Enhancing Distractor Generation for Multiple-Choice Questions with Retrieval Augmented Pretraining and Knowledge Graph Integration
Annual Meeting of the Association for Computational Linguistics(2024)
摘要
In this paper, we tackle the task of distractor generation (DG) formultiple-choice questions. Our study introduces two key designs. First, wepropose retrieval augmented pretraining, which involves refining thelanguage model pretraining to align it more closely with the downstream task ofDG. Second, we explore the integration of knowledge graphs to enhance theperformance of DG. Through experiments with benchmarking datasets, we show thatour models significantly outperform the state-of-the-art results. Ourbest-performing model advances the F1@3 score from 14.80 to 16.47 in MCQdataset and from 15.92 to 16.50 in Sciq dataset.
更多查看译文
AI 理解论文
溯源树
样例
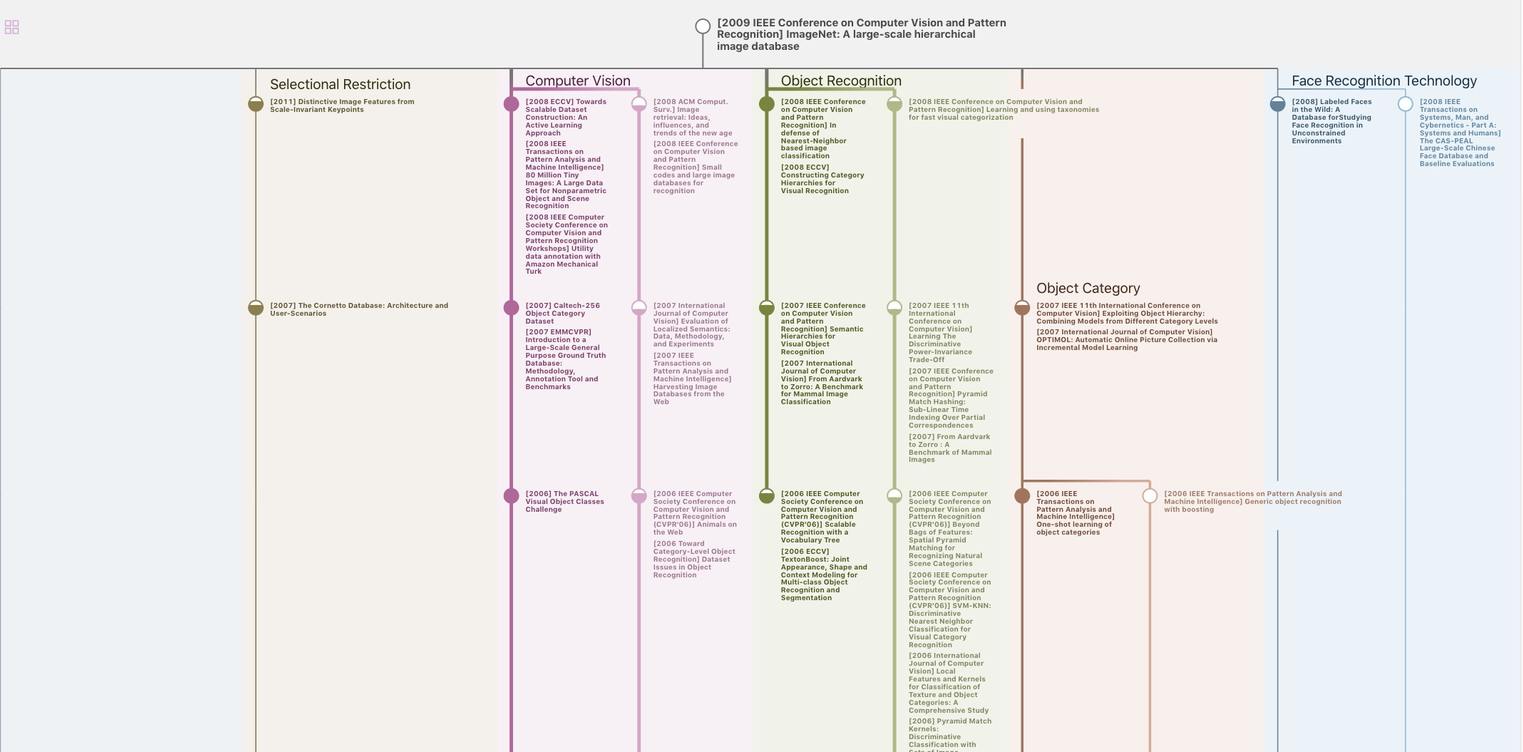
生成溯源树,研究论文发展脉络
Chat Paper
正在生成论文摘要