Data-driven prediction of inter-layer process condition variations in laser powder bed fusion
Additive Manufacturing(2024)
摘要
The pressing problem of laser powder bed fusion of metals (PBF-LB/M) is the inability to predict and mitigate undesired process conditions in a build. This study aims to develop an efficient and geometric-agnostic prediction tool for the variations of the average meltpool temperature of each layer given a laser scanning path. The approach is a data-driven model based on defined physics-based features and measurement data from a two-color coaxial photodiode system. Although the coaxial photodiode system cannot measure absolute meltpool temperature, it can measure relative changes in the meltpool temperature if the process is performed in the shallow or no-keyhole regimes, the focus of this study. Once trained, the model can predict meltpool temperature variations before the start of the build process for a part geometry which had not yet been printed. The results show that variations in the layer average meltpool temperature can be predicted with the average coefficient of determination (r2) score of 0.65. Furthermore, the method shows the significance of considering the effect of surrounding parts on the average meltpool temperature profile of an observed part. For part geometries investigated in this study, the model shows the information from at least 40 previous layers is needed to enable sufficient and stabilized prediction performance.
更多查看译文
关键词
Additive manufacturing,Laser powder bed fusion,Meltpool temperature variations,Coaxial measurements,Machine learning
AI 理解论文
溯源树
样例
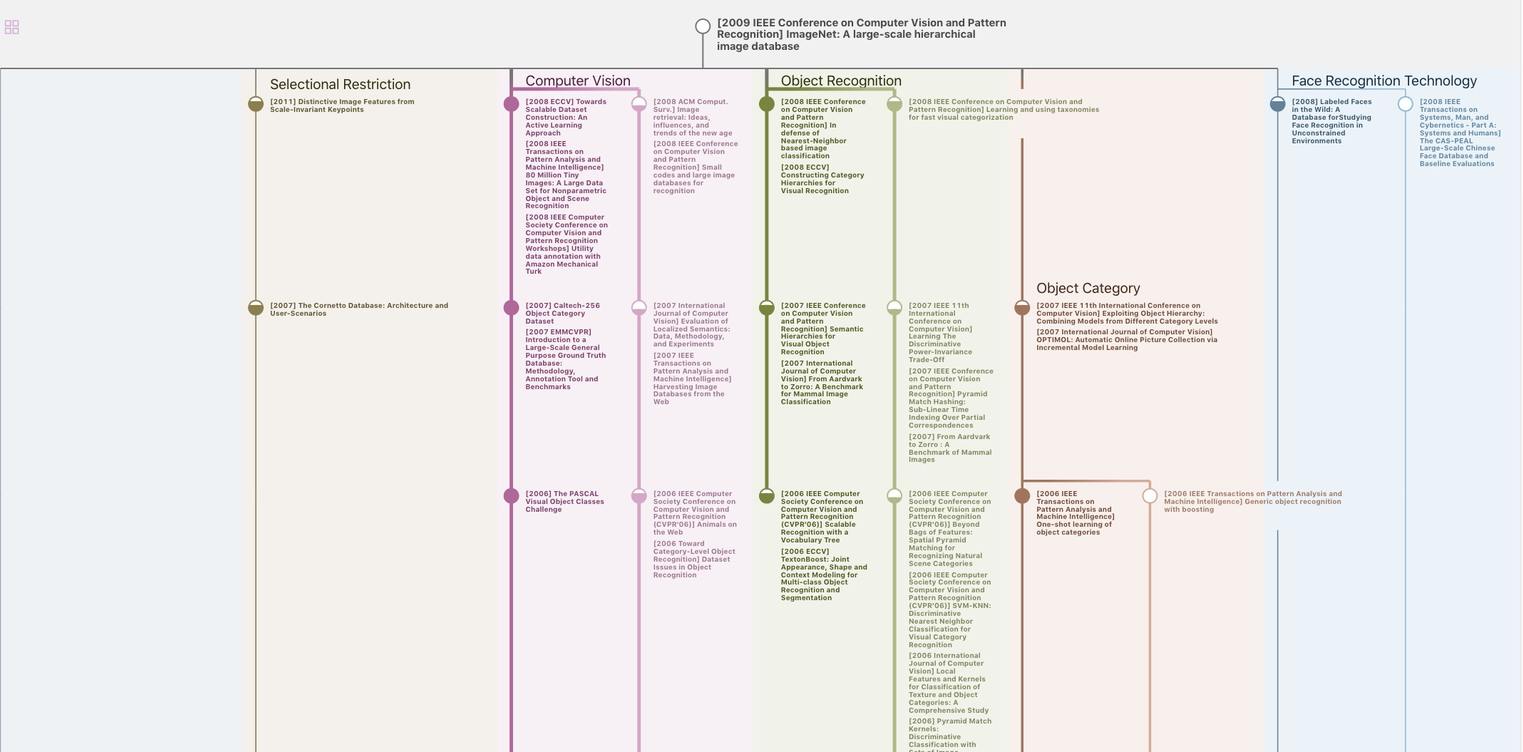
生成溯源树,研究论文发展脉络
Chat Paper
正在生成论文摘要