Dual Causes Generation Assisted Model for Multimodal Aspect-Based Sentiment Classification
IEEE transactions on neural networks and learning systems(2024)
摘要
Multimodal aspect-based sentiment classification (MABSC) aims to identify the sentiment polarity toward specific aspects in multimodal data. It has gained significant attention with the increasing use of social media platforms. Existing approaches primarily focus on analyzing the content of posts to predict sentiment. However, they often struggle with limited contextual information inherent in social media posts, hindering accurate sentiment detection. To overcome this issue, we propose a novel multimodal dual cause analysis (MDCA) method to track the underlying causes behind expressed sentiments. MDCA can provide additional reasoning cause (RC) and direct cause (DC) to explain why users express certain emotions, thus helping improve the accuracy of sentiment prediction. To develop a model with MDCA, we construct MABSC datasets with RC and DC by utilizing large language models (LLMs) and visual-language models. Subsequently, we devise a multitask learning framework that leverages the datasets with cause data to train a small generative model, which can generate RC and DC, and predict the sentiment assisted by these causes. Experimental results on MABSC benchmark datasets demonstrate that our MDCA model achieves the state-of-the-art performance, and the small fine-tuned model exhibits superior adaptability to MABSC compared to large models like ChatGPT and BLIP-2.
更多查看译文
关键词
Task analysis,Cognition,Analytical models,Sentiment analysis,Predictive models,Data models,Chatbots,Cause analysis,large language models (LLMs),multimodal aspect-based sentiment analysis,sentiment analysis
AI 理解论文
溯源树
样例
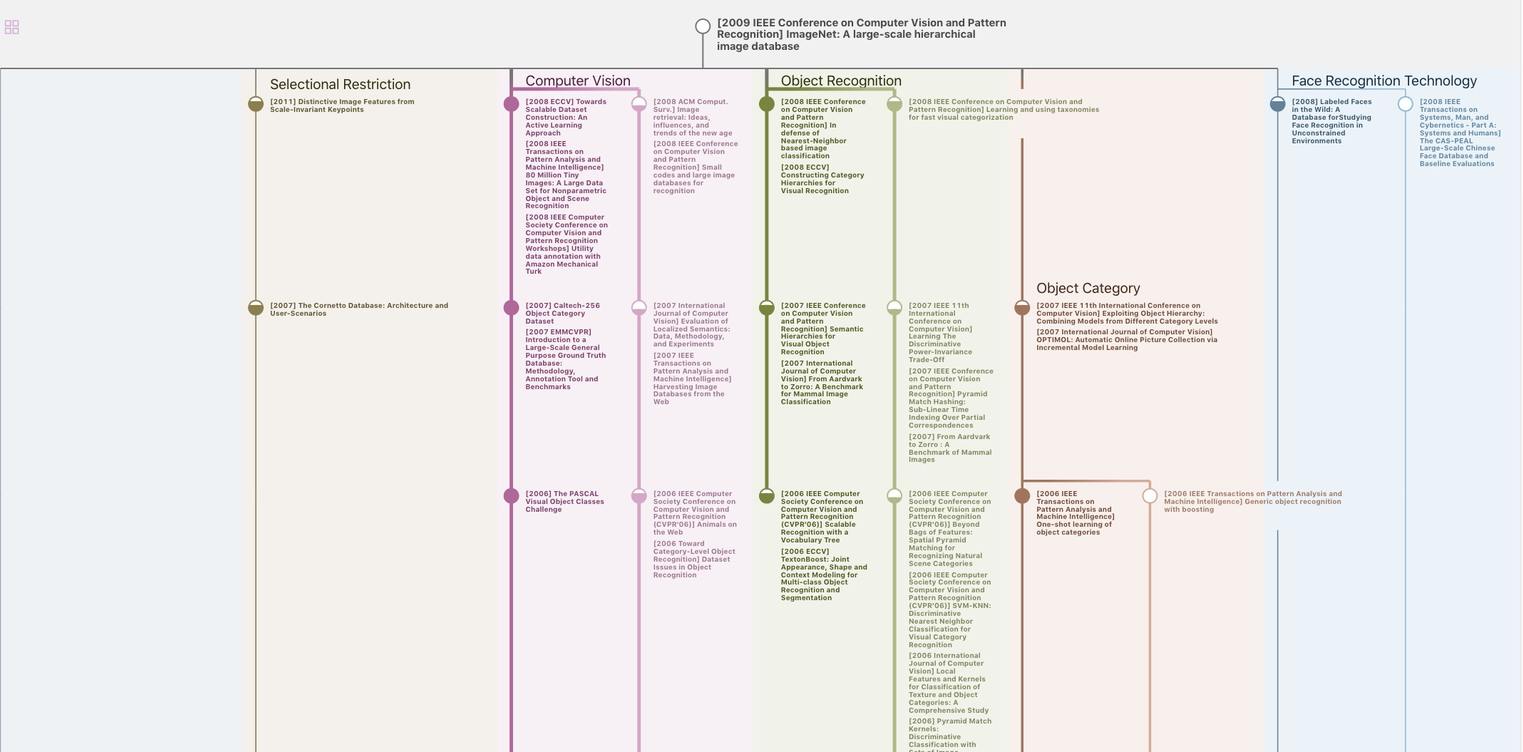
生成溯源树,研究论文发展脉络
Chat Paper
正在生成论文摘要