CLeVer: Continual Learning Visualizer for Detecting Task Transition Failure.
IEEE Pacific Visualization Symposium(2024)
摘要
We introduce CLeVer, a novel visualization system designed to analyze and enhance the performance of continual learning models by detecting task transition failures. Based on the literature review, we discovered and classified three primary causes of task transition failure in classification tasks: class/position inconsistency, biased/noisy samples, and diverse class scopes. These problems are critical in terms of model performance but were not tackled in prior research on continual learning. CLeVer is designed to address these challenges as an integrated system for model experiments and visual analysis. Firstly, users can easily configure the tasks for continual learning through a single JSON file. Then, our system automatically simulates the continual learning process in a relatively short time while generating data for visualization. Finally, the transition visualizer provides an effective visual representation of the task transition process where users can easily detect the task transition failures in continual learning. Our interview with machine learning experts and a case study with three participants demonstrate CLeVer’s utility in detecting and addressing such failures. We also discuss the system’s potential applicability and adaptability for various computer vision tasks while suggesting our future work.
更多查看译文
关键词
Human-centered computing [Visualization toolkits],Computing methodologies [Lifelong machine learning]
AI 理解论文
溯源树
样例
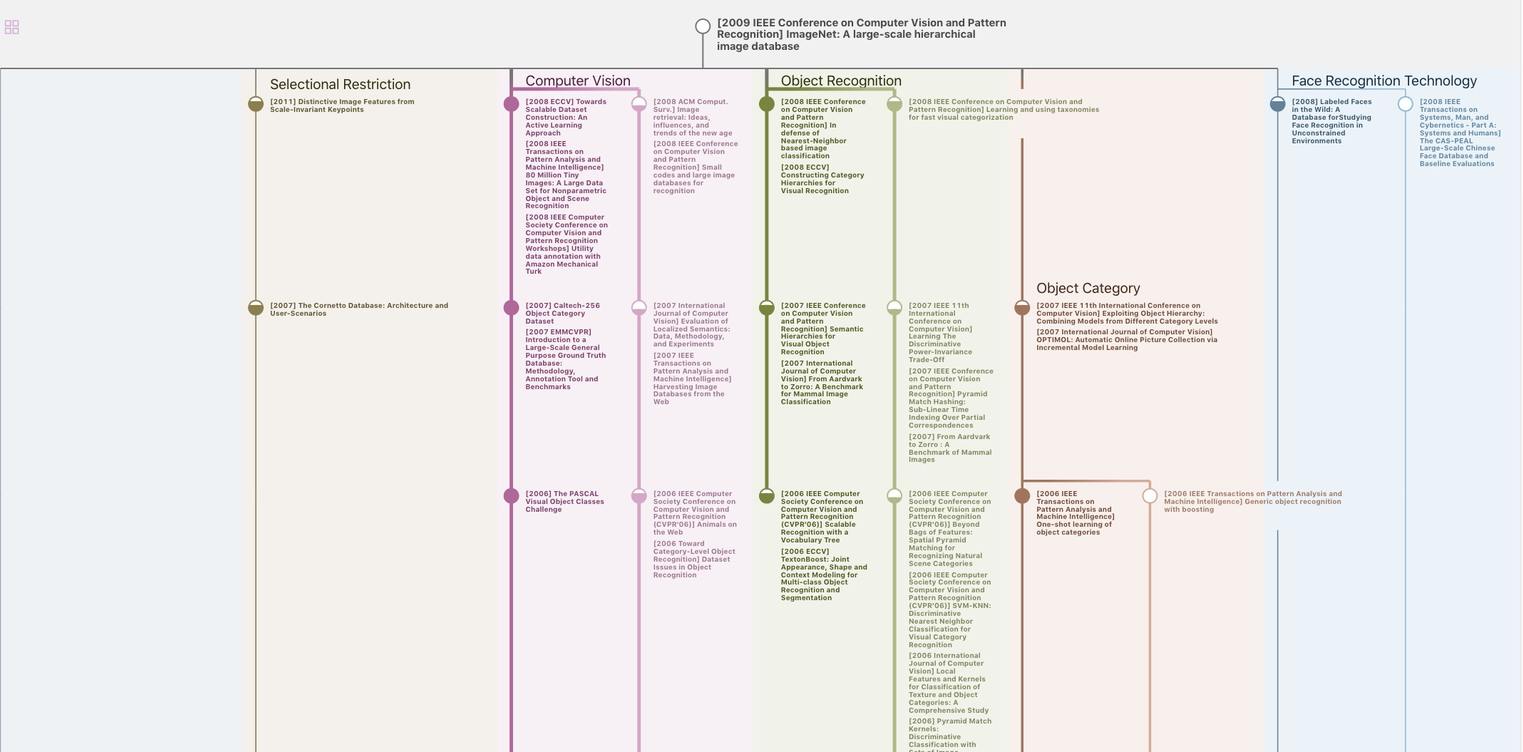
生成溯源树,研究论文发展脉络
Chat Paper
正在生成论文摘要