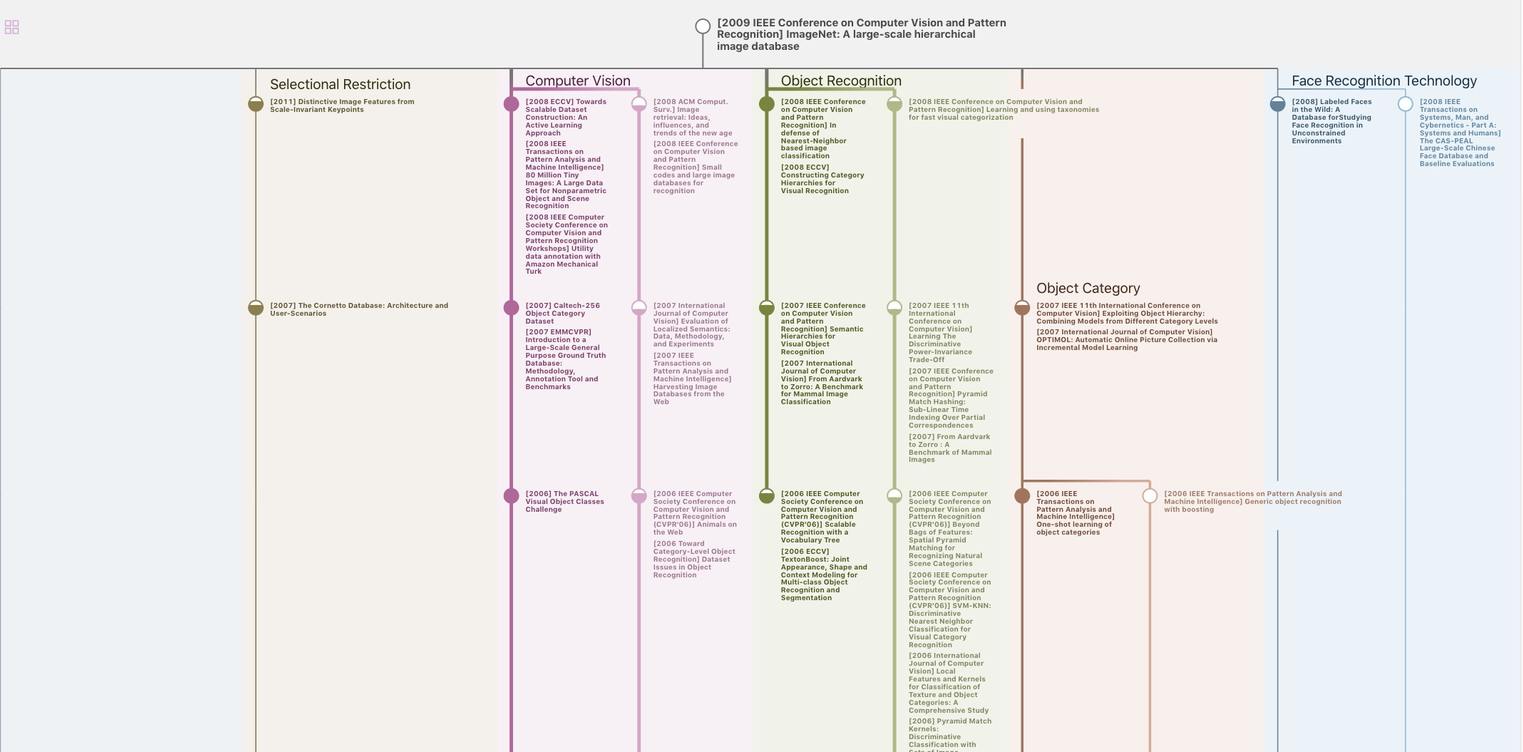
生成溯源树,研究论文发展脉络
Chat Paper
正在生成论文摘要