Machine Learning Models for Assessing Risk Factors Affecting Health Care Costs: 12-Month Exercise-Based Cardiac Rehabilitation
Frontiers in Public Health(2024)
摘要
Introduction: Exercise-based cardiac rehabilitation (ECR) has proven to be effective and cost-effective dominant treatment option in health care. However, the contribution of well-known risk factors for prognosis of coronary artery disease (CAD) to predict health care costs is not well recognized. Since machine learning (ML) applications are rapidly giving new opportunities to assist health care professionals' work, we used selected ML tools to assess the predictive value of defined risk factors for health care costs during 12-month ECR in patients with CAD. Methods: The data for analysis was available from a total of 71 patients referred to Oulu University Hospital, Finland, due to an acute coronary syndrome (ACS) event (75% men, age 61 +/- 12 years, BMI 27 +/- 4 kg/m2, ejection fraction 62 +/- 8, 89% have beta-blocker medication). Risk factors were assessed at the hospital immediately after the cardiac event, and health care costs for all reasons were collected from patient registers over a year. ECR was programmed in accordance with international guidelines. Risk analysis algorithms (cross-decomposition algorithms) were employed to rank risk factors based on variances in their effects. Regression analysis was used to determine the accounting value of risk factors by entering first the risk factor with the highest degree of explanation into the model. After that, the next most potent risk factor explaining costs was added to the model one by one (13 forecast models in total). Results: The ECR group used health care services during the year at an average of 1,624 +/- 2,139 per patient. Diabetes exhibited the strongest correlation with health care expenses (r = 0.406), accounting for 16% of the total costs (p < 0.001). When the next two ranked markers (body mass index; r = 0.171 and systolic blood pressure; r = - 0.162, respectively) were added to the model, the predictive value was 18% for the costs (p = 0.004). The depression scale had the weakest independent explanation rate of all 13 risk factors (explanation value 0.1%, r = 0.029, p = 0.811). Discussion: Presence of diabetes is the primary reason forecasting health care costs in 12-month ECR intervention among ACS patients. The ML tools may help decision-making when planning the optimal allocation of health care resources.
更多查看译文
关键词
exercise,cardiac rehabilitation,coronary artery disease,coronary heart disease,artificial intelligence,health care costs,economic evaluation
AI 理解论文
溯源树
样例
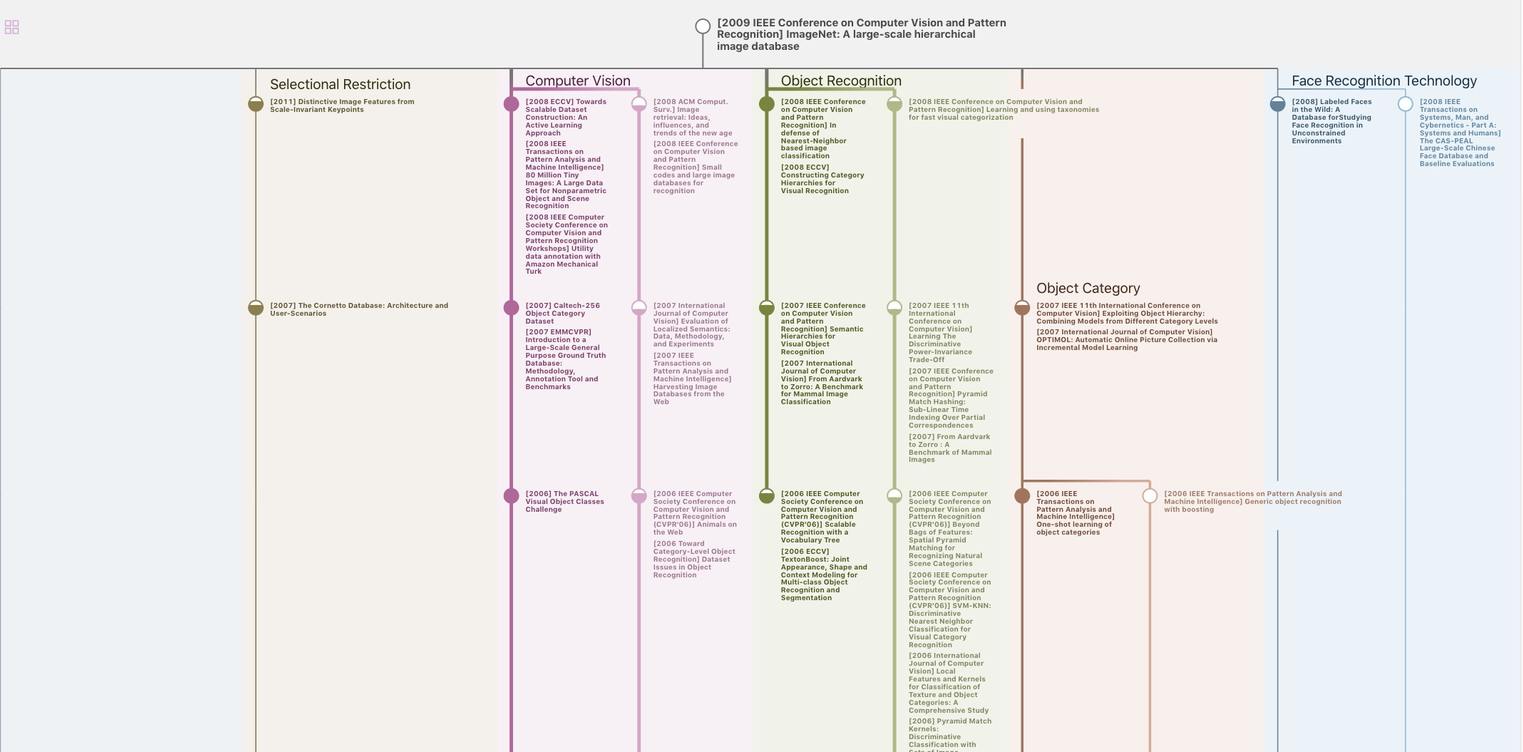
生成溯源树,研究论文发展脉络
Chat Paper
正在生成论文摘要