Efficient Online Resource Management in MEC: A Chance-Constrained Approach for Handling Uncertain Demands
IEEE Transactions on Vehicular Technology(2024)
摘要
With the proliferation of resource-hungry applications, a common paradigm to quickly respond to the everincreasing demands of tasks is mobile edge computing (MEC), which deploys resources on the premise of grasping task information (including time and quantity of task release, etc). Actually, task generation is dynamic in many practical scenarios, and it is unrealistic for MEC servers to acquire a task's demand for computing resources beforehand until task execution is completed. It is also challenging to ensure all tasks can be processed before their deadlines. In this paper, we characterize task generation as a dynamic process, implying that MEC servers only obtain the task information in the current time slot. Also, we assume the task's demand for computing resources is uncertain with only knowledge of its mean and variance. To eliminate this uncertainty, we leverage the chance-constrained program (CCP) method, which also offers a probabilistic guarantee to the task deadline. Moreover, we formulate a long-term time-averaged task processing power minimization problem, which is transformed into a series of per-slot deterministic optimization problems utilizing the Lyapunov algorithm. Given that the transformed problem is a mixed-integer non-linear programming (MINLP) problem, a joint optimization algorithm considering UE access, power control, and resource allocation (JUPR) is proposed to decouple the MINLP problem into three sub-problems. Finally, simulation results demonstrate the JUPR algorithm can consume 22% less power per task than the average resource allocation algorithm and 12% less power per task than the random resource allocation algorithm.
更多查看译文
关键词
Mobile edge computing,online resource management,chance-constrained program,probabilistic deadline guarantee,uncertain demands
AI 理解论文
溯源树
样例
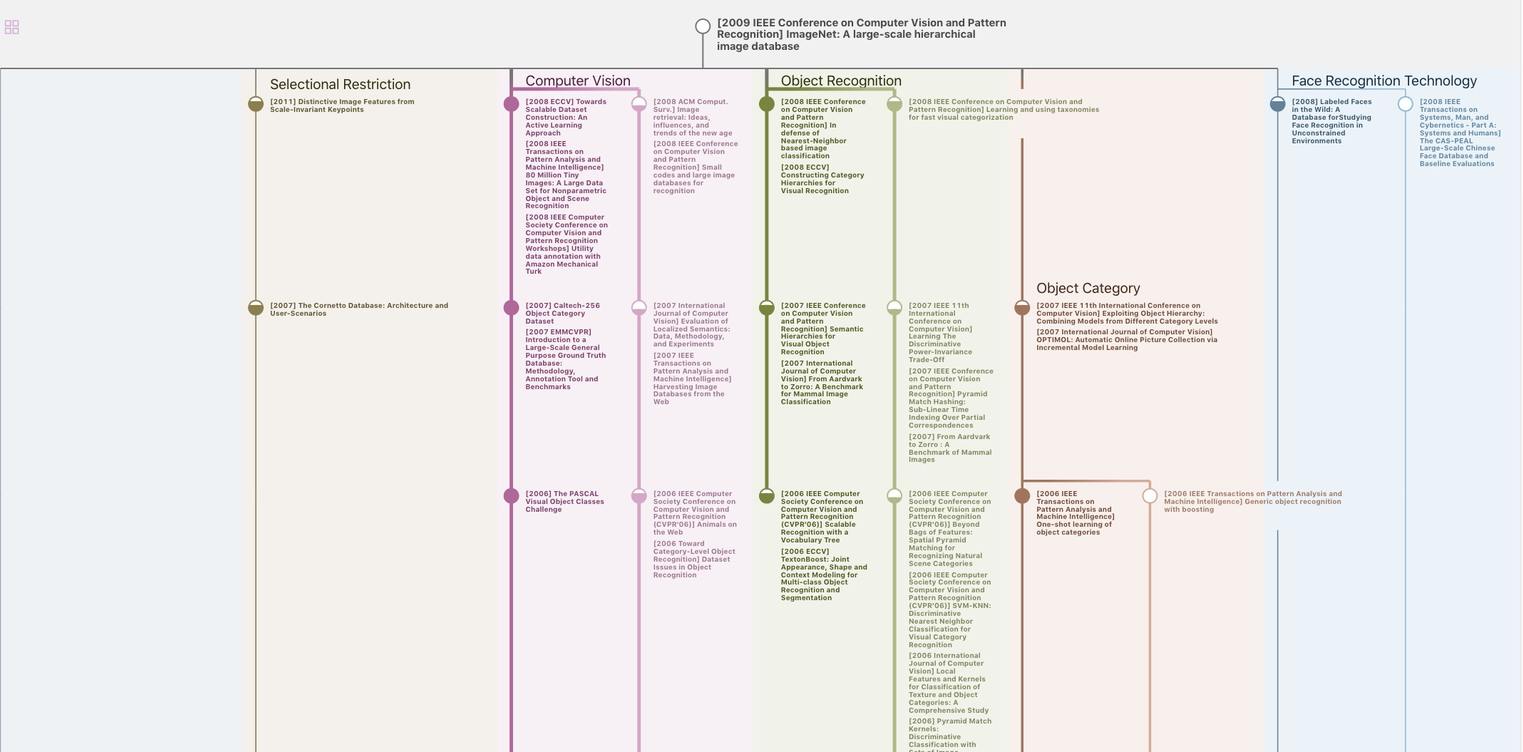
生成溯源树,研究论文发展脉络
Chat Paper
正在生成论文摘要