Mitigating annotation shift in cancer classification using single image generative models
17th International Workshop on Breast Imaging (IWBI 2024)(2024)
Abstract
Artificial Intelligence (AI) has emerged as a valuable tool for assisting
radiologists in breast cancer detection and diagnosis. However, the success of
AI applications in this domain is restricted by the quantity and quality of
available data, posing challenges due to limited and costly data annotation
procedures that often lead to annotation shifts. This study simulates, analyses
and mitigates annotation shifts in cancer classification in the breast
mammography domain. First, a high-accuracy cancer risk prediction model is
developed, which effectively distinguishes benign from malignant lesions. Next,
model performance is used to quantify the impact of annotation shift. We
uncover a substantial impact of annotation shift on multiclass classification
performance particularly for malignant lesions. We thus propose a training data
augmentation approach based on single-image generative models for the affected
class, requiring as few as four in-domain annotations to considerably mitigate
annotation shift, while also addressing dataset imbalance. Lastly, we further
increase performance by proposing and validating an ensemble architecture based
on multiple models trained under different data augmentation regimes. Our study
offers key insights into annotation shift in deep learning breast cancer
classification and explores the potential of single-image generative models to
overcome domain shift challenges.
MoreTranslated text
AI Read Science
Must-Reading Tree
Example
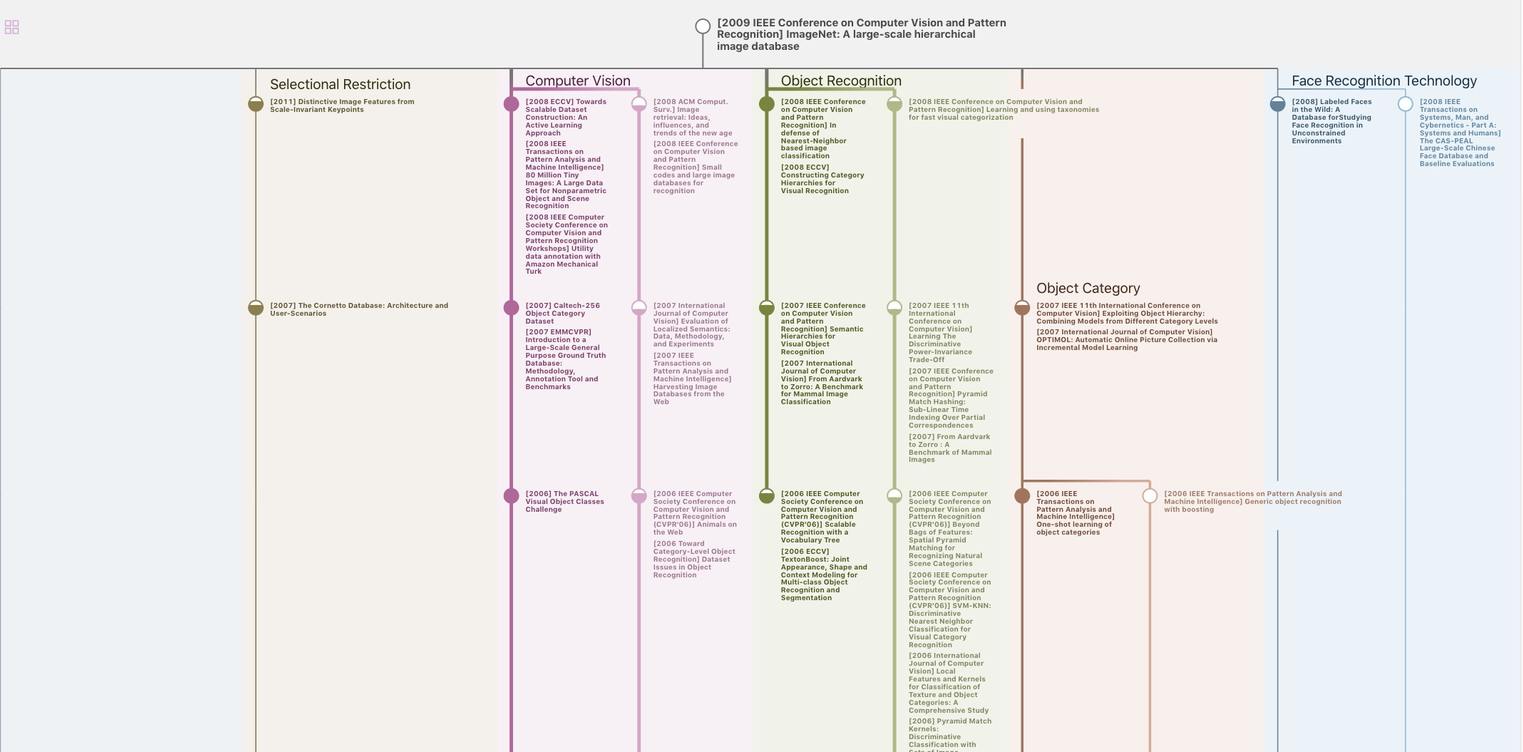
Generate MRT to find the research sequence of this paper
Chat Paper
Summary is being generated by the instructions you defined