Predictive Assessment of the Interior Quality of Apartments Based on Multimodal Data with Variable Visual Input
SN Computer Science(2024)
摘要
Multimodal analyses in the context of automated real estate valuation (AVM) offer the possibility of enriching the models with additional information, which benefits the accuracy of the models. However, this variety of data can overwhelm common machine learning models, which generally only process certain data modalities and only a fixed data quantity. This leads to a bottleneck in information processing, as in many cases much more information is available per observation, of which only a single selection or sample is used to train the algorithm and the remaining information is disregarded. We propose a multimodal network architecture that incorporates both textual and visual inputs and fuses their information. Furthermore, we introduce a training strategy that can take advantage of a variable number of input images for each real estate object. In our experiments, we test and compare several unimodal (baseline) models with our multimodal architecture. Our approach shows several advantages in terms of model performance over unimodal approaches. The results show the best performance for the multimodal model with a variable number of visual inputs, as well as improved prediction for the underrepresented classes of indoor quality, mitigating the effects of unbalanced data. With the presented approach, which efficiently combines and merges multiple data modalities, we have shown how such a method can be easily adapted to an AVM for the extraction of supplementary information.
更多查看译文
关键词
Automated valuation models,Apartment condition,Multimodal learning,Variable CNN input
AI 理解论文
溯源树
样例
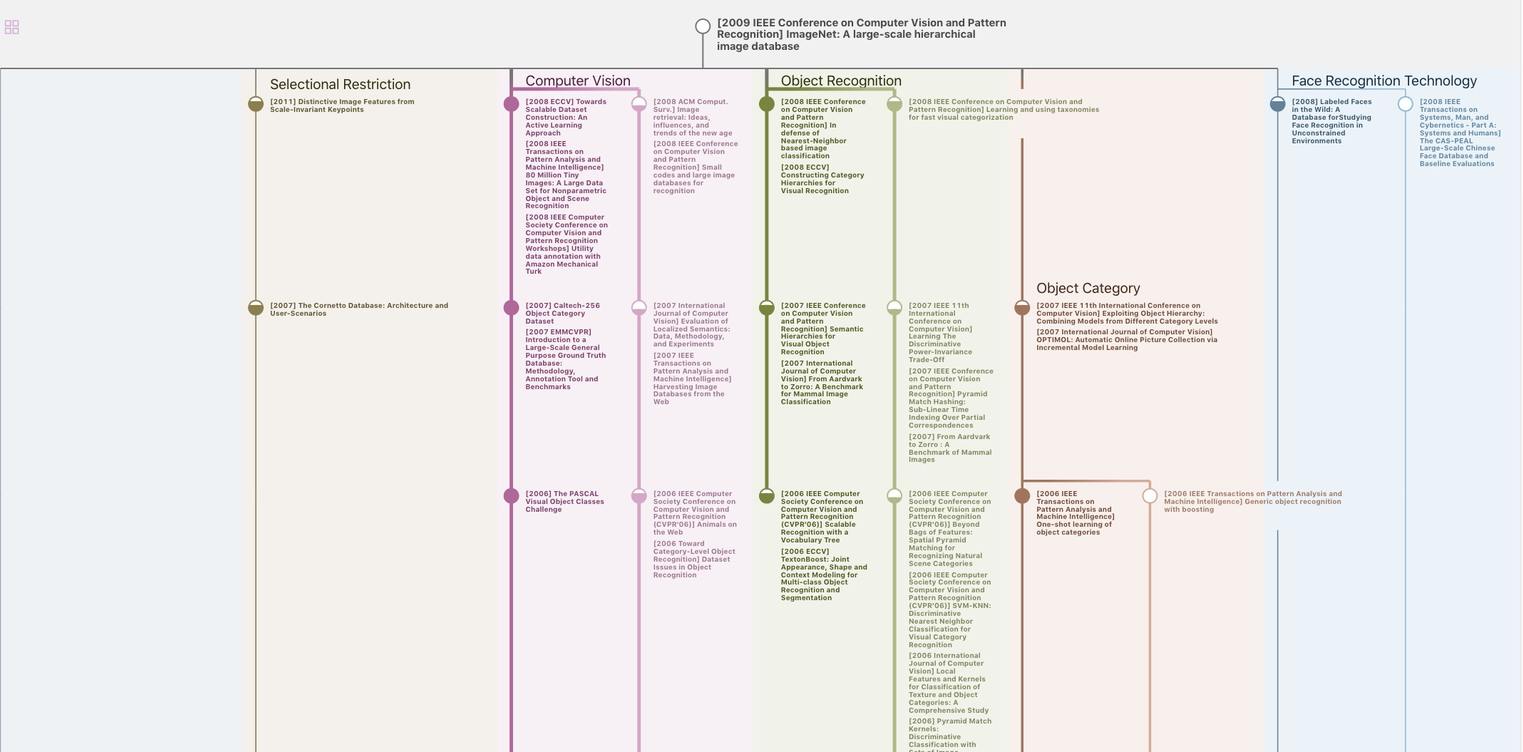
生成溯源树,研究论文发展脉络
Chat Paper
正在生成论文摘要